Improving Targeted Mass Spectrometry Data Analysis with Nested Active Machine Learning
ADVANCED INTELLIGENT SYSTEMS(2024)
摘要
Targeted mass spectrometry (MS) holds promise for precise protein and protein-representative peptide identification and quantification, enhancing disease diagnosis. However, its clinical application is hindered by complex data analysis and expert review requirements. It is hypothesized that machine learning (ML) models can automate data analysis to accelerate the clinical application of MS. The approach involves an ML-driven pipeline that extracts statistical and morphological features from an MS target region and feeds these features into ML algorithms to generate and assess predictive models. The findings demonstrate ML prediction models exhibit superior performance when trained on extracted features versus raw spectra intensity data and that random forest models exhibit robust classification performance in both internal and external validation datasets. These models remain effective across varying training dataset sizes and positive sample rates and are enhanced by a nested active learning approach. This approach can thus revolutionize clinical MS applications. This study develops a machine learning pipeline for targeted mass spectrometry (MS) data, enhancing data analysis automation. It integrates statistical and morphological feature extraction from MS signals, uses a nested active learning algorithm to enhance the training set, and achieves high predictive accuracy with smaller datasets.image (c) 2024 WILEY-VCH GmbH
更多查看译文
关键词
active learning,feature extraction,importance analysis,liquid chromatography tandem mass spectrometry,small training datasets
AI 理解论文
溯源树
样例
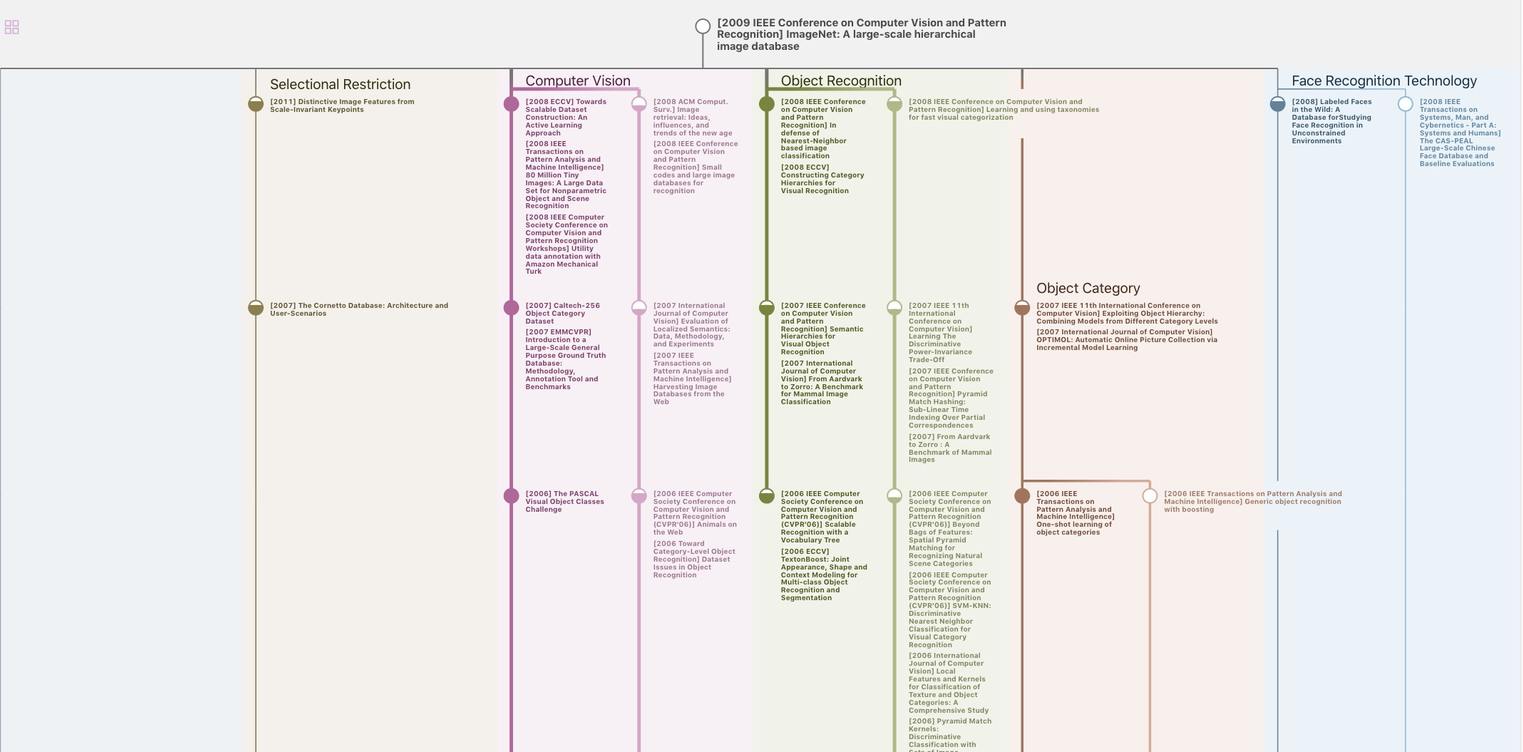
生成溯源树,研究论文发展脉络
Chat Paper
正在生成论文摘要