Resolving Ambiguities in Text-to-Image Generative Models
PROCEEDINGS OF THE 61ST ANNUAL MEETING OF THE ASSOCIATION FOR COMPUTATIONAL LINGUISTICS (ACL 2023): LONG PAPERS, VOL 1(2023)
摘要
Natural language often contains ambiguities that can lead to misinterpretation and miscommunication. While humans can handle ambiguities effectively by asking clarifying questions and/or relying on contextual cues and commonsense knowledge, resolving ambiguities can be notoriously hard for machines. In this work, we study ambiguities that arise in text-to-image generative models. We curate the Text-toimage Ambiguity Benchmark (TAB) dataset to study different types of ambiguities in text-to-image generative models.1 We then propose the Text-to-ImagE Disambiguation (TIED) framework to disambiguate the prompts given to the text-to-image generative models by soliciting clarifications from the end user. Through automatic and human evaluations, we show the effectiveness of our framework in generating more faithful images aligned with end user intention in the presence of ambiguities.
更多查看译文
AI 理解论文
溯源树
样例
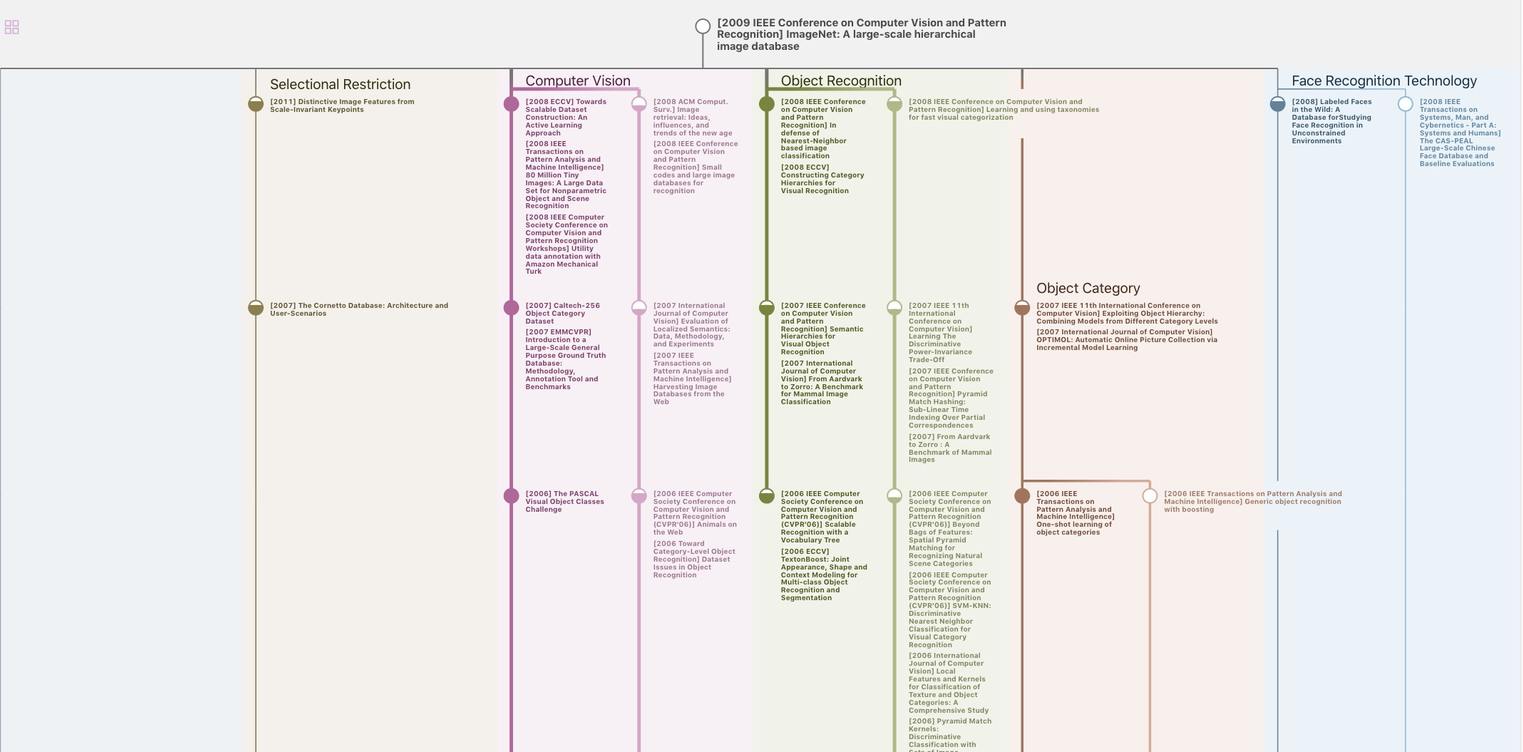
生成溯源树,研究论文发展脉络
Chat Paper
正在生成论文摘要