One Nose but Two Nostrils: Learn to Align with Sparse Connections Between Two Olfactory Cortices
ArXiv(2024)
摘要
The integration of neural representations in the two hemispheres is animportant problem in neuroscience. Recent experiments revealed that odorresponses in cortical neurons driven by separate stimulation of the twonostrils are highly correlated. This bilateral alignment points to structuredinter-hemispheric connections, but detailed mechanism remains unclear. Here, wehypothesized that continuous exposure to environmental odors shapes theseprojections and modeled it as online learning with local Hebbian rule. We foundthat Hebbian learning with sparse connections achieves bilateral alignment,exhibiting a linear trade-off between speed and accuracy. We identified aninverse scaling relationship between the number of cortical neurons and theinter-hemispheric projection density required for desired alignment accuracy,i.e., more cortical neurons allow sparser inter-hemispheric projections. Wenext compared the alignment performance of local Hebbian rule and the globalstochastic-gradient-descent (SGD) learning for artificial neural networks. Wefound that although SGD leads to the same alignment accuracy with modestlysparser connectivity, the same inverse scaling relation holds. We showed thattheir similar performance originates from the fact that the update vectors ofthe two learning rules align significantly throughout the learning process.This insight may inspire efficient sparse local learning algorithms for morecomplex problems.
更多查看译文
AI 理解论文
溯源树
样例
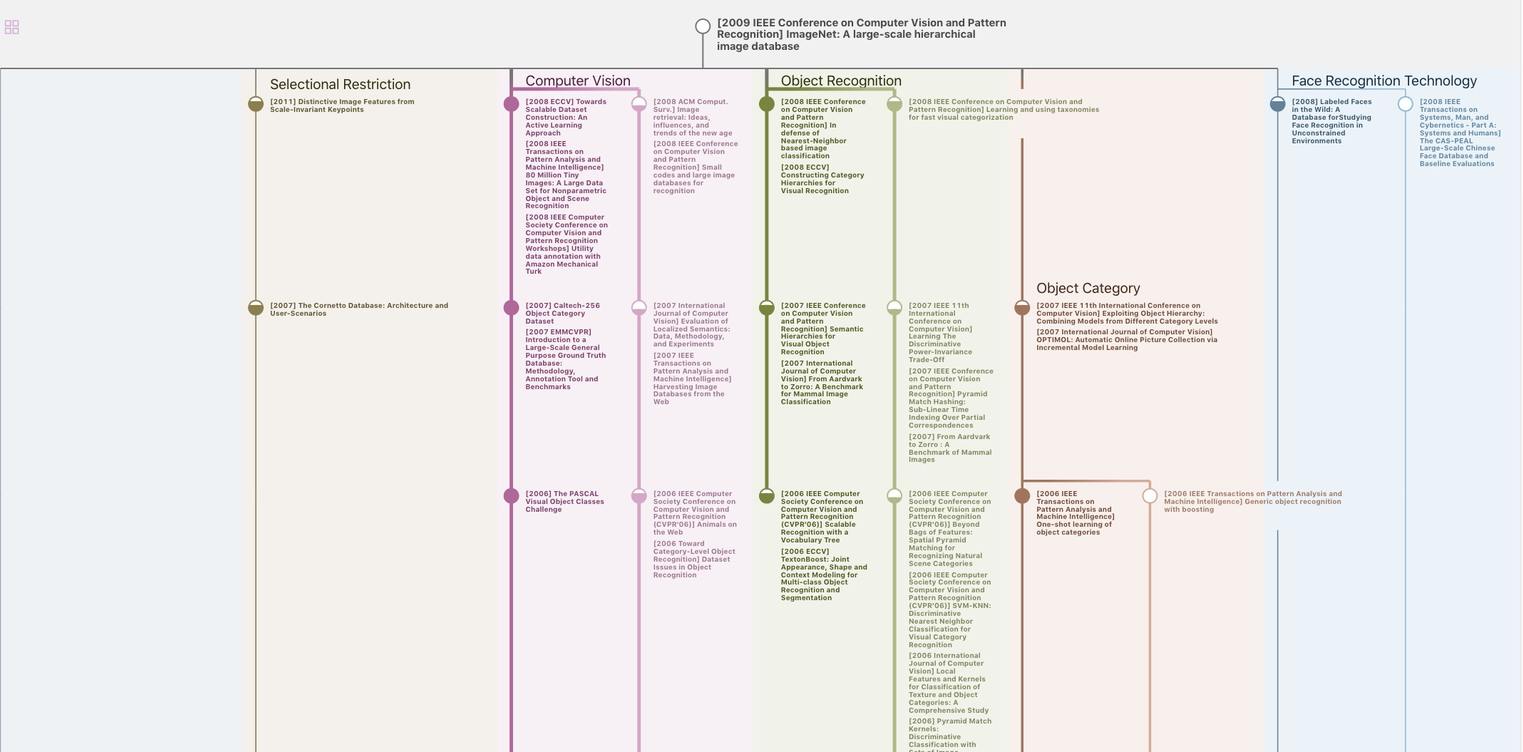
生成溯源树,研究论文发展脉络
Chat Paper
正在生成论文摘要