Making Informed Choices on Incorporating Chemoprevention into Care (michoice, SWOG 1904): Design and Methods of a Cluster Randomized Controlled Trial
CONTEMPORARY CLINICAL TRIALS(2024)
161 Ft Washington Ave | SWOG Stat & Data Management Ctr | Columbia Univ | Mayo Clin | Miami Canc Inst Baptist Hlth South Florida | Good Samaritan Hosp Corvallis | Carle Canc Ctr | Univ Utah | Holy Redeemer Hosp & Med Ctr | Brigham & Womens Hosp | City Hope Natl Med Ctr | Indiana Univ | Univ Hawaii | Mem Sloan Kettering Canc Ctr | Univ Wisconsin | Kaiser Permanente | Virginia Commonwealth Univ | Heartland NCORP | Sanford Roger Maris Canc Ctr | Baptist Mem Hlth Care | Aurora NCORP | Lahey Hosp & Med Ctr | Loyola Univ | Emory Univ Hosp | Providence Canc Inst | NCORP | Ohio State Univ | Fred Hutchinson Canc Ctr | Univ Texas MD Anderson Canc Ctr
Abstract
Introduction: Women with atypical hyperplasia (AH) or lobular carcinoma in situ (LCIS) have a significantly increased risk of breast cancer, which can be substantially reduced with antiestrogen therapy for chemoprevention. However, antiestrogen therapy for breast cancer risk reduction remains underutilized. Improving knowledge about breast cancer risk and chemoprevention among high -risk patients and their healthcare providers may enhance informed decision -making about this critical breast cancer risk reduction strategy. Methods/design: We are conducting a cluster randomized controlled trial to evaluate the effectiveness and implementation of patient and provider decision support tools to improve informed choice about chemoprevention among women with AH or LCIS. We have cluster randomized 26 sites across the U.S. through the SWOG Cancer Research Network. A total of 415 patients and 200 healthcare providers are being recruited. They are assigned to standard educational materials alone or combined with the web -based decision support tools. Patient -reported and clinical outcomes are assessed at baseline, after a follow-up visit at 6 months, and yearly for 5 years. The primary outcome is chemoprevention informed choice after the follow-up visit. Secondary endpoints include other patient -reported outcomes, such as chemoprevention knowledge, decision conflict and regret, and self -reported chemoprevention usage. Barriers and facilitators to implementing decision support into clinic workflow are assessed through patient and provider interviews at baseline and mid -implementation. Results/discussion: With this hybrid effectiveness/implementation study, we seek to evaluate if a multi -level intervention effectively promotes informed decision -making about chemoprevention and provide valuable insights on how the intervention is implemented in U.S. clinical settings. Trial Registration: NCT04496739
MoreTranslated text
Key words
Breast cancer chemoprevention,Decision support,Cluster randomized trial
求助PDF
上传PDF
View via Publisher
AI Read Science
AI Summary
AI Summary is the key point extracted automatically understanding the full text of the paper, including the background, methods, results, conclusions, icons and other key content, so that you can get the outline of the paper at a glance.
