Language Fairness in Multilingual Information Retrieval
SIGIR 2024(2024)
摘要
Multilingual information retrieval (MLIR) considers the problem of rankingdocuments in several languages for a query expressed in a language that maydiffer from any of those languages. Recent work has observed that approachessuch as combining ranked lists representing a single document language each orusing multilingual pretrained language models demonstrate a preference for onelanguage over others. This results in systematic unfair treatment of documentsin different languages. This work proposes a language fairness metric toevaluate whether documents across different languages are fairly ranked throughstatistical equivalence testing using the Kruskal-Wallis test. In contrast tomost prior work in group fairness, we do not consider any language to be anunprotected group. Thus our proposed measure, PEER (Probability ofEqualExpected Rank), is the first fairness metric specifically designed tocapture the language fairness of MLIR systems. We demonstrate the behavior ofPEER on artificial ranked lists. We also evaluate real MLIR systems on twopublicly available benchmarks and show that the PEER scores align with prioranalytical findings on MLIR fairness. Our implementation is compatible withir-measures and is available at http://github.com/hltcoe/peer_measure.
更多查看译文
AI 理解论文
溯源树
样例
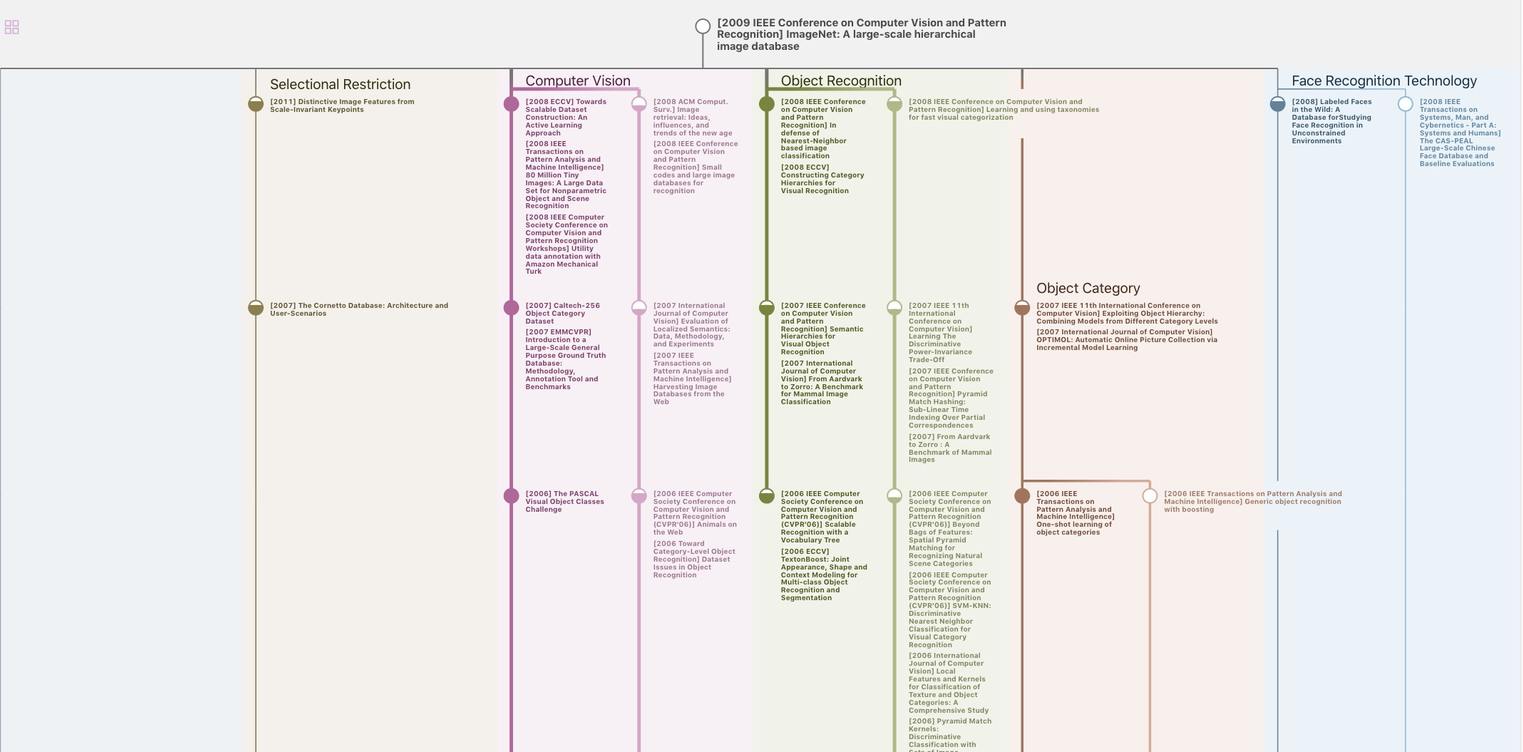
生成溯源树,研究论文发展脉络
Chat Paper
正在生成论文摘要