Online Recognition of Manually Spray-printed Ladle Numbers in Complex Dynamic Environments
IEEE Transactions on Instrumentation and Measurement(2024)
摘要
During the converter process, it is crucial to automatically identify and record ladle numbers to track steel product quality and enhance automation levels. However, the steelmaking environment presents several challenges, including intricate ladle scheduling, varying lighting conditions, severe background interference, and significant disparities between manually spray-printed ladle number characteristics and publicly available datasets. The combination of these problems makes it challenging to perform accurate and real-time ladle number identification. In response, this paper suggests an automatic ladle number recognition approach based on deep learning and image processing. Firstly, a double-region object detection model based on YOLOv5 is employed to capture keyframe images of the ladle to be identified from the video stream. Then, a method that can enable the acquisition of an accurate region of ladle numbers in sophisticated industrial settings is proposed to address the distortion of numerical features caused by lighting variations and background interference in industrial environments. Lastly, leveraging the proprietary dataset founded, a ladle number recognition model integrating CNN and multi-frame image fusion is designed, developing multithreading design and image queue management to ensure real-time and accurate ladle number recognition. In this study, the video data of a steel plant is used for testing. Through testing 176 steelmaking production cycles, all ladle numbers are accurately identified prior to finishing charging molten iron, indicating the high accuracy and real-time capability of the recognition system.
更多查看译文
关键词
Ladle number recognition,complex dynamic environments,online recognition,image processing,deep learning
AI 理解论文
溯源树
样例
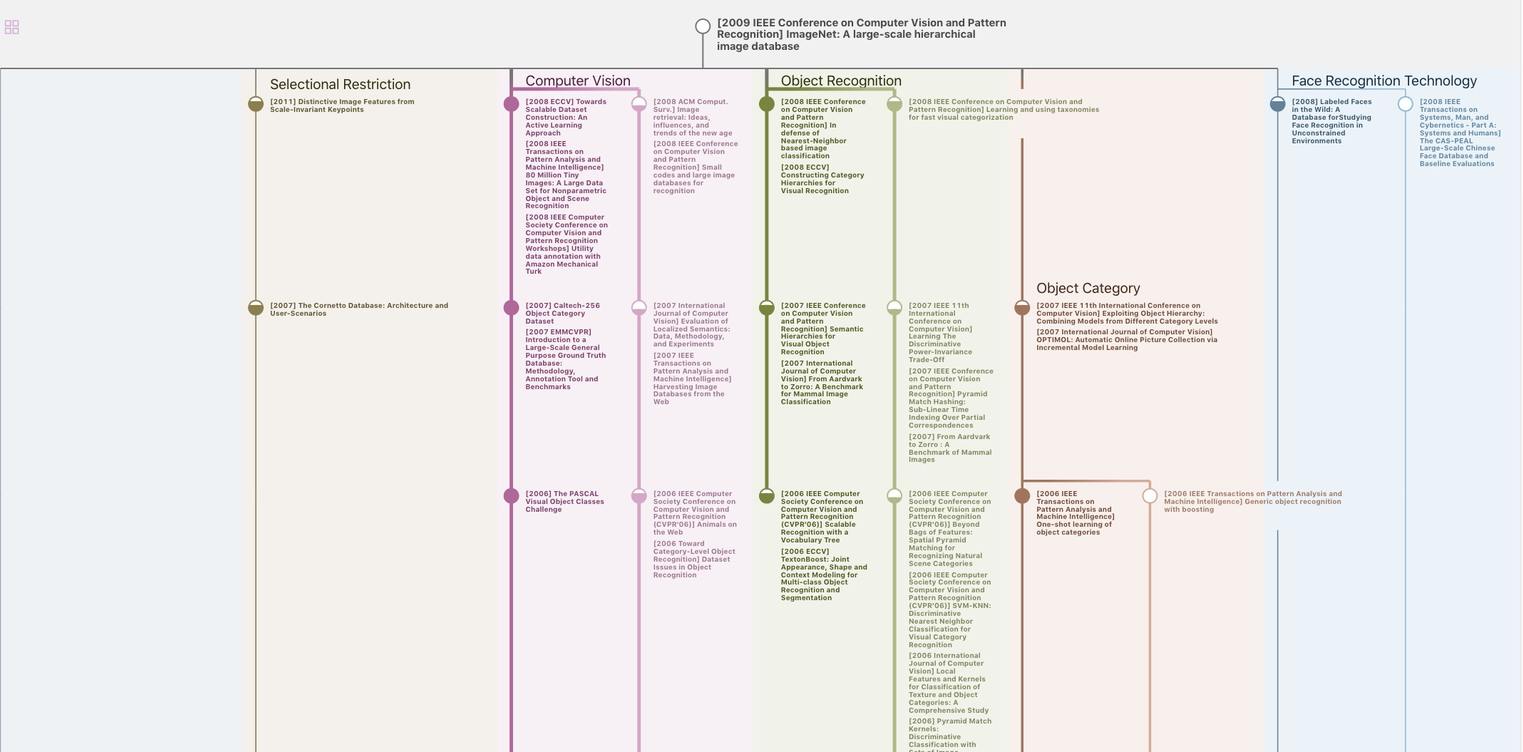
生成溯源树,研究论文发展脉络
Chat Paper
正在生成论文摘要