DC-YOLO: Improved YOLOv7 Based on Deformable Convolution and Attention Mechanism for Fabric Defect Detection.
International Conference on Advanced Cloud and Big Data(2023)
摘要
Fabric defect detection plays a crucial role in the fabric production process. To accurately identify and locate the defect points in the fabric, we proposed a fabric defect detection algorithm DC-YOLO based on YOLOv7. Firstly, according to the size distribution of defect boxes in the dataset, we use K-means algorithm to cluster boxes in the dataset, obtaining anchor boxes with 9 different sizes based on their distribution. Then, the DCN-bottleneck module fused with deformable convolution is introduced into the backbone to enhance the network's feature extraction ability for irregular shape defects. Finally, the down-sampling module MP was replaced with the MPCA module integrating the coordinate attention to alleviate the loss of key spatial information caused by down-sampling. Comparative experiments show that the improved YOLOv7 algorithm is superior to the current mainstream object detection algorithms in terms of mAP (Mean Average Precision). Compared with the original YOLOv7 algorithm, our method improves the mAP by 15.43%. On the whole, the improved YOLOv7 algorithm has better detection accuracy, and it is of great significance to promote the automatic detection of fabric defects.
更多查看译文
关键词
deep learning,defect detection,YOLOv7,deformable convolution
AI 理解论文
溯源树
样例
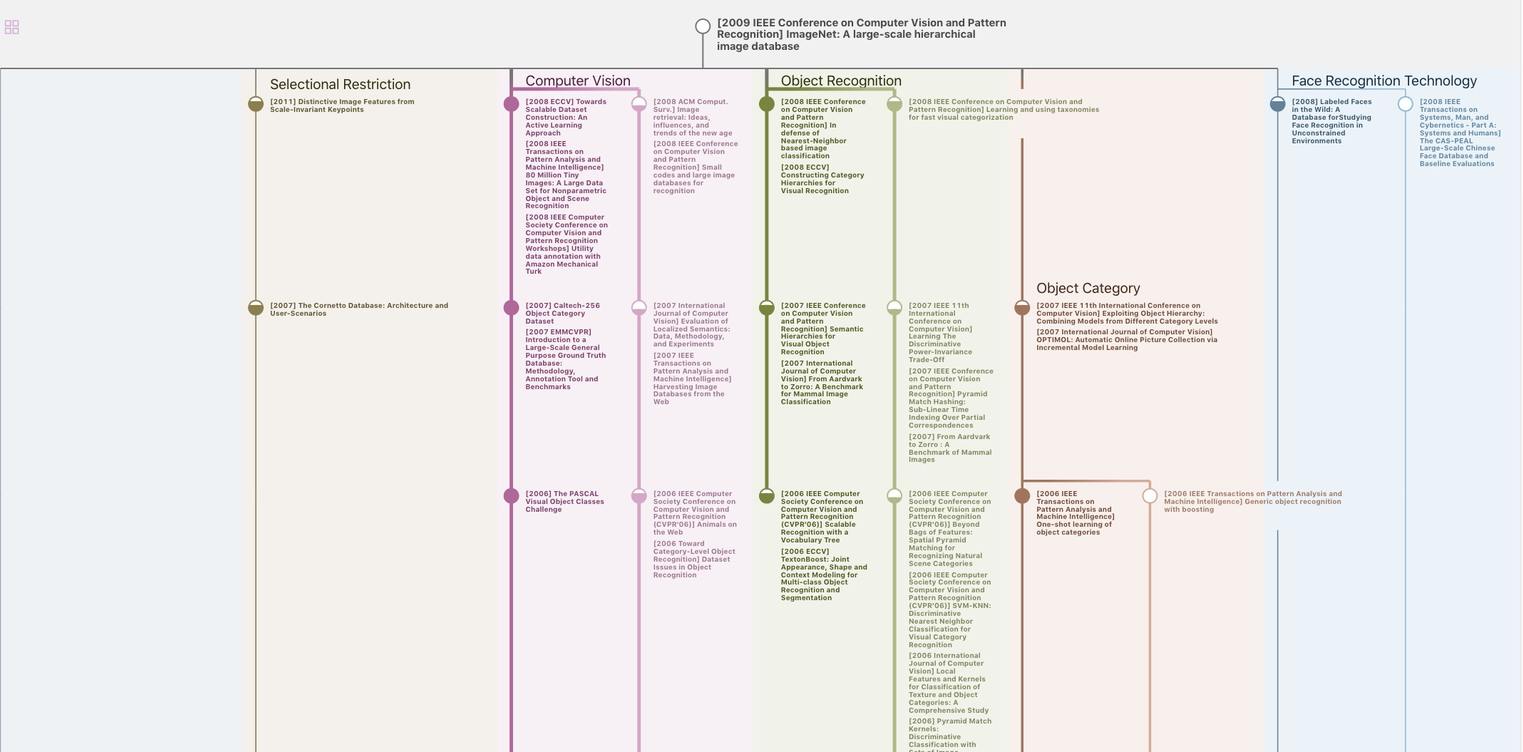
生成溯源树,研究论文发展脉络
Chat Paper
正在生成论文摘要