Efficient End-to-End Detection of 6-DoF Grasps for Robotic Bin Picking
arxiv(2024)
摘要
Bin picking is an important building block for many robotic systems, in
logistics, production or in household use-cases. In recent years, machine
learning methods for the prediction of 6-DoF grasps on diverse and unknown
objects have shown promising progress. However, existing approaches only
consider a single ground truth grasp orientation at a grasp location during
training and therefore can only predict limited grasp orientations which leads
to a reduced number of feasible grasps in bin picking with restricted
reachability. In this paper, we propose a novel approach for learning dense and
diverse 6-DoF grasps for parallel-jaw grippers in robotic bin picking. We
introduce a parameterized grasp distribution model based on Power-Spherical
distributions that enables a training based on all possible ground truth
samples. Thereby, we also consider the grasp uncertainty enhancing the model's
robustness to noisy inputs. As a result, given a single top-down view depth
image, our model can generate diverse grasps with multiple collision-free grasp
orientations. Experimental evaluations in simulation and on a real robotic bin
picking setup demonstrate the model's ability to generalize across various
object categories achieving an object clearing rate of around 90 % in
simulation and real-world experiments. We also outperform state of the art
approaches. Moreover, the proposed approach exhibits its usability in real
robot experiments without any refinement steps, even when only trained on a
synthetic dataset, due to the probabilistic grasp distribution modeling.
更多查看译文
AI 理解论文
溯源树
样例
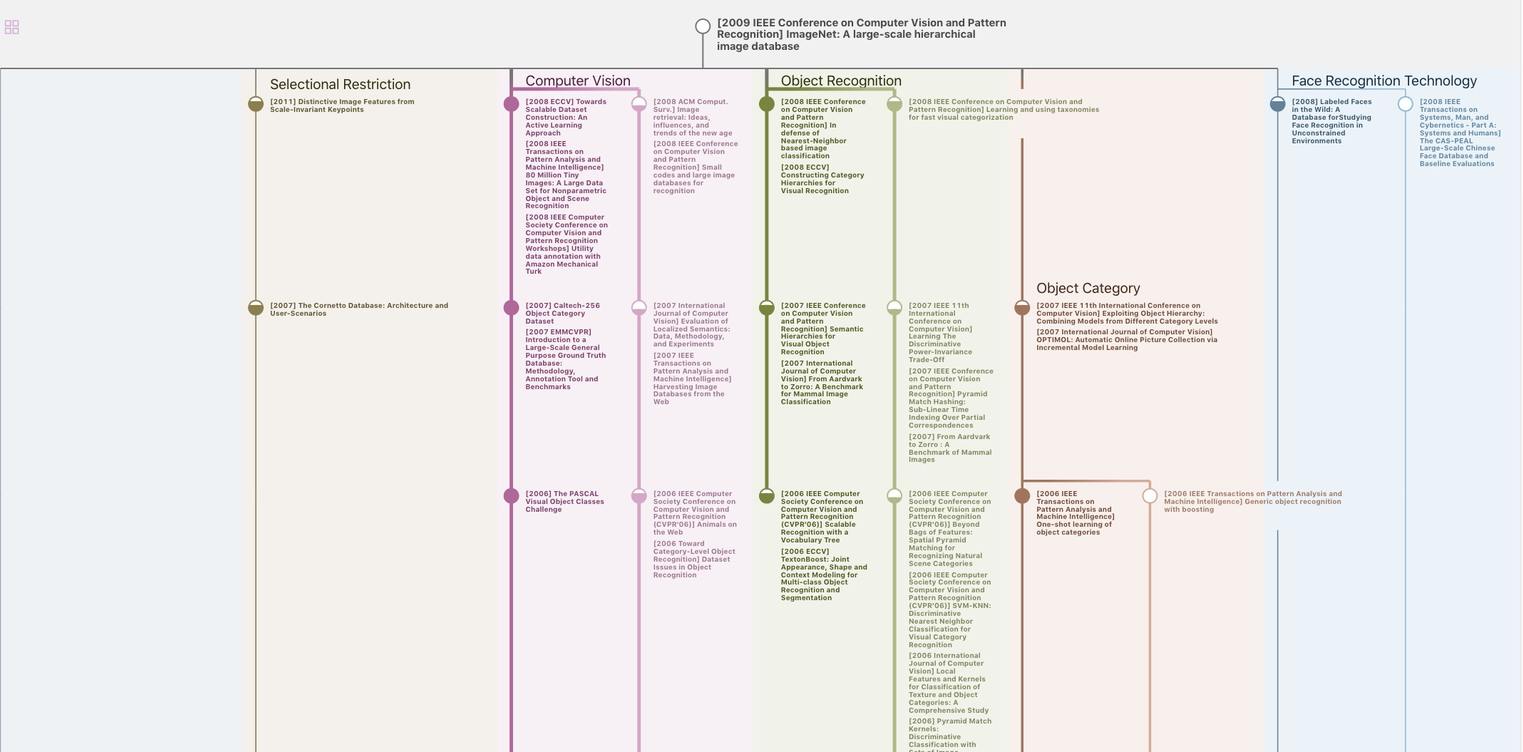
生成溯源树,研究论文发展脉络
Chat Paper
正在生成论文摘要