Deep Incubation: Training Large Models by Divide-and-Conquering
2023 IEEE/CVF INTERNATIONAL CONFERENCE ON COMPUTER VISION (ICCV 2023)(2023)
摘要
Recent years have witnessed a remarkable success of large deep learning models. However, training these models is challenging due to high computational costs, painfully slow convergence, and overfitting issues. In this paper, we present Deep Incubation, a novel approach that enables the efficient and effective training of large models by dividing them into smaller sub-modules which can be trained separately and assembled seamlessly. A key challenge for implementing this idea is to ensure the compatibility of the independently trained sub-modules. To address this issue, we first introduce a global, shared meta model, which is leveraged to implicitly link all the modules together, and can be designed as an extremely small network with negligible computational overhead. Then we propose a module incubation algorithm, which trains each sub-module to replace the corresponding component of the meta model and accomplish a given learning task. Despite the simplicity, our approach effectively encourages each sub-module to be aware of its role in the target large model, such that the finally-learned sub-modules can collaborate with each other smoothly after being assembled. Empirically, our method can outperform end-to-end (E2E) training in well-established training setting and shows transferable performance gain for downstream tasks (e.g., object detection and image segmentation on COCO and ADE20K). Our code is available at https://github.com/LeapLabTHU/Deep-Incubation.
更多查看译文
AI 理解论文
溯源树
样例
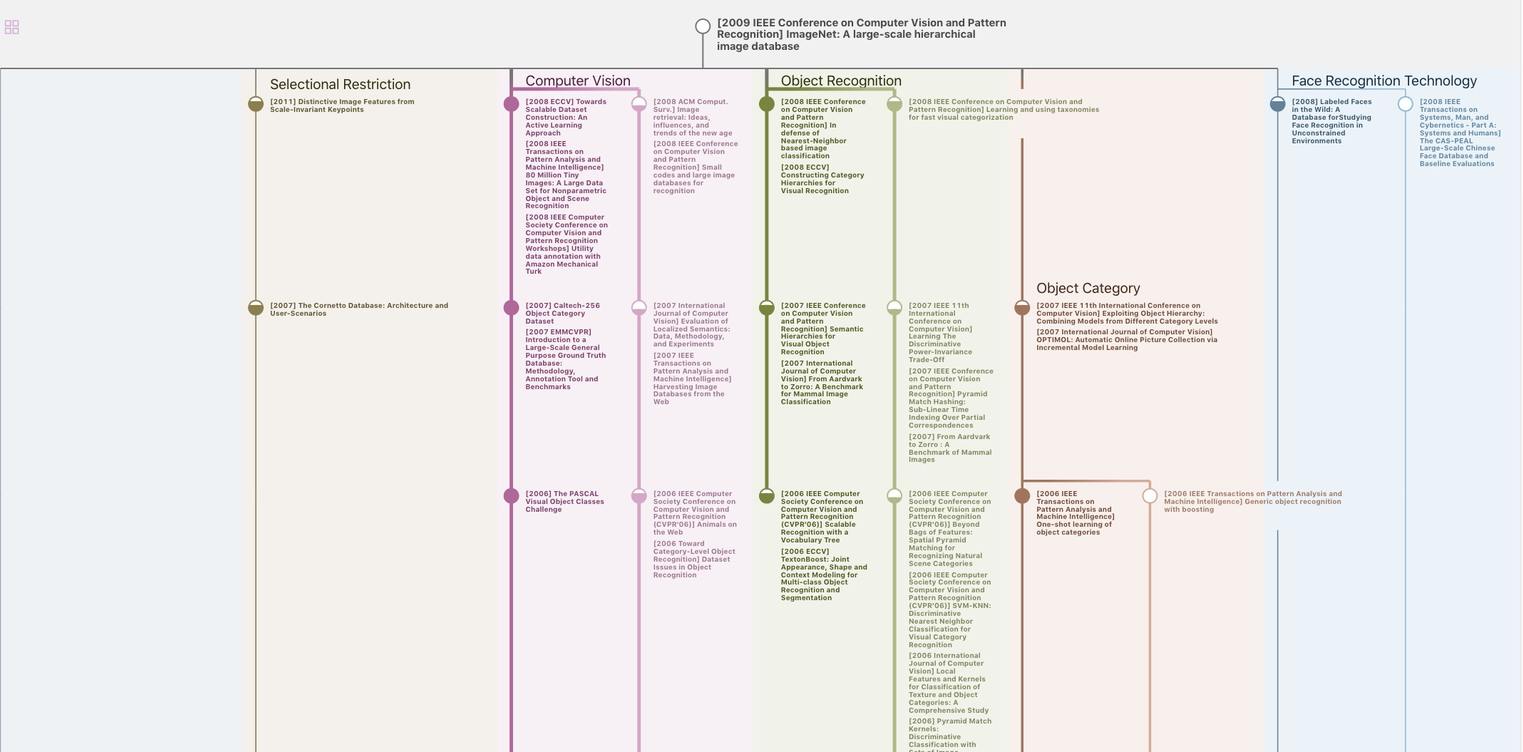
生成溯源树,研究论文发展脉络
Chat Paper
正在生成论文摘要