Toward Energy-Efficiency: Integrating DRL and Ze-RIS for Task Offloading in UAV-MEC Environments
IEEE access(2024)
摘要
Unmanned aerial vehicles (UAVs) play an important role within mobile edge computing (MEC) networks in improving communications for ground users during emergency situations. However, sustaining high-quality service for extended periods is challenging because of constraints on battery capacity and computing capabilities of UAVs. To address this issue, we leverage zero-energy reconfigurable intelligent surfaces (ze-RIS) within UAV-MEC networks and introduce a comprehensive strategy that combines task offloading and resource sharing. A deep reinforcement learning (DRL) driven energy efficient task offloading (DEETO) scheme is presented. The primary objective is to minimize UAV energy ingestion. DEETO aims to enhance task offloading decision mechanism, computing and communication resource allocation, while adopting hybrid task offloading mechanism with intelligent RIS phase-shift control. We begin by modeling it as a DRL problem, structuring it as a Markov decision process (MDP), and subsequently resolving it effectively through the use of the advantage actor-critic (A2C) algorithm. Our simulation results highlight the superiority of the DEETO scheme compared to alternative approaches. DEETO excelled by achieving a notable energy savings of 16.98% from the allocated energy resources, coupled with the highest task turnover rate of 94.12%, all achieved within a shorter learning time frames per second (TFPS) and yielding higher rewards.
更多查看译文
关键词
DRL,MEC,task offloading,UAV,ze-RIS
AI 理解论文
溯源树
样例
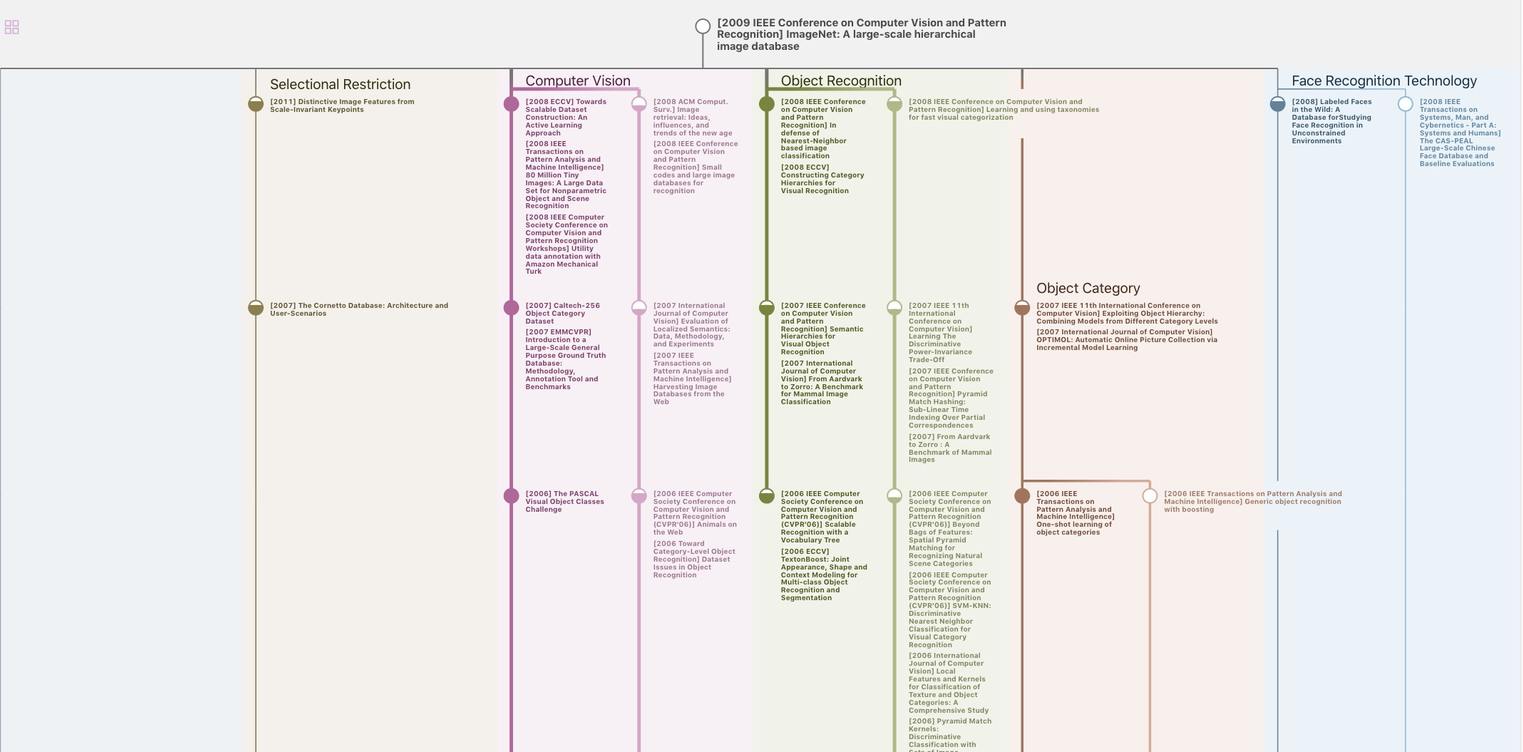
生成溯源树,研究论文发展脉络
Chat Paper
正在生成论文摘要