Distributed Nonconvex Optimization: Gradient-free Iterations and $\epsilon$ -Globally Optimal Solution
IEEE Transactions on Control of Network Systems(2024)
摘要
Distributed optimization utilizes local computation and communication to realize a global aim of optimizing the sum of local objective functions. This article addresses a class of constrained distributed nonconvex optimization problems involving univariate objectives, aiming to achieve global optimization without requiring local evaluations of gradients at every iteration. We propose a novel algorithm named CPCA, exploiting the notion of combining Chebyshev polynomial approximation, average consensus, and polynomial optimization. The proposed algorithm is i) able to obtain
$\epsilon$
-globally optimal solutions for any arbitrarily small given accuracy
$\epsilon$
, ii) efficient in both zeroth-order queries (i.e., evaluations of function values) and inter-agent communication, and iii) distributed terminable when the specified precision requirement is met. The key insight is to use polynomial approximations to substitute for general local objectives, distribute these approximations via average consensus, and solve an easier approximate version of the original problem. Due to the nice analytic properties of polynomials, this approximation not only facilitates efficient global optimization, but also allows the design of gradient-free iterations to reduce cumulative costs of queries and achieve geometric convergence for solving nonconvex problems. We provide a comprehensive analysis of the accuracy and complexities of the proposed algorithm.
更多查看译文
关键词
Distributed optimization,nonconvex optimization,consensus,Chebyshev polynomial approximation
AI 理解论文
溯源树
样例
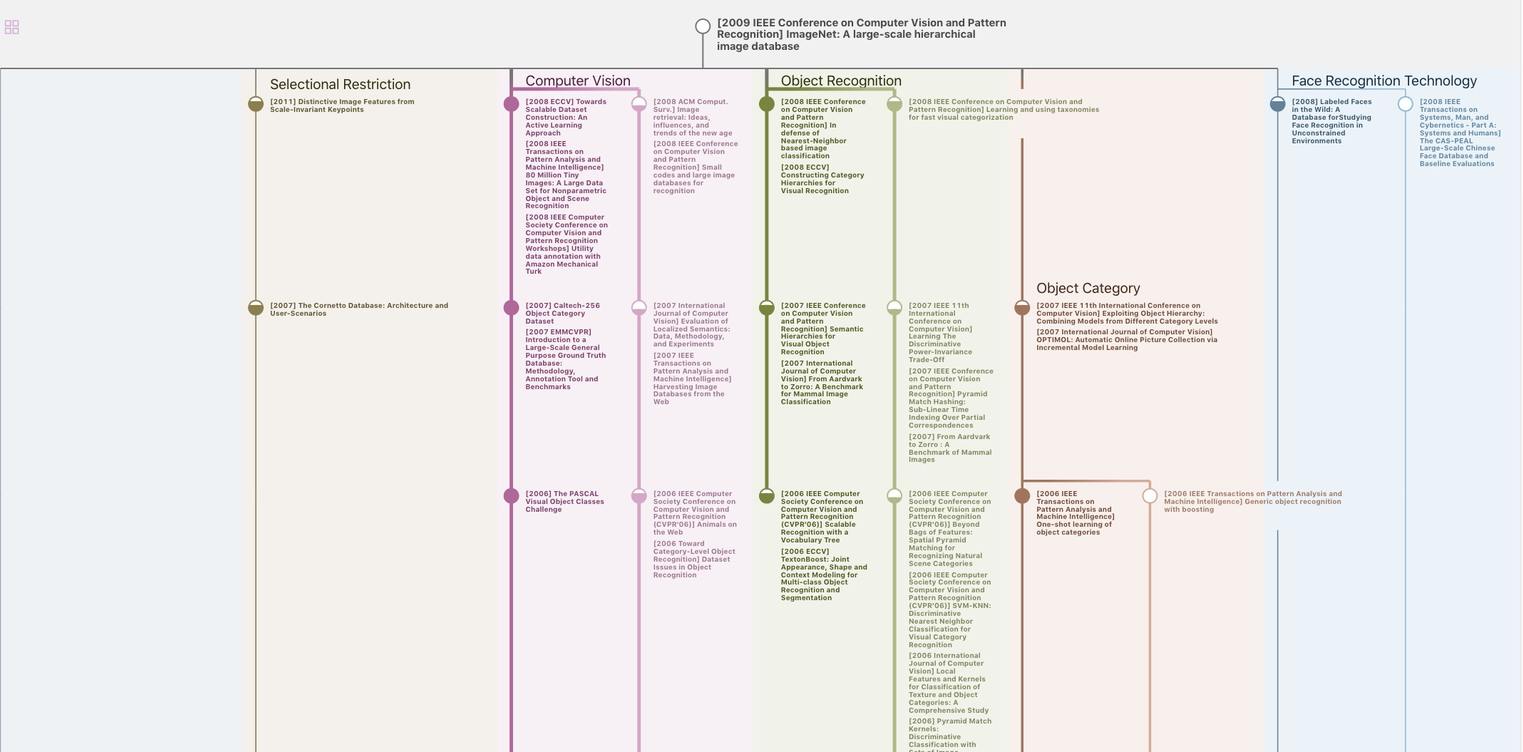
生成溯源树,研究论文发展脉络
Chat Paper
正在生成论文摘要