Deep Learning-Based Dispersion Spectrum Inversion for Surface Wave Exploration.
IEEE Trans. Geosci. Remote. Sens.(2024)
摘要
The conventional surface wave inversion methodology relying upon dispersion curve analyses is frequently confounded by the phenomenon known as "mode kissing" as well as the absorptive properties of seismic waves traversing near-surface geologic strata. We propose a deep learning approach to overcome challenges in surface wave inversion due to complex near-surface geology. A convolutional neural network regression model is developed to directly map surface wave dispersion spectra to shear wave velocity profiles. The network takes entire spectrograms as input, avoiding issues from manual picking of individual dispersion curves. This circumvents problems of mode misidentification and crossover that hamper traditional inversion in layered media. Furthermore, we incorporate the quality factor Q into calculating the theoretical frequency dispersion spectrum to account for the absorptive influence of geologic formations. Through studying theoretical spectra, we found that when the Q factor is less than 10, the absorption of the formation will have a greater impact on the dispersion imaging. Compared to existing workflows requiring manual parameter tuning and computationally expensive inversion calculations, the trained network directly infers velocities without user intervention or iterative optimization. The end-to-end relation learned from synthetic training data enables rapid interpretation of field records complicated by low-velocity zones, high-velocity inclusions, which inhibit conventional inversion algorithms.
更多查看译文
关键词
Rayleigh wave,surface wave,deep learning,the kernel of Green’s function (KGF)
AI 理解论文
溯源树
样例
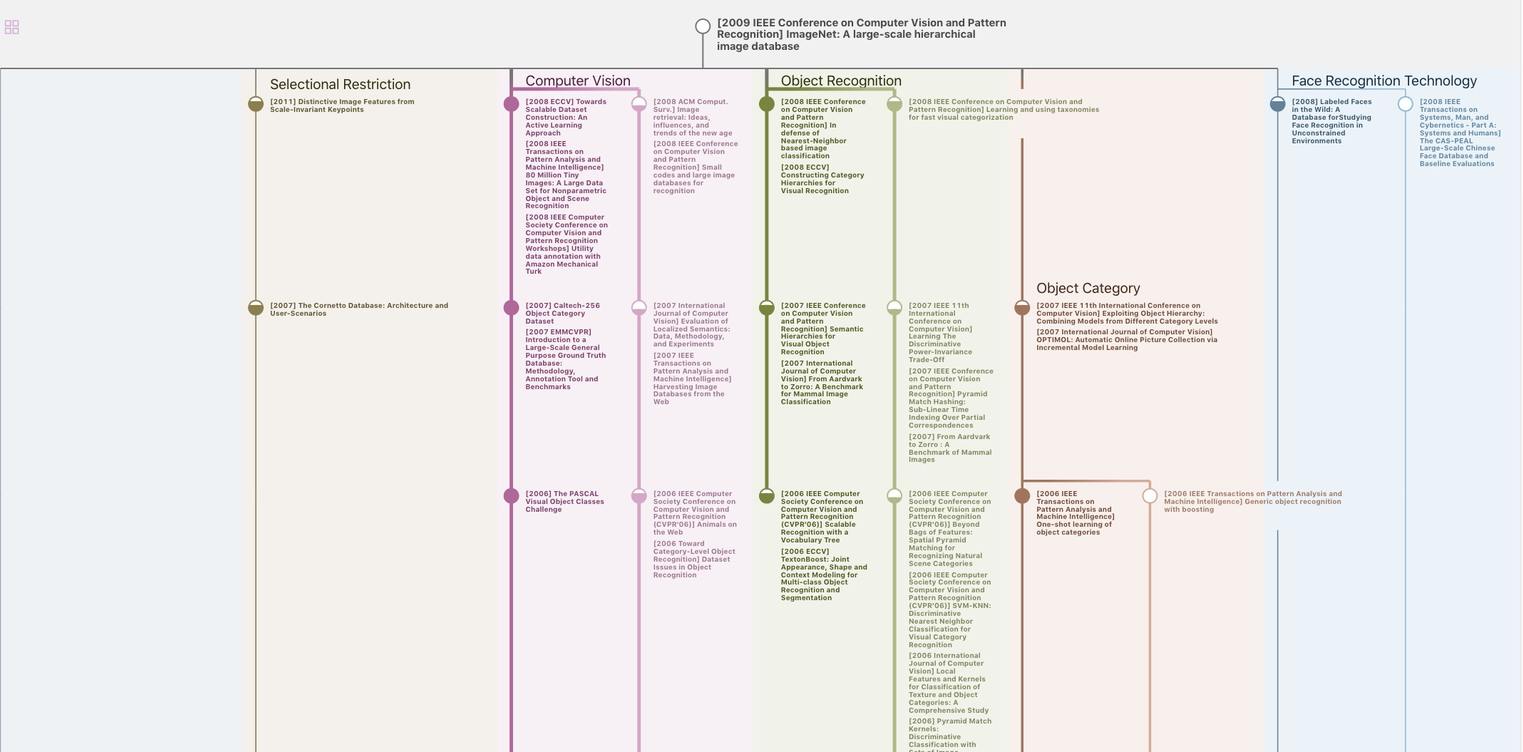
生成溯源树,研究论文发展脉络
Chat Paper
正在生成论文摘要