Example
Background
Key content
Introduction
Methods
Results
Related work
Fund
Key content
- Pretraining has recently greatly promoted the development of natural language processing (NLP)
- We show that M6 outperforms the baselines in multimodal downstream tasks, and the large M6 with 10 parameters can reach a better performance
- We propose a method called M6 that is able to process information of multiple modalities and perform both single-modal and cross-modal understanding and generation
- The model is scaled to large model with 10 billion parameters with sophisticated deployment, and the 10 -parameter M6-large is the largest pretrained model in Chinese
- Experimental results show that our proposed M6 outperforms the baseline in a number of downstream tasks concerning both single modality and multiple modalities We will continue the pretraining of extremely large models by increasing data to explore the limit of its performance
Upload PDF to Generate Summary
Must-Reading Tree
Example
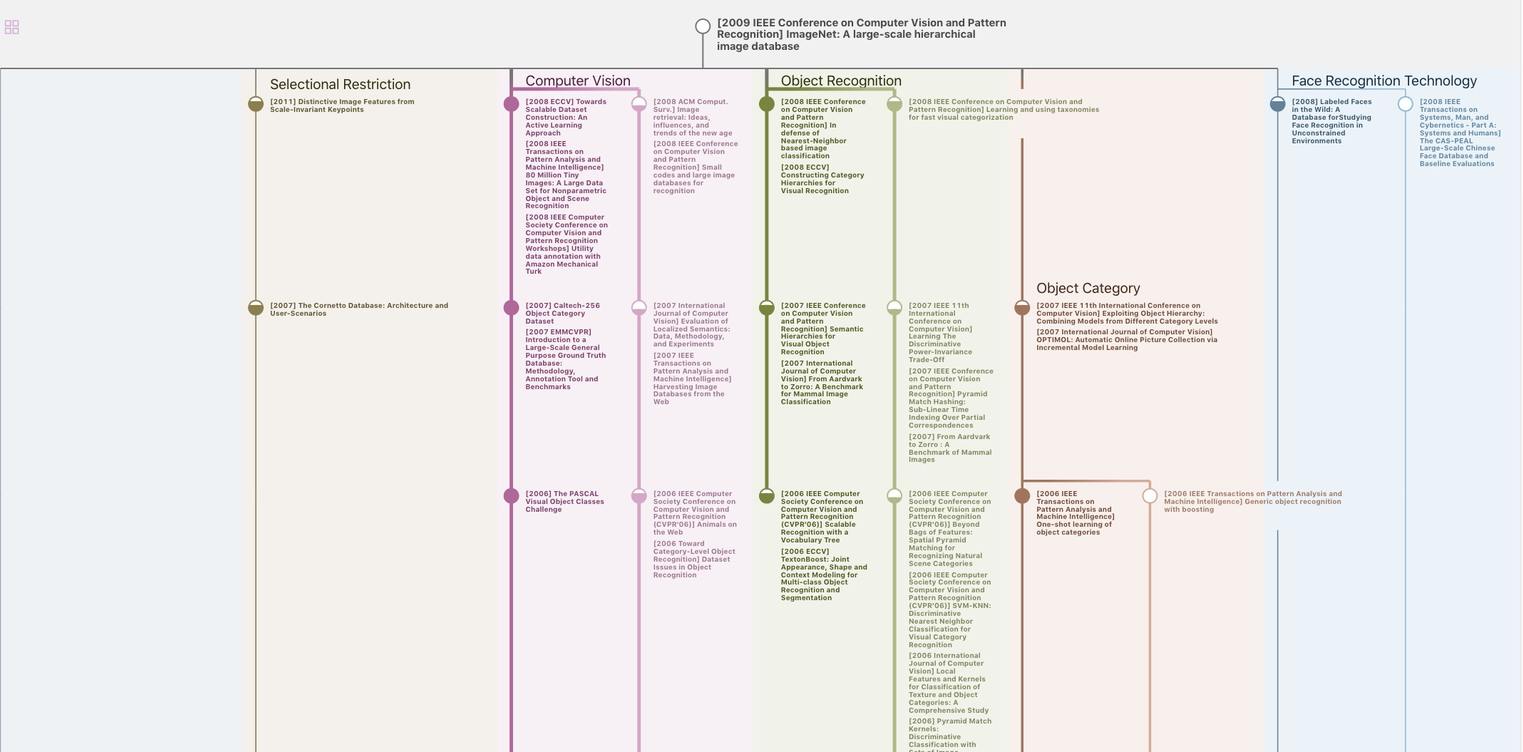
Generate MRT to find the research sequence of this paper
Data Disclaimer
The page data are from open Internet sources, cooperative publishers and automatic analysis results through AI technology. We do not make any commitments and guarantees for the validity, accuracy, correctness, reliability, completeness and timeliness of the page data. If you have any questions, please contact us by email: report@aminer.cn
Chat Paper
GPU is busy, summary generation fails
Rerequest