Fault Prediction and Awareness for Power Distribution in Grid Connected RES Using Hybrid Machine Learning
Electric power components and systems(2024)
摘要
This study addresses defects in electrical power systems, focusing on short circuits that can disrupt normal operation. The method emphasis is on hybrid microgrid systems connected to the grid, crucial for efficient power management. The novel method proposes an adaptive electricity management technique for fault scenarios in grid-tied conversions, minimizing sensor requirements. Power flow involves the hybrid energy storage system (HESS), renewable energy sources (RES), the electrical grid, and loads through a parallel hybrid RES arrangement. Semiconductor switch failures are common vulnerabilities, impacting grid functionality. The novel electricity management technique considers converter failures, renewable energy availability, and HESS state of charge restrictions. Power quality goals such as harmonic reduction, reactive power support, and a power factor of one are addressed. The proposed method was evaluated using MATLAB-based Simulink, showcasing its performance. The approach employs an Adaptive Power Management Strategy (APMS) in a hybrid renewable energy system, integrating wind and solar sources with battery and supercapacitor storage. A Hybrid Machine Learning-based Active Power Management System (HML-APMS) optimizes energy production, storage, and distribution for stability, reliability, and efficiency in the power utility system. The study underscores the significance of technology integration in achieving sustainable, reliable, and cost-effective power solutions.
更多查看译文
关键词
grid connected RES,HESS,hybrid machine learning,fault prediction and adaptive power management
AI 理解论文
溯源树
样例
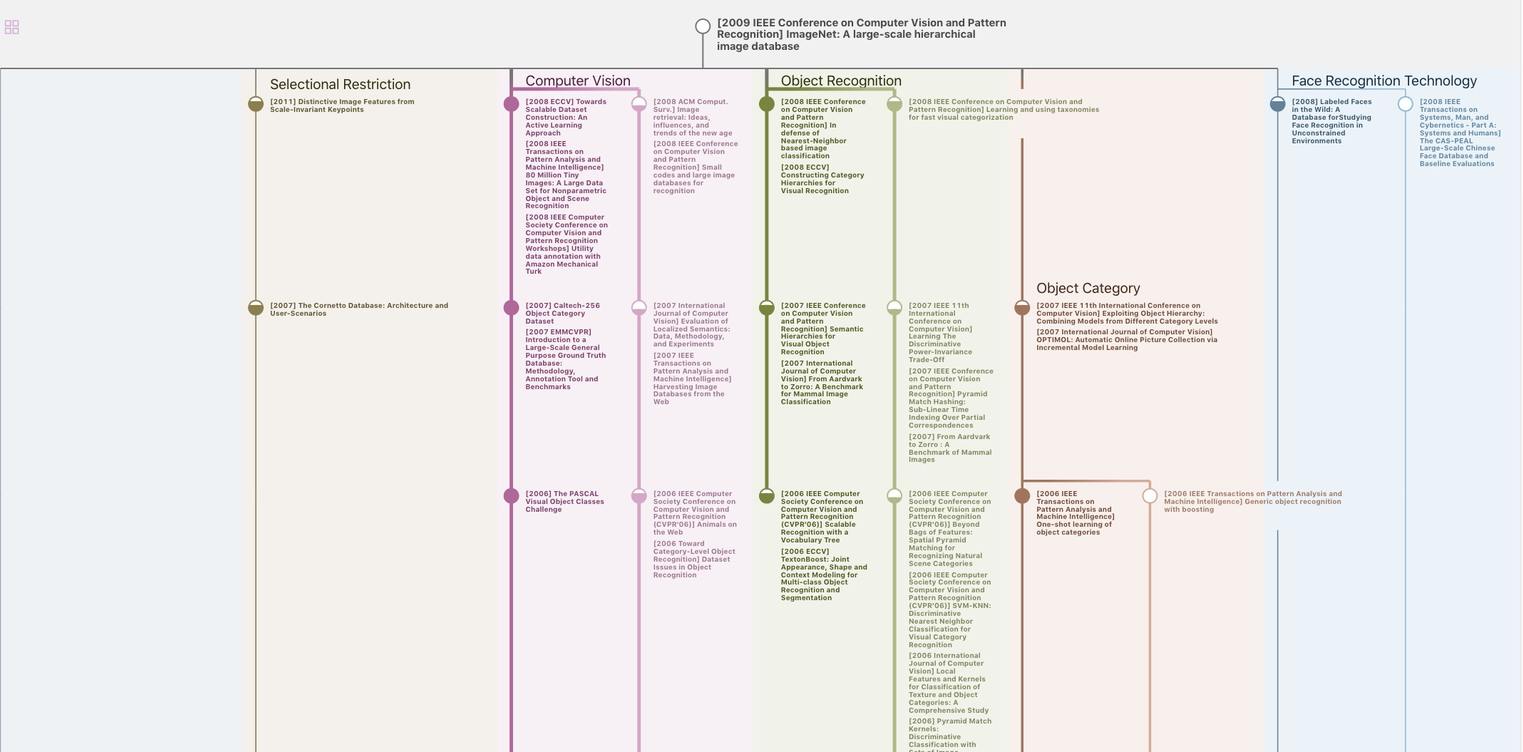
生成溯源树,研究论文发展脉络
Chat Paper
正在生成论文摘要