Sparsify dynamically expandable network via variational dropout
Applied Soft Computing(2024)
摘要
This paper develops a new method for lifelong learning referred to as Sparsify Dynamically Expandable Network (SDEN) via Variational Dropout, which explores a sparse model while preserving the performance. Dynamically Expandable Network (DEN) can learn a sequence of tasks via performing network retraining, network expansion by adding only the necessary neurons, and network split to effectively prevent semantic drift in an online manner. To overcome point estimation of parameters in DEN, Bayesian Compression for DEN is developed under the Bayesian framework. However, this method demands more time for model training and testing. To improve the model efficiency, we propose SDEN under the efficient sparse learning framework. We validate our SDEN in the lifelong learning scenarios with multiple frequently used benchmarks, on which it can obtain comparable classification accuracy, and less training and testing time compared with the comparison methods. Furthermore, our method can also learn a more sparse network structure which means fewer network parameters.
更多查看译文
关键词
Variational dropout,Dynamically expandable network,Lifelong learning,Network retraining,Network expansion,Catastrophic forgetting or semantic drift
AI 理解论文
溯源树
样例
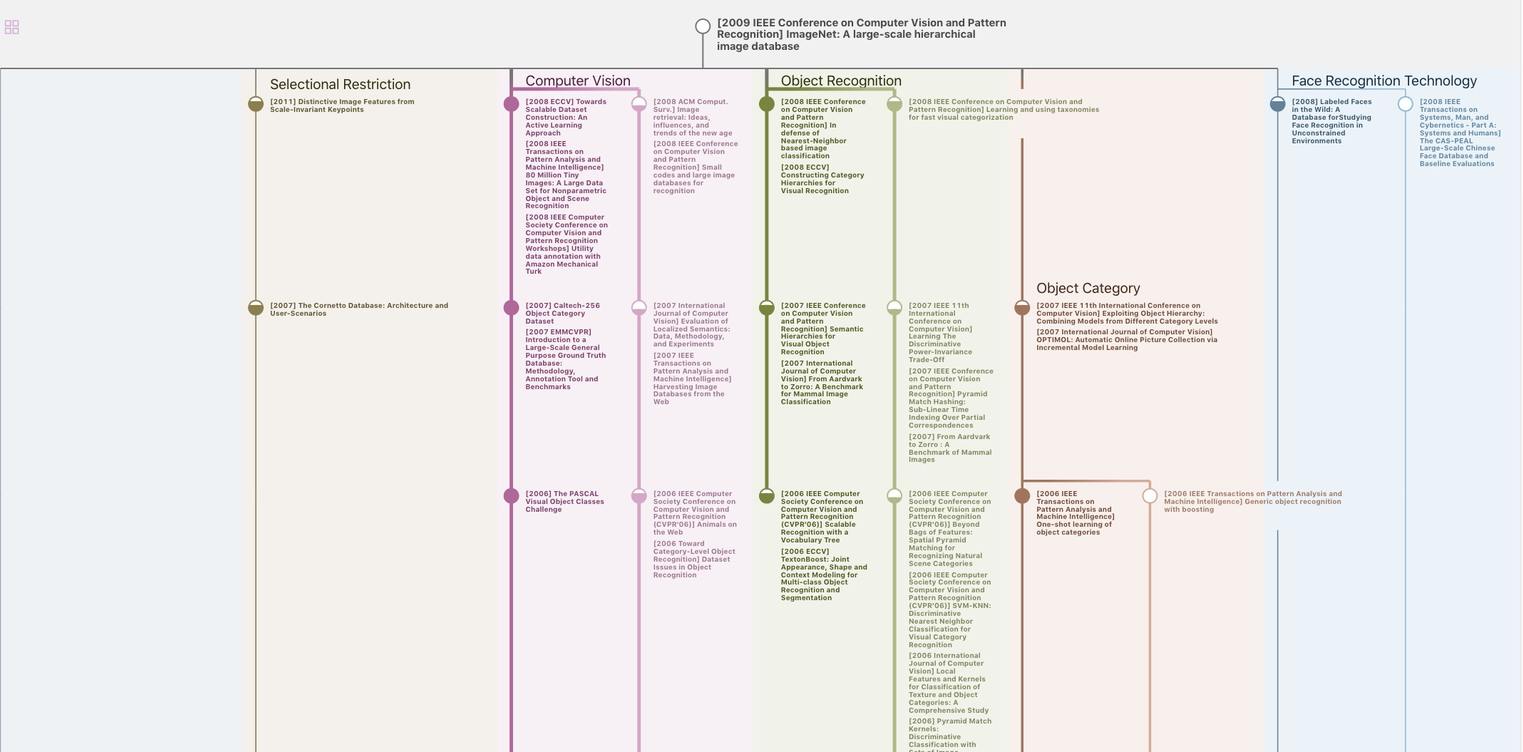
生成溯源树,研究论文发展脉络
Chat Paper
正在生成论文摘要