Bilateral Improvement in Local Personalization and Global Generalization in Federated Learning
IEEE Internet of Things Journal(2024)
摘要
Federated Learning (FL) is a machine learning paradigm where a server trains a global model by amalgamating contributions from multiple clients, without accessing personal client data directly. Personalized Federated Learning (PFL), a specific subset of this domain, shifts focus from a global model to providing personalized models for each client. This difference in training objectives signifies that while conventional FL aims for optimal generalization at the server level, PFL focuses on client-side model personalization. Often, achieving both generalization and personalization in a model is challenging. In response, we introduce FedCACS, a Classifier Aggregation with Cosine Similarity in Federated Learning method to bridge the gap between conventional FL and PFL. On the one hand, FedCACS adopts cosine similarity and a new PFL training strategy, which enhances the personalization ability of the local model on the client and enables the model to learn more compact image representation. On the other hand, FedCACS uses a classifier aggregation module to aggregate personalized classifiers from each client to restore the generalization ability of the global model. Experiments on public datasets affirm the effectiveness of FedCACS in personalization, generalization ability, and fast adaptation.
更多查看译文
关键词
Federated learning,personalized federated learning,fine-tuning,cosine similarity
AI 理解论文
溯源树
样例
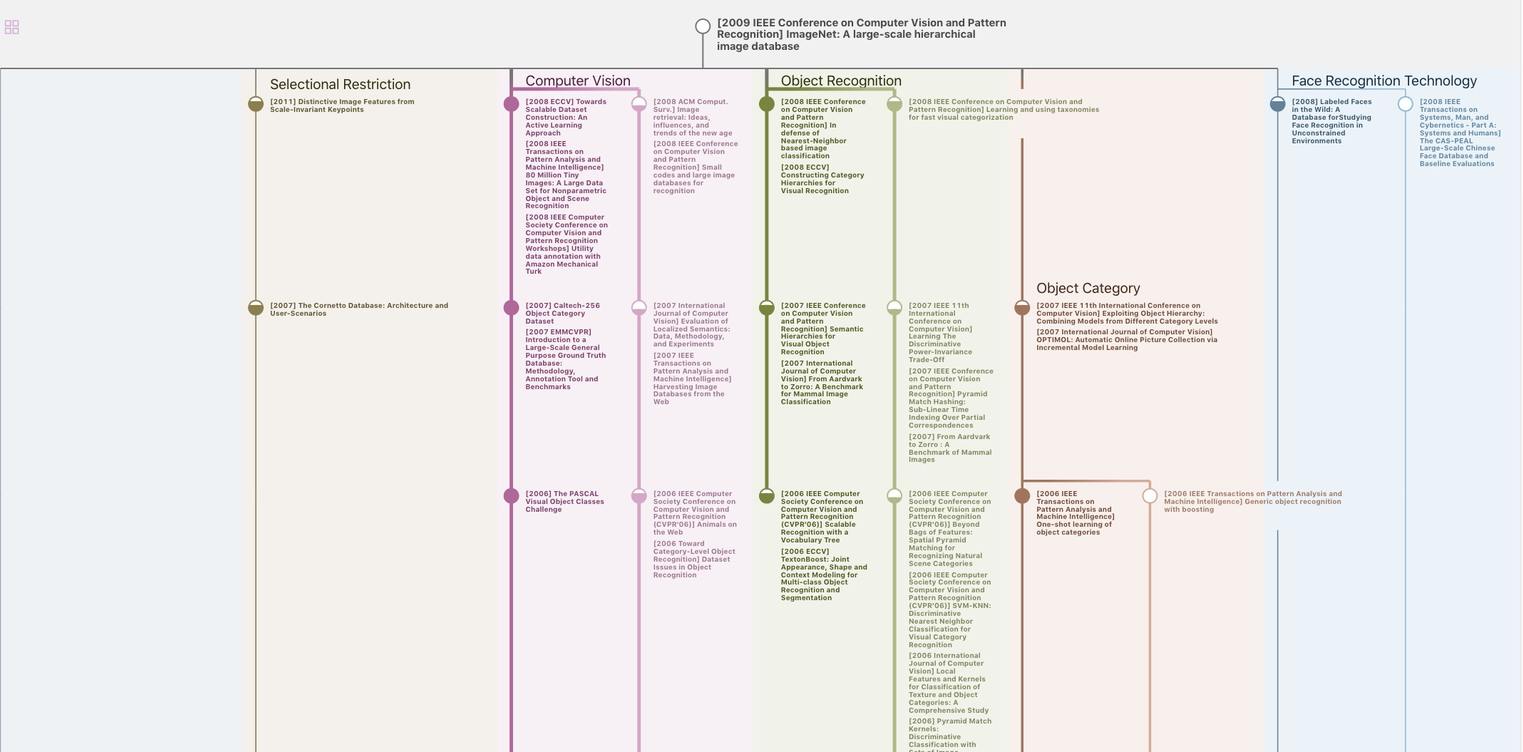
生成溯源树,研究论文发展脉络
Chat Paper
正在生成论文摘要