Parallel Cross Strip Attention Network for Single Image Dehazing
CoRR(2024)
摘要
The objective of single image dehazing is to restore hazy images and produce
clear, high-quality visuals. Traditional convolutional models struggle with
long-range dependencies due to their limited receptive field size. While
Transformers excel at capturing such dependencies, their quadratic
computational complexity in relation to feature map resolution makes them less
suitable for pixel-to-pixel dense prediction tasks. Moreover, fixed kernels or
tokens in most models do not adapt well to varying blur sizes, resulting in
suboptimal dehazing performance. In this study, we introduce a novel dehazing
network based on Parallel Stripe Cross Attention (PCSA) with a multi-scale
strategy. PCSA efficiently integrates long-range dependencies by simultaneously
capturing horizontal and vertical relationships, allowing each pixel to capture
contextual cues from an expanded spatial domain. To handle different sizes and
shapes of blurs flexibly, We employs a channel-wise design with varying
convolutional kernel sizes and strip lengths in each PCSA to capture context
information at different scales.Additionally, we incorporate a softmax-based
adaptive weighting mechanism within PCSA to prioritize and leverage more
critical features.
更多查看译文
AI 理解论文
溯源树
样例
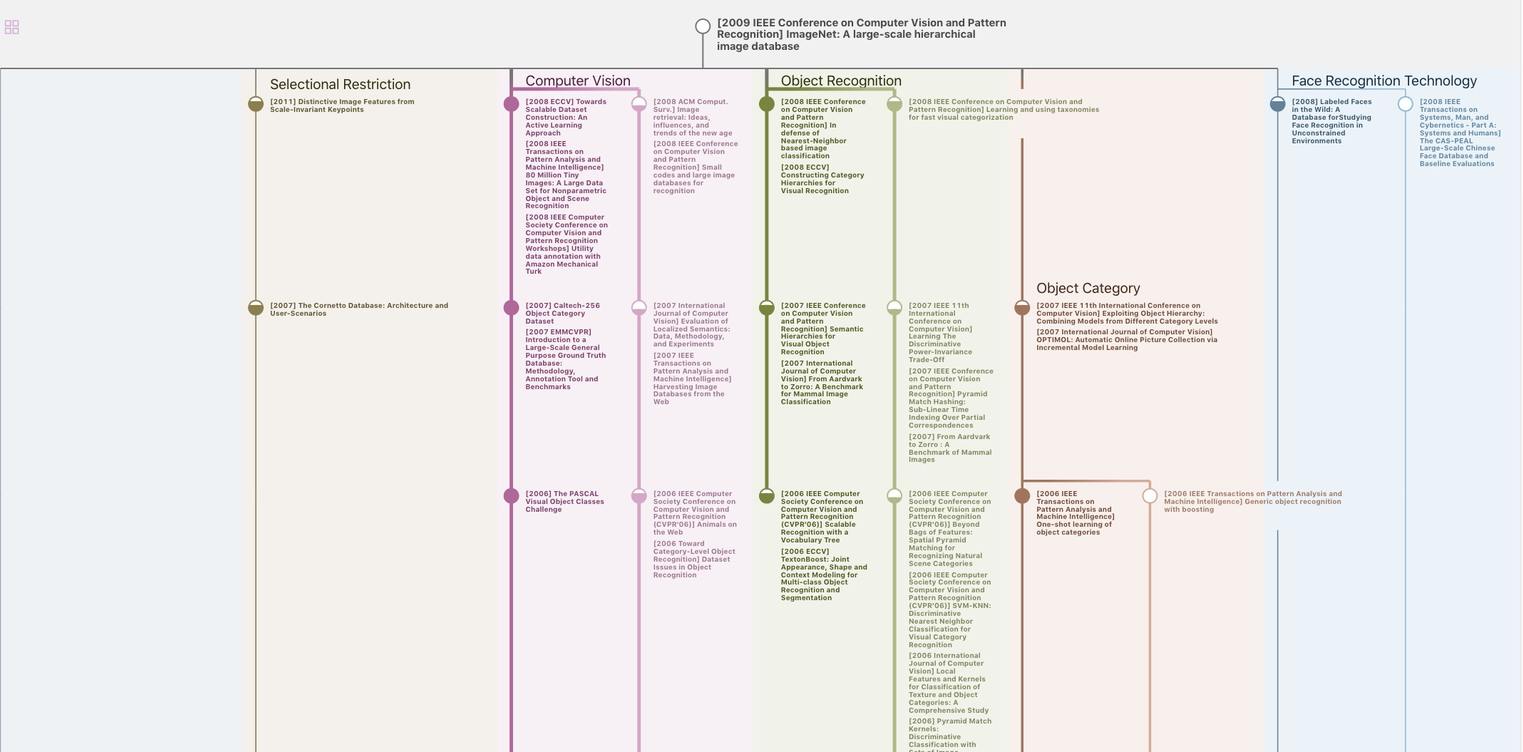
生成溯源树,研究论文发展脉络
Chat Paper
正在生成论文摘要