Msdra-net: a multi-scale attention-guided network for magnetic resonance image restoration
JOURNAL OF MECHANICS IN MEDICINE AND BIOLOGY(2024)
摘要
The acquisition and transmission of magnetic resonance (MR) images are frequently affected by random noise pollution, which hampers the diagnosis of diseases by doctors or automated systems. Hence, the search for advanced denoising methods is of great research interest, particularly in magnetic resonance imaging (MRI) denoising models, which are based on deep learning networks and achieve satisfactory results. However, the mining of noisy contextual information and effective information-guided transfer are often neglected in the denoising process, which leads to poor extraction of information at different scales, and poor retention of details. This greatly hinders the further development of MR image denoising methods. Here, we propose a denoising method, MSDRA-Net, for the mining and exploitation of different hierarchical noise features and construct a multi-scale dilated residual (MSDR) structure to transfer and retain noise information at different levels across the layer. Next, a contextual guidance attention (CGA) module guides and transfers contextual information, utilizing the features learnt from different layers of the model as weights. A reconstruction refinement block (RRB) is utilized to construct clean images from the obtained noise bias and the given noisy images. Experiments on simulated and clinical MRI data validated the effectiveness of our method, which demonstrated a superior performance compared to several state-of-the-art methods.
更多查看译文
关键词
Magnetic resonance imaging,denoising,multi-scale,dilation convolution,deep learning
AI 理解论文
溯源树
样例
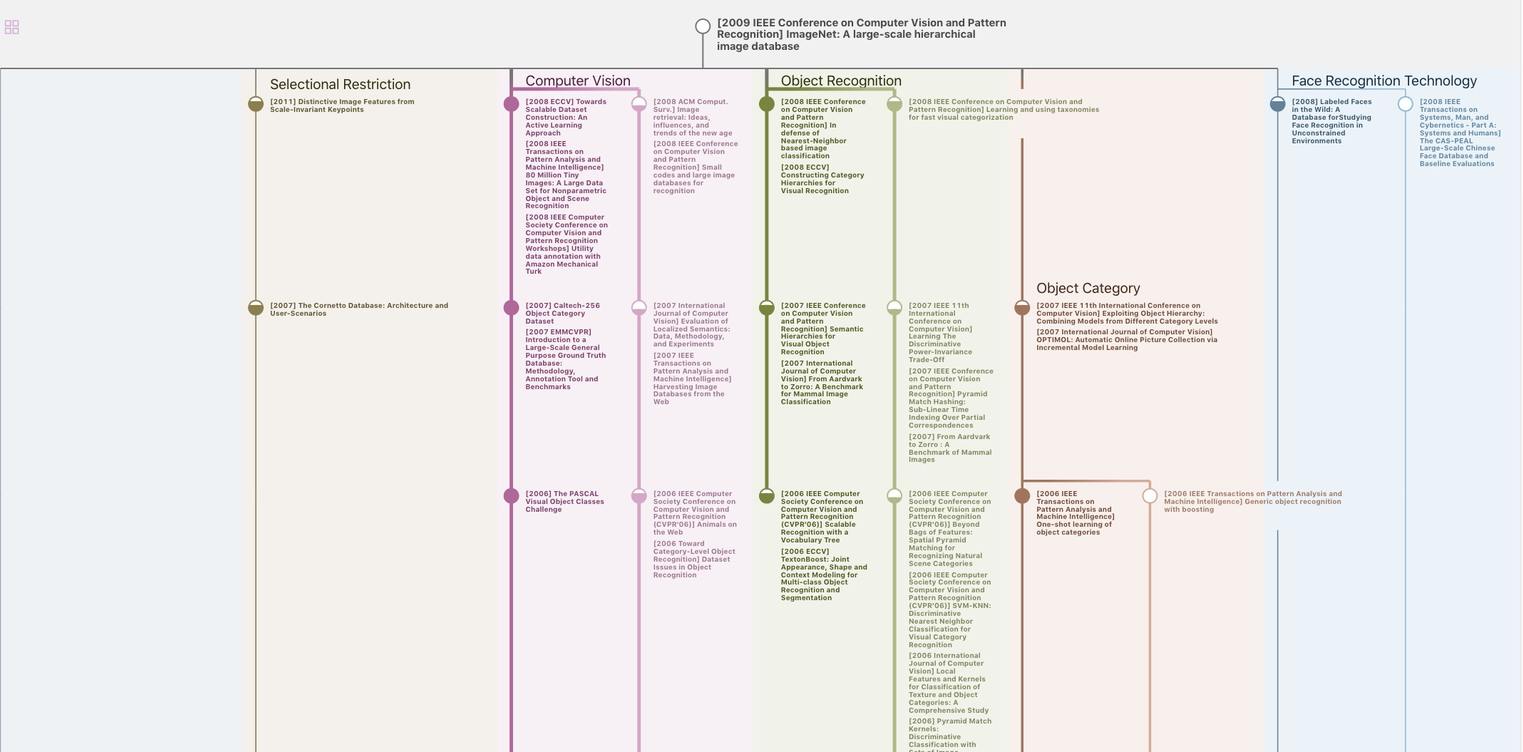
生成溯源树,研究论文发展脉络
Chat Paper
正在生成论文摘要