Enhancing Contextual Understanding in Large Language Models Through Contrastive Decoding
NAACL-HLT(2024)
Abstract
Large language models (LLMs) tend to inadequately integrate input contextduring text generation, relying excessively on encoded prior knowledge in modelparameters, potentially resulting in generated text with factualinconsistencies or contextually unfaithful content. LLMs utilize two primaryknowledge sources: 1) prior (parametric) knowledge from pretraining, and 2)contextual (non-parametric) knowledge from input prompts. The study addressesthe open question of how LLMs effectively balance these knowledge sourcesduring the generation process, specifically in the context of open-domainquestion answering. To address this issue, we introduce a novel approachintegrating contrastive decoding with adversarial irrelevant passages asnegative samples to enhance robust context grounding during generation.Notably, our method operates at inference time without requiring furthertraining. We conduct comprehensive experiments to demonstrate its applicabilityand effectiveness, providing empirical evidence showcasing its superiority overexisting methodologies. Our code is publicly available at:https://github.com/amazon-science/ContextualUnderstanding-ContrastiveDecoding.
MoreTranslated text
AI Read Science
Must-Reading Tree
Example
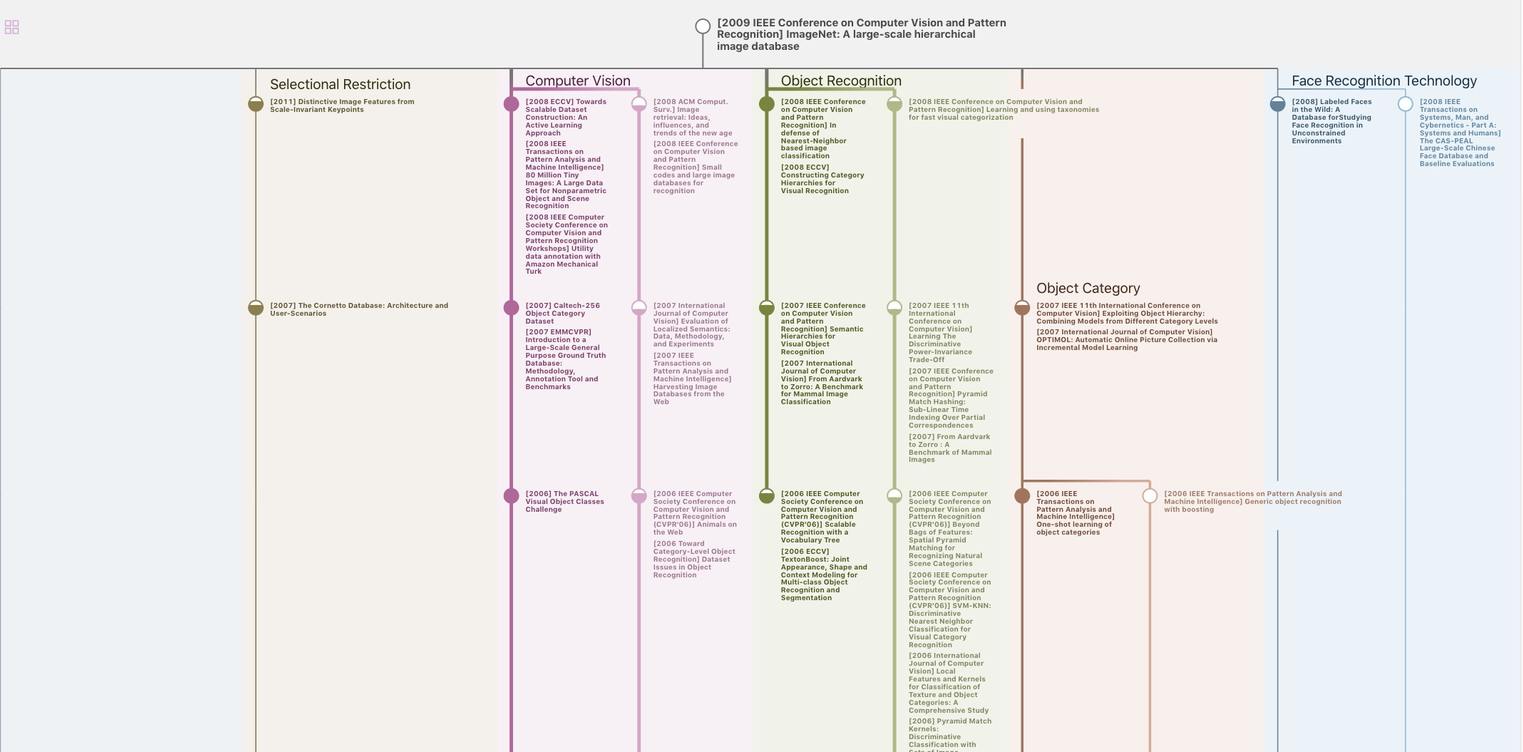
Generate MRT to find the research sequence of this paper
Chat Paper
Summary is being generated by the instructions you defined