Zero-shot LLM-guided Counterfactual Generation for Text
arxiv(2024)
摘要
Counterfactual examples are frequently used for model development and
evaluation in many natural language processing (NLP) tasks. Although methods
for automated counterfactual generation have been explored, such methods depend
on models such as pre-trained language models that are then fine-tuned on
auxiliary, often task-specific datasets. Collecting and annotating such
datasets for counterfactual generation is labor intensive and therefore,
infeasible in practice. Therefore, in this work, we focus on a novel problem
setting: zero-shot counterfactual generation. To this end, we propose
a structured way to utilize large language models (LLMs) as general purpose
counterfactual example generators. We hypothesize that the
instruction-following and textual understanding capabilities of recent LLMs can
be effectively leveraged for generating high quality counterfactuals in a
zero-shot manner, without requiring any training or fine-tuning. Through
comprehensive experiments on various downstream tasks in natural language
processing (NLP), we demonstrate the efficacy of LLMs as zero-shot
counterfactual generators in evaluating and explaining black-box NLP models.
更多查看译文
AI 理解论文
溯源树
样例
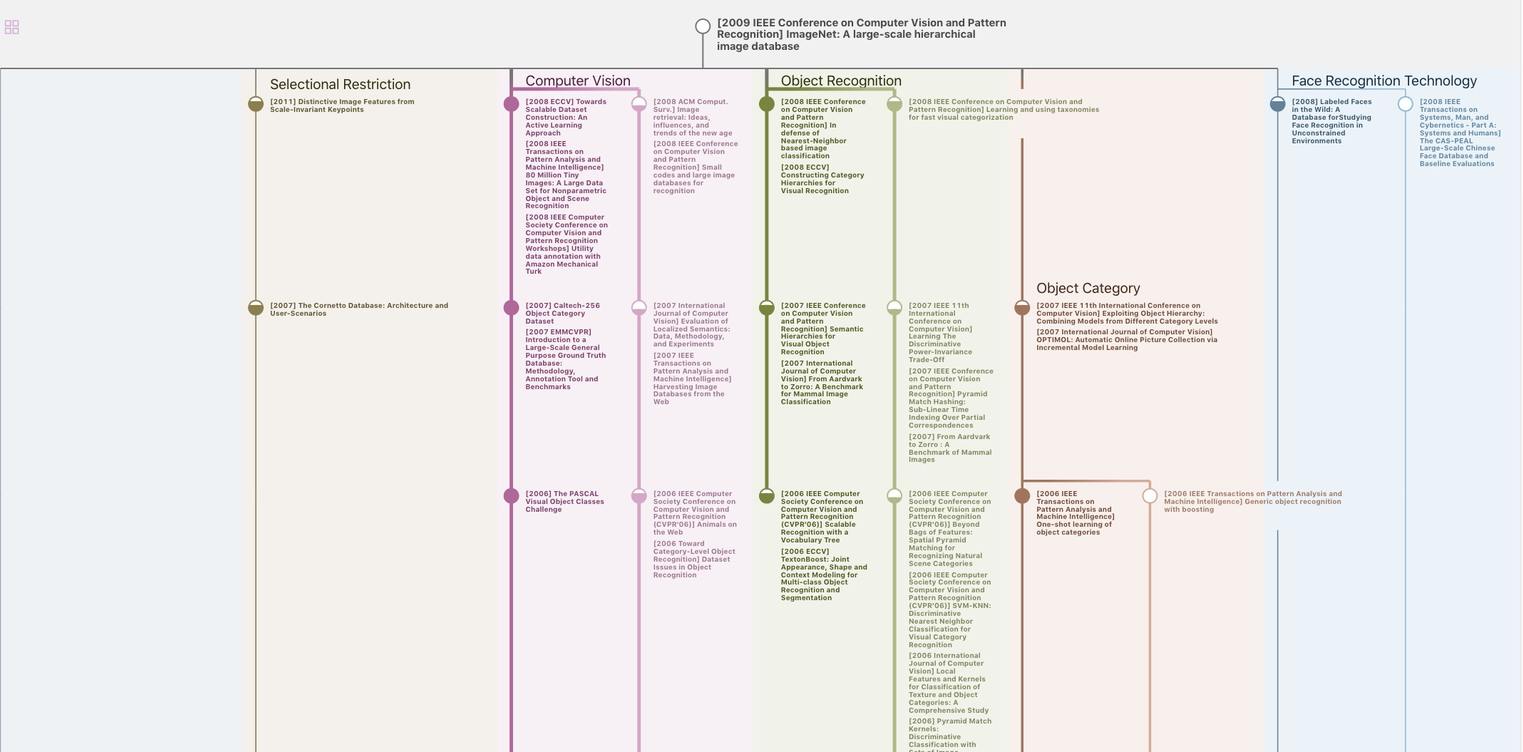
生成溯源树,研究论文发展脉络
Chat Paper
正在生成论文摘要