Real-time County-Aggregated Wastewater-Based Estimates for SARS-CoV-2 Effective Reproduction Numbers
crossref(2024)
摘要
Background:The effective reproduction number ({R{e}}) serves as a metric of population-wide, time-varying disease spread. During the COVID-19 pandemic,{ R{e}}was primarily estimated from clinical surveillance data streams ({R{cc}}), which have varied in quality and representativeness due to changes in testing volume, test-seeking behavior, and resource constraints. Deriving{R{e}}from alternative data sources such as wastewater could inform future public health responses.Objectives:We estimated county-aggregated, sewershed-restricted wastewater-based SARS-CoV-2{R{e}}({R{ww}}) from May 1, 2022 to April 30, 2023 for five counties in California of varying population sizes, clinical testing rates, demographics, proportions surveilled by wastewater, and sampling frequencies to validate the reliability of{R{ww}}as a real-time disease surveillance metric.{Methods:}We produced both instantaneous and cohort sewershed-restricted{R{e}}using smoothed and deconvolved wastewater concentrations. We then population-weighted and aggregated these sewershed-level estimates to arrive at county-level{R{e}}. Using mean absolute error (MAE), Spearman's rank correlation (ρ), confusion matrix classification, and cross-correlation analyses, we compared the timing and trajectory of two{R{ww}}models to: (1) a publicly available, county-level ensemble of{R{cc}}estimates, and (2) a county-aggregated, sewershed-restricted{R{cc}}.{Results:}Both{R{ww}}models demonstrated high concordance with traditional{R{cc}}estimates, as indicated by low mean absolute errors (MAE ≤ 0.09), significant positive Spearman correlation (Spearman ρ ≥ 0.66, p < 0.001), and high confusion matrix classification accuracy (≥ 0.81). The relative timings of{R{ww}}and{R{cc}}were less clear, with cross-correlation analyses suggesting strong associations for a wide range of temporal lags that varied by county and{R{ww}}model type.{Discussion:}This{R{e}}estimation methodology provides a generalizable, robust, and operationalizable framework for estimating county-level{R{ww}}. Our results support the additional use of{R{ww}}as an epidemiological tool for surveillance. Based on this research, we produced publicly available{R{ww}}nowcasts for the California Communicable diseases Assessment Tool (https://calcat.covid19.ca.gov/cacovidmodels/).
更多查看译文
AI 理解论文
溯源树
样例
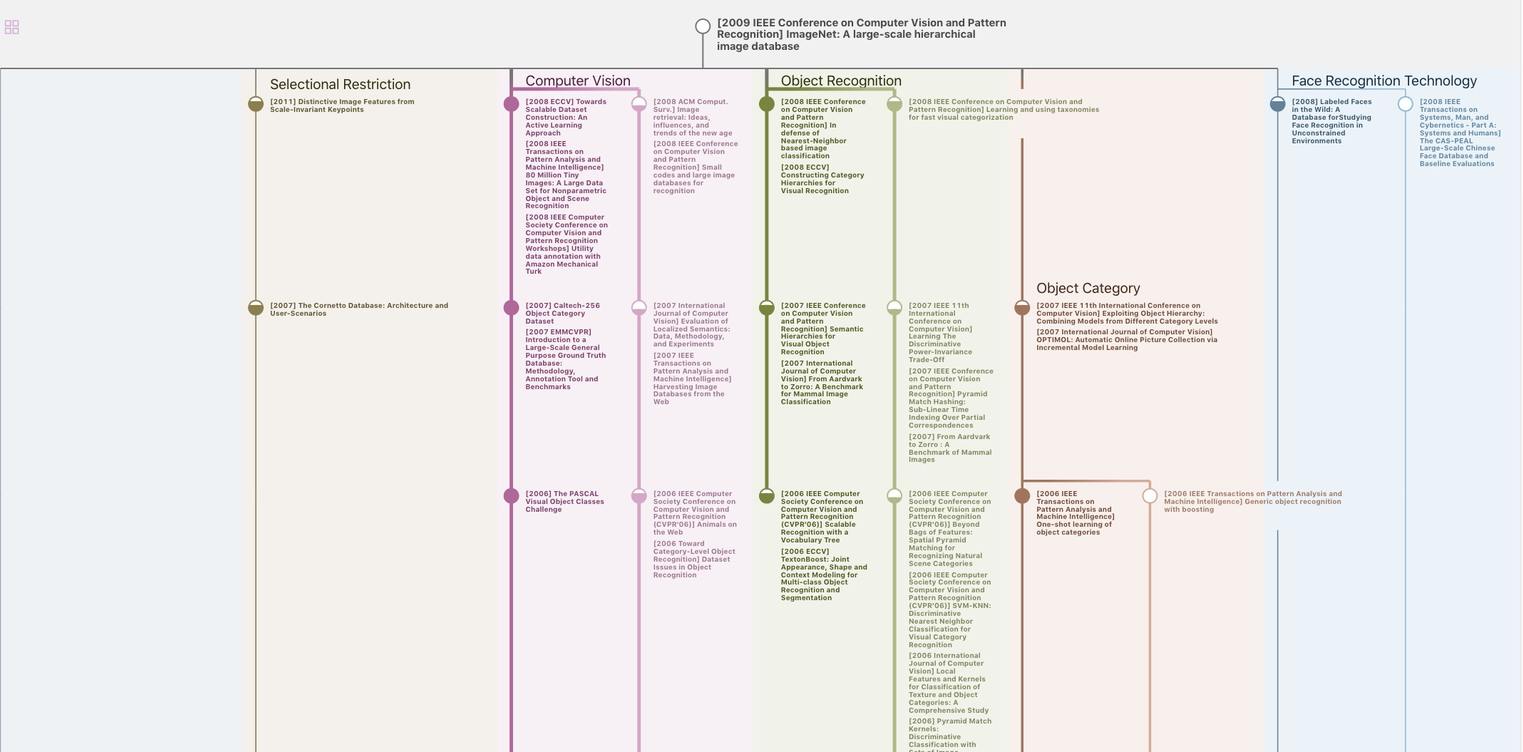
生成溯源树,研究论文发展脉络
Chat Paper
正在生成论文摘要