Deep-learning-based prediction framework for protein-peptide interactions with structure generation pipeline
Cell Reports Physical Science(2024)
摘要
Peptides play a crucial role in regulating various cellular processes through protein-peptide interactions (PpIs), offering promising avenues for therapeutic development. However, the reliable prediction of PpIs faces challenges in generating high-quality protein-peptide structures for large-scale modeling. Despite recent advancements in deep learning (DL) techniques, many predicted structures lack the necessary accuracy for efficient peptide screening. To address this, we propose a template-based PpI structure modeling pipeline, optimizing the accuracy-speed trade-off. In addition, we present a DL-based PpI prediction framework, called the Interaction Transformer Net (ITN), to detect PpIs at the residue level. The ITN model trained on the structures generated by our pipeline achieves state-of-the-art (SOTA) predictions on peptide-SH3 systems (average AUC = 0.88) and is comparable with the SOTA sequence-based prediction methods for peptide-MHC I (average AUC = 0.92), implying that ITN is a powerful tool for PpI prediction and may help advance vaccine design and peptide drug development.
更多查看译文
关键词
protein-peptide interactions,protein-peptide complex structure generation,template-based modeling,deep learning
AI 理解论文
溯源树
样例
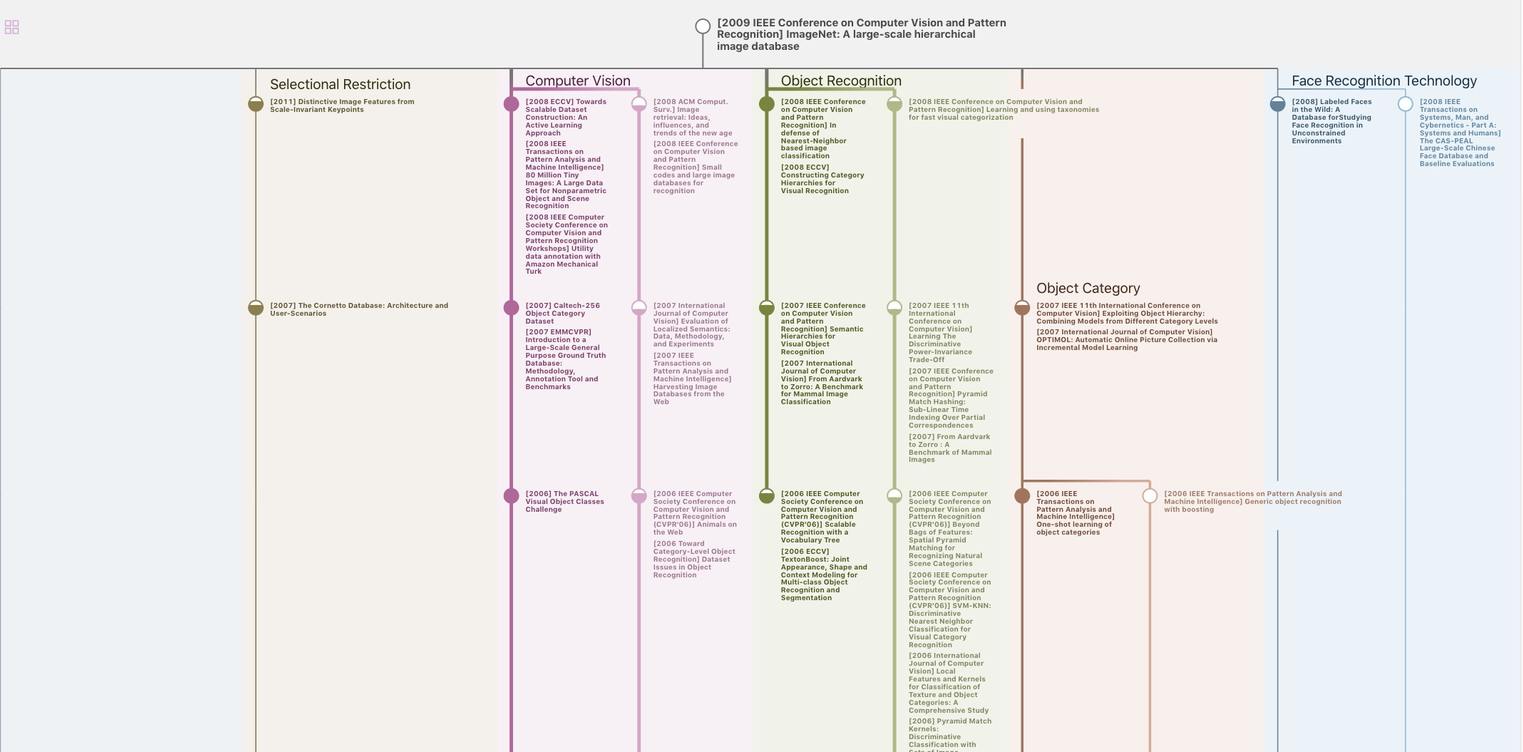
生成溯源树,研究论文发展脉络
Chat Paper
正在生成论文摘要