Water Pipeline Leakage Detection Based on Coherent φ-OTDR and Deep Learning Technology
Applied Sciences(2024)
摘要
Leakage in water supply pipelines remains a significant challenge. It leads to resource and economic waste. Researchers have developed several leak detection methods, including the use of embedded sensors and pressure prediction. The former approach involves pre-installing detectors inside pipelines to detect leaks. This method allows for the precise localization of leak points. The stability is compromised because of the wireless signal strength. The latter approach, which relies on pressure measurements to predict leak events, does not achieve precise leak point localization. To address these challenges, in this paper, a coherent optical time-domain reflectometry (φ-OTDR) system is employed to capture vibration signal phase information. Subsequently, two pre-trained neural network models based on CNN and Resnet18 are responsible for processing this information to accurately identify vibration events. In an experimental setup simulating water pipelines, phase information from both leaking and non-leaking pipe segments is collected. Using this dataset, classical CNN and ResNet18 models are trained, achieving accuracy rates of 99.7% and 99.5%, respectively. The multi-leakage point experiment results indicate that the Resnet18 model has better generalization compared to the CNN model. The proposed solution enables long-distance water-pipeline precise leak point localization and accurate vibration event identification.
更多查看译文
关键词
leakage detection,φ-OTDR,deep leaning,Mels spectrograms
AI 理解论文
溯源树
样例
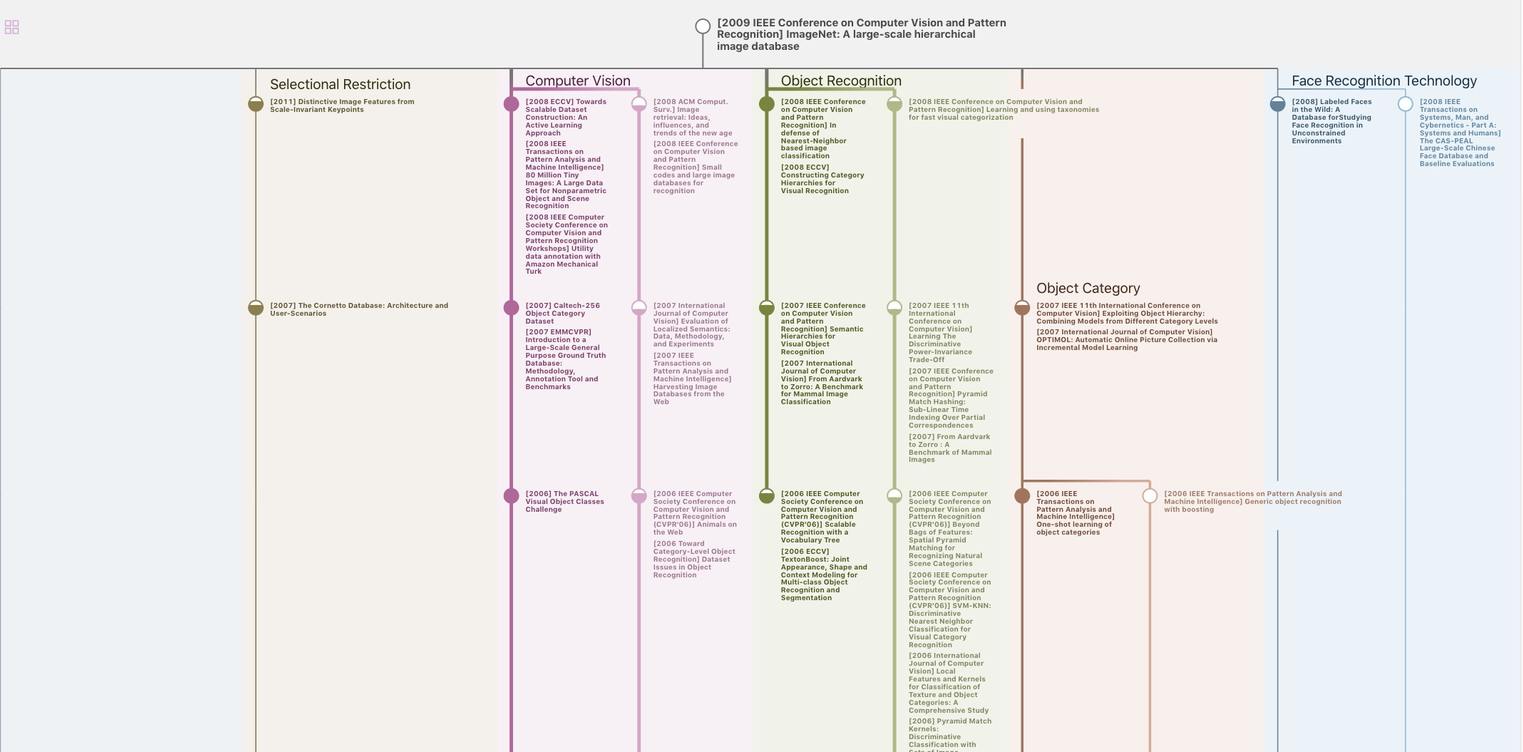
生成溯源树,研究论文发展脉络
Chat Paper
正在生成论文摘要