A lightweight dynamic dual-damped wavelet-based convolutional neural network for interpretable bearing fault diagnosis
Measurement Science and Technology(2024)
摘要
Abstract Wavelet-based convolutional neural networks (CNNS) have attracted widespread attention because they can improve the interpretability of intelligent fault diagnosis methods. However, the fault feature representation capability of typical wavelet-based convolution kernel frameworks must be strengthened to improve the diagnostic accuracy of complex faults. In the meantime, the large number of network parameters leads to high computational costs. To address these issues, a lightweight wavelet-based dynamic CNN, which comprises a dual-damping wavelet-based dynamic CNN (DWDC) block and a discrete wavelet transformation enhancement (DWTE) block, is put forward. In the DWDC block, a wavelet convolution layer is initially designed, where a dual-damping wavelet is used as the kernel function to improve the match of the convolution kernel with fault impulses. Subsequently, a dynamic convolution layer with multiple parallel small-size convolutional kernels is designed to screen the fault features instead of a multilayer network structure, thereby greatly reducing the number of network parameters. Finally, the DWTE block is constructed by combining the discrete wavelet transformation (DWT) and residual dense block (RDB), and it can mine more fault information from the previously extracted features. The experiments on the variable speed bearing dataset, locomotive bearing dataset with constant speed and the Case Western Reserve University (CWRU) dataset prove that the proposed approach outperforms five classical CNN models and six advanced wavelet-based CNN models. In addition, it can effectively solve the issue of data imbalance because of its powerful feature extraction capability.
更多查看译文
AI 理解论文
溯源树
样例
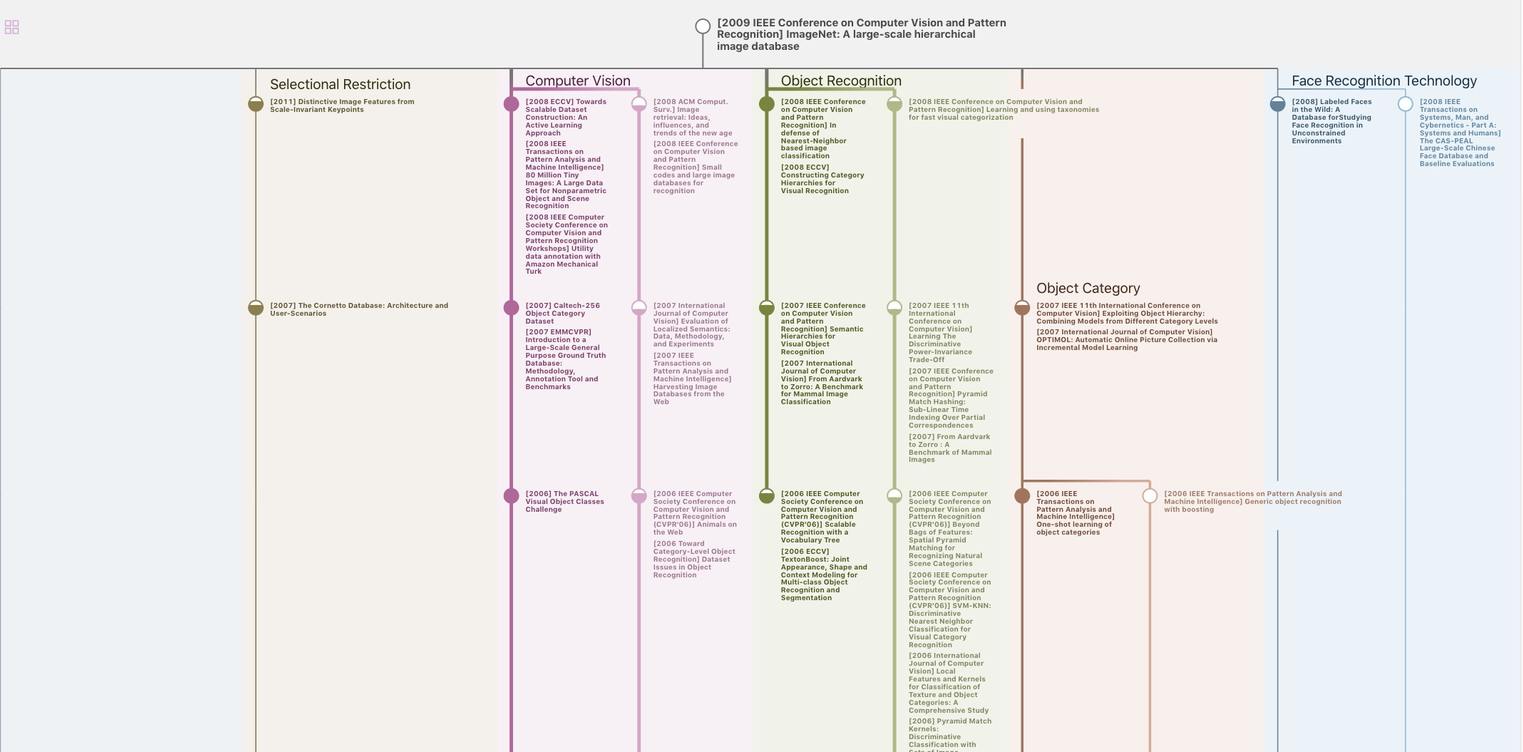
生成溯源树,研究论文发展脉络
Chat Paper
正在生成论文摘要