Believing Anthropomorphism: Examining the Role of Anthropomorphic Cues on Trust in Large Language Models
Extended Abstracts of the CHI Conference on Human Factors in Computing Systems(2024)
摘要
People now regularly interface with Large Language Models (LLMs) via speech
and text (e.g., Bard) interfaces. However, little is known about the
relationship between how users anthropomorphize an LLM system (i.e., ascribe
human-like characteristics to a system) and how they trust the information the
system provides. Participants (n=2,165; ranging in age from 18-90 from the
United States) completed an online experiment, where they interacted with a
pseudo-LLM that varied in modality (text only, speech + text) and grammatical
person ("I" vs. "the system") in its responses. Results showed that the "speech
+ text" condition led to higher anthropomorphism of the system overall, as well
as higher ratings of accuracy of the information the system provides.
Additionally, the first-person pronoun ("I") led to higher information accuracy
and reduced risk ratings, but only in one context. We discuss these findings
for their implications for the design of responsible, human-generative AI
experiences.
更多查看译文
AI 理解论文
溯源树
样例
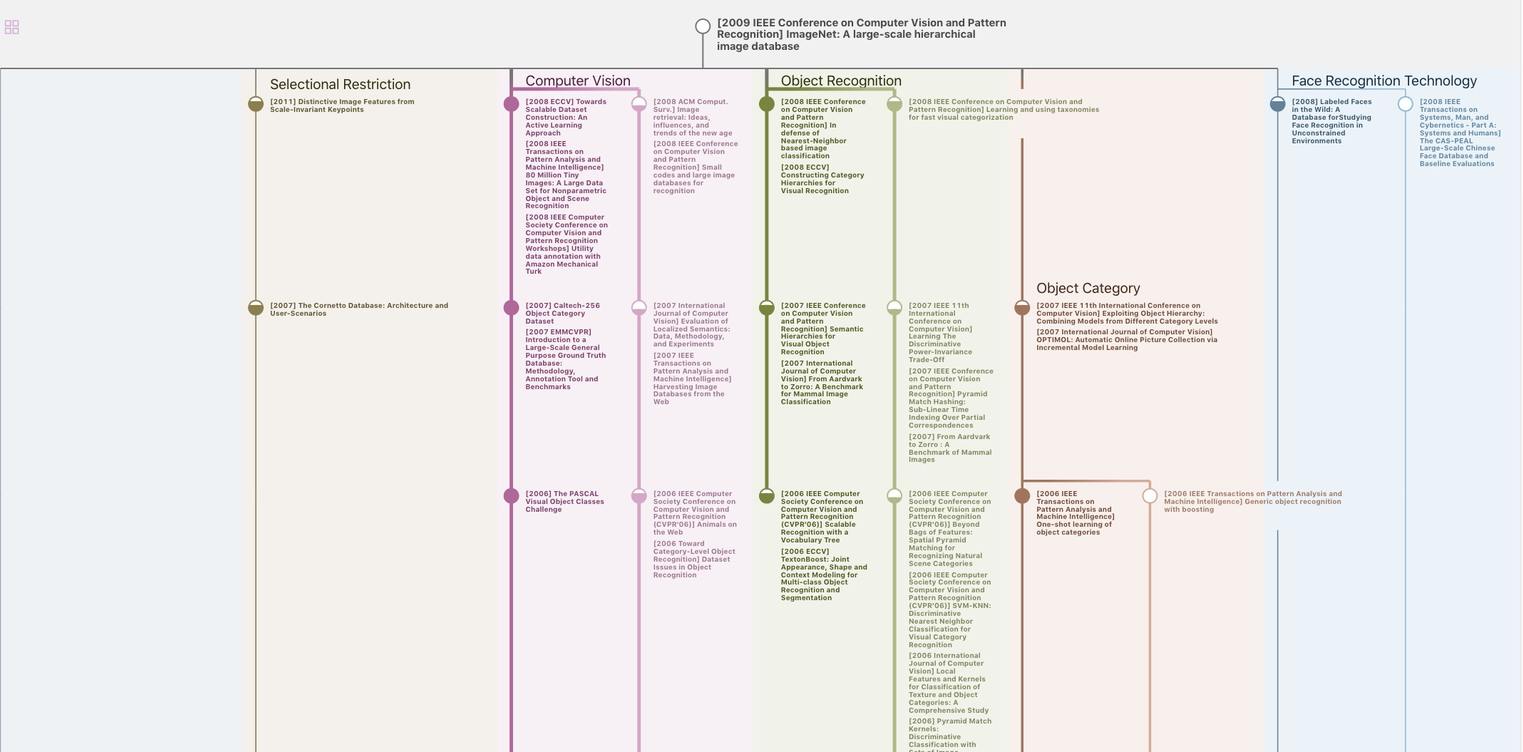
生成溯源树,研究论文发展脉络
Chat Paper
正在生成论文摘要