FewarNet: An Efficient Few-Shot View Synthesis Network Based on Trend Regularization
IEEE Transactions on Circuits and Systems for Video Technology(2024)
摘要
Novel view synthesis from existing inputs remains a research focus in computer vision. Predicting views becomes more challenging when only a limited number of views are available. This challenge is commonly referred to as the few-shot view synthesis problem. Recently, various strategies have emerged for few-shot view synthesis, such as transfer learning, depth supervision, and regularization constraints. However, transfer learning relies on massive scene data, depth supervision is affected by input depth quality, and regularization causes increased computational costs or impaired generalization. To address these issues, we propose a new few-shot view synthesis framework called FewarNet that introduces trend regularization to leverage depth structural features and a warping loss to supervise depth estimation, possessing the advantages of existing few-shot strategies, enabling high-quality novel view prediction with generalization and efficiency. Specifically, FewarNet consists of three stages: fusion, warping, and rectification. In the fusion stage, a fusion network is introduced to estimate depths using scene priors from coarse depths. In the warping stage, the predicted depths are used to guide the warping of the input views, and a distance-weighted warping loss is proposed to correctly guide depth estimation. To further improve prediction accuracy, we propose trend regularization which imposes penalties on depth variation trends to provide depth structural constraints. In the rectification stage, a rectification network is introduced to refine occluded regions in each warped view to generate novel views. Additionally, a rapid view synthesis strategy that leverages depth interpolation is designed to improve efficiency. We validate the method’s effectiveness and generalization on various datasets. Given the same sparse inputs, our method demonstrates superior performance in quality and efficiency over state-of-the-art few-shot view synthesis methods.
更多查看译文
关键词
Depth estimation,few-shot view synthesis,regularization constraint,prior depth
AI 理解论文
溯源树
样例
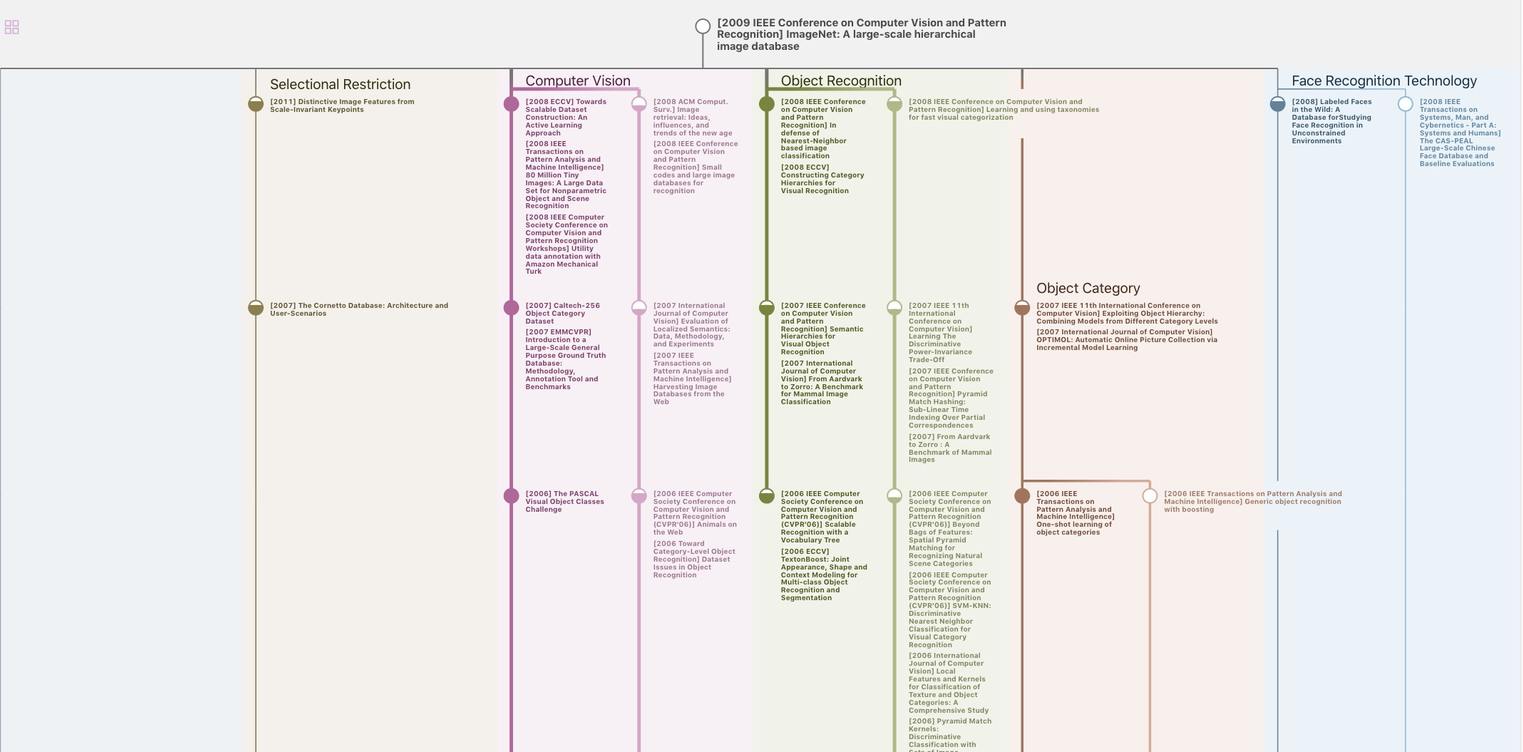
生成溯源树,研究论文发展脉络
Chat Paper
正在生成论文摘要