Multimodal MRI segmentation of key structures for microvascular decompression via knowledge-driven mutual distillation and topological constraints
International Journal of Computer Assisted Radiology and Surgery(2024)
摘要
Microvascular decompression (MVD) is a widely used neurosurgical intervention for the treatment of cranial nerves compression. Segmentation of MVD-related structures, including the brainstem, nerves, arteries, and veins, is critical for preoperative planning and intraoperative decision-making. Automatically segmenting structures related to MVD is still challenging for current methods due to the limited information from a single modality and the complex topology of vessels and nerves. Considering that it is hard to distinguish MVD-related structures, especially for nerve and vessels with similar topology, we design a multimodal segmentation network with a shared encoder–dual decoder structure and propose a clinical knowledge-driven distillation scheme, allowing reliable knowledge transferred from each decoder to the other. Besides, we introduce a class-wise contrastive module to learn the discriminative representations by maximizing the distance among classes across modalities. Then, a projected topological loss based on persistent homology is proposed to constrain topological continuity. We evaluate the performance of our method on in-house dataset consisting of 100 paired HR-T2WI and 3D TOF-MRA volumes. Experiments indicate that our model outperforms the SOTA in DSC by 1.9 https://github.com/JaronTu/Multimodal_MVD_Seg .
更多查看译文
关键词
Multimodal MRI segmentation,MVD,Topological constraints,Contrastive learning,Mutual distillation
AI 理解论文
溯源树
样例
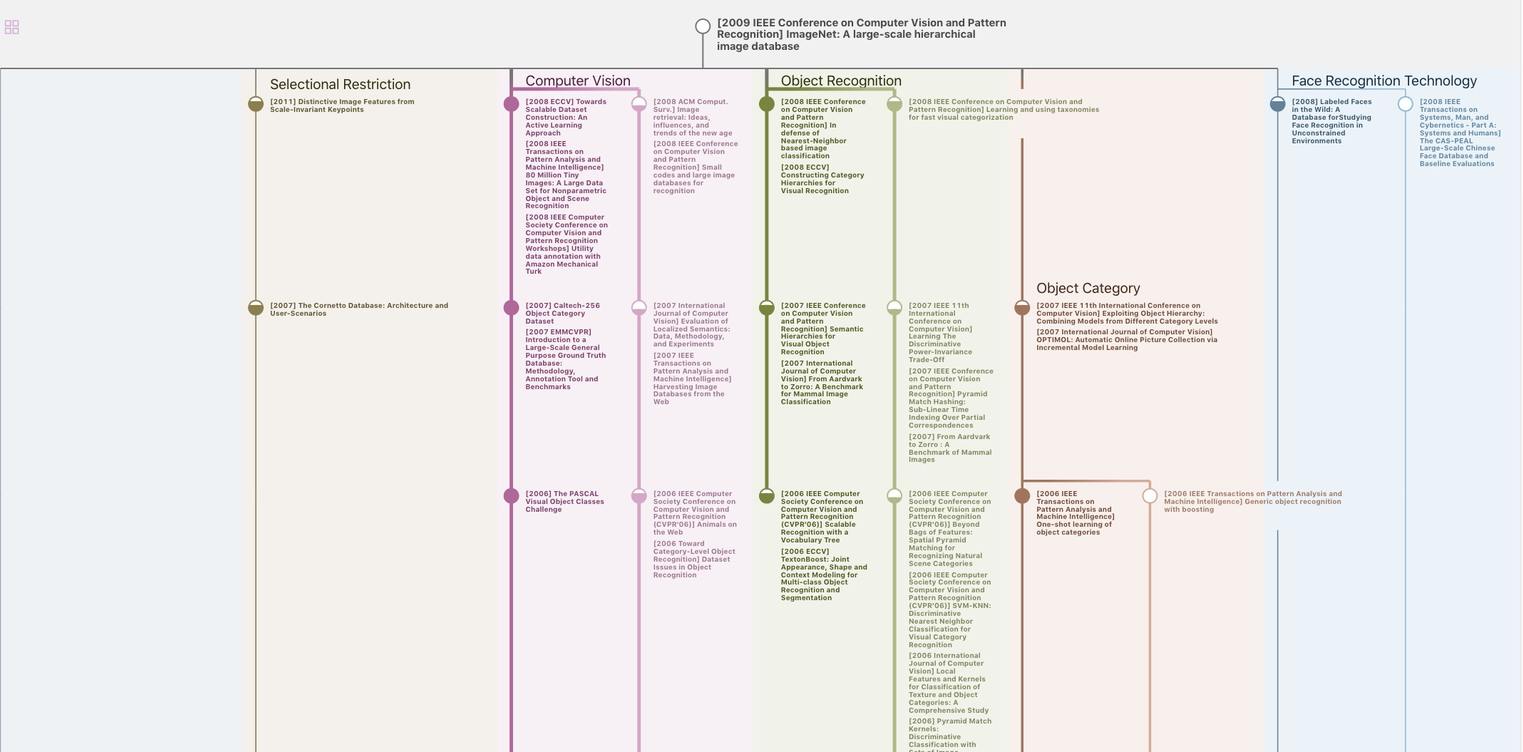
生成溯源树,研究论文发展脉络
Chat Paper
正在生成论文摘要