Low Complexity Deep Learning Method for Base Graph of Protograph LDPC Decoding
2023 9th International Conference on Computer and Communications (ICCC)(2023)
摘要
Deep learning-based decoding algorithms have emerged as a focal point currently in contemporary research. Neural Offset Min-Sum Belief Propagation decoding algorithm is one of the traditional deep learning methods rooted in model-driven approaches. However, with the gradual increase in codeword length, there is an inevitable surge in the training cost and time complexity of such kind of deep learning algorithms. Addressing this issue, a novel deep learning algorithm based on the structural characteristics of the base graph in protograph LDPC codes is proposed. By strategically training the base graph and introducing a unique bit decoding allocation strategy, we endeavor to harmonize the Deep Learning algorithm with the intrinsic structure of the base graph itself. This approach contrasts favorably with several existing methods that utilize conventional segmental deep learning decoding algorithms for protograph LDPC codes. Simulation results demonstrate that, compared to other model-driven based deep learning algorithms, the proposed algorithm significantly reduces the number of parameters required for storage and training, while the loss in decoding performance remains within acceptable bounds.
更多查看译文
关键词
model-driven neural network,Protograph LDPC Code,Base graph,Error-correction Performance
AI 理解论文
溯源树
样例
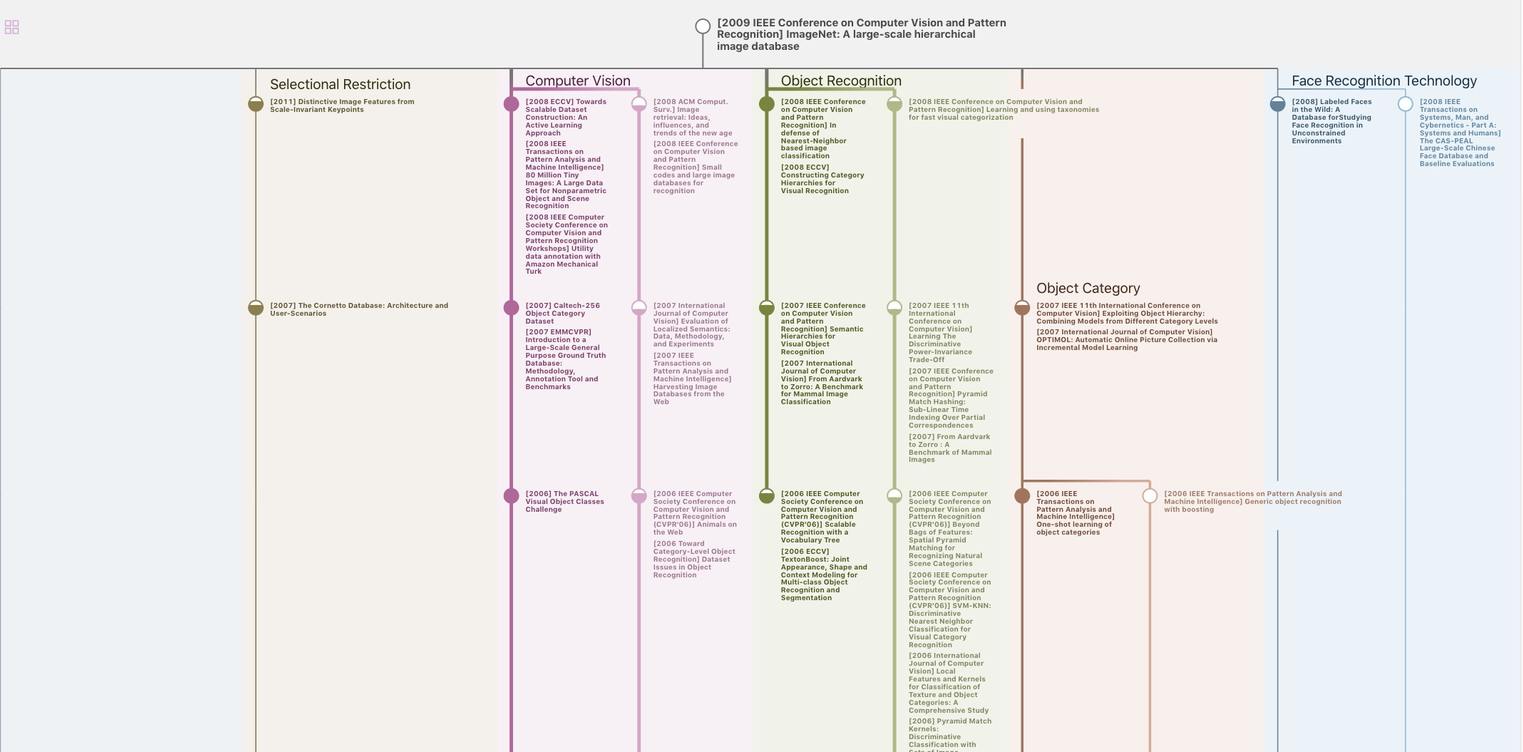
生成溯源树,研究论文发展脉络
Chat Paper
正在生成论文摘要