Spatial Heterodyne Raman Spectral Baseline Correction Based on Improved Adaptive Iterative Re-Weighted Penalized Least Square Method
ACTA OPTICA SINICA(2024)
摘要
Objective Raman spectroscopy is a non-destructive analytical technique based on the inelastic scattering of light by matter.While inducing Raman signals,the fluorescence background directly affects the characterization of sample Raman properties.The common approaches to reducing fluorescence background are primarily implemented through hardware and software methods.Hardware methods mainly involve techniques such as shifted excitation Raman difference spectroscopy,time-resolved Raman spectroscopy,and deep ultraviolet Raman spectroscopy.Although these methods exhibit effective outcomes,they often entail complex instrument setups and high costs.Software methods,on the other hand,refer to utilizing signal processing techniques to subtract fluorescence background from Raman spectra.Raman spectra are characterized by typically discontinuous with sharp peaks,contrasted with the continuous and smooth trends often present in fluorescence spectra.Given the difference in their spectral characteristics,when Raman spectroscopy analysis is carried out,employing baseline correction algorithms to eliminate fluorescence interference helps ensure the reliability and accuracy of Raman spectroscopy data.Common methods for mitigating fluorescence background include polynomial fitting,discrete wavelet transform,morphological algorithms,variational mode decomposition,least squares methods,and neural networks.However,due to the presence of Raman peaks,these methods typically result in varying degrees of baseline rise in the fitting outcomes.In the present study,we report an adaptive iterative re-weighted penalized least square(airPLS)method based on Raman peaks truncation.By identifying the positions of Raman peaks,truncating the corresponding regions,and employing the airPLS algorithm for baseline fitting,the method reduces the rise in the fitted baseline caused by abrupt changes in intensity within the Raman peak regions,making the fitted baseline approach closer to the true baseline.We hope that this improved method will further enhance the accuracy of Raman spectroscopy baseline fitting. Methods Baseline fitting is conducted with airPLS based on Raman peaks truncation.Initially,the spectral signal is denoised by the Savitzky-Golay filter.Subsequently,we employ a peak-finding algorithm to identify Raman peaks within the denoised spectrum and use the first derivative of the spectrum to determine the left and right boundaries for each Raman peak.Following this,we truncate the Raman peaks within these defined boundaries to obtain the original baseline.An airPLS fitting is performed on this original baseline to derive a new baseline.At this stage,we compute the difference between the new baseline and the original baseline and truncate the regions where the absolute difference exceeds a threshold.We iterate this process by subjecting the truncated signal to successive airPLS fitting until the absolute difference between the baselines from two consecutive fittings is less than the threshold,concluding the iteration.The resulting fitted baseline is output.Here,the threshold represents the average of the absolute differences between the baselines from two consecutive fittings.Subtracting the fitted baseline from the original Raman spectrum yields the corrected Raman spectrum. Results and Discussions The airPLS based on Raman peaks truncation has demonstrated outstanding performance in baseline fitting.Simulated Raman spectra and measured Raman spectra from lipstick are utilized to validate the proposed baseline fitting method,respectively.Comparative analyses are conducted against commonly used baseline fitting methods,including polynomial fitting,discrete wavelet transform,variational mode decomposition,and airPLS.As depicted in Figs.6(a),7(a),and 7(b),although the algorithm proposed in this article achieves a baseline fit closer to the theoretical baseline in regions with weak Raman peaks or without Raman peaks,the improvement compared to the aforementioned algorithms is not notably conspicuous.This indicates that the fitting capabilities of these algorithms are similar in spectra exhibiting gradual trends.However,near the Raman peak regions,as shown in Figs.6(b),6(c),and 7(c),these methods experience baseline elevation due to abrupt changes in spectral peak intensity.In contrast,the proposed baseline fitting method,which incorporates Raman peaks truncation,minimizes the influence of Raman peaks on the fitting results,resulting in the closest fit to the theoretical values.Table 3 presents the root mean square errors(RMSE)between the fitted baseline and the theoretical baseline for our method and the aforementioned commonly used methods,evaluating the performance of these methods in spectra exhibiting both single and complex trends.Comparative analysis indicates that under various signal-to-noise ratios of spectra,our method yields the lowest RMSE,showcasing its superior performance.The baseline fitting results of the lipstick Raman spectrum shown in Fig.8 are consistent with the simulated analysis outcomes.In the region devoid of Raman peaks(800-1000 cm-1),the baseline fitting capabilities of each algorithm are similar.However,within the region with numerous Raman peaks(1100 to 1650 cm-1),the baseline fits of all algorithms are affected to varying degrees by the spectral peaks,resulting in baseline rises.Our baseline fitting method,employing spectral peak truncation and an iterative approach,significantly mitigates the influence of spectral peaks on the fitting outcomes.This method maximizes the preservation of Raman peak intensities and stands out as the optimal choice among the various methods evaluated. Conclusions We introduce the Raman peak-truncated airPLS baseline fitting method.The utilization of Raman peak truncation mitigates the influence of abrupt changes in Raman peak intensity on baseline fitting.The method not only inherits the effective baseline fitting performance of airPLS in peak-free regions but also resolves the issue of baseline elevation caused by Raman peak intensity,thereby enhancing the accuracy of baseline fitting.Comparative experiments conducted on simulated spectra demonstrate the superior baseline fitting performance of Raman peak-truncated airPLS.Under different spectral signal-to-noise ratios,the RMSE for Type 1 spectra fitted by our method is below 0.0042,and for Type 2 spectra,it is below 0.0052,which is the lowest among various methods.In experiments fitting Raman spectra from lipstick samples,airPLS based on Raman peaks truncation outperforms several commonly used algorithms.It accurately restores Raman peak intensities without distorting the corrected spectra,effectively removing fluorescence baseline trends and meeting the requirements of Raman spectroscopy data processing.
更多查看译文
关键词
Raman spectroscopy,spatial heterodyne,baseline correction,least squares method
AI 理解论文
溯源树
样例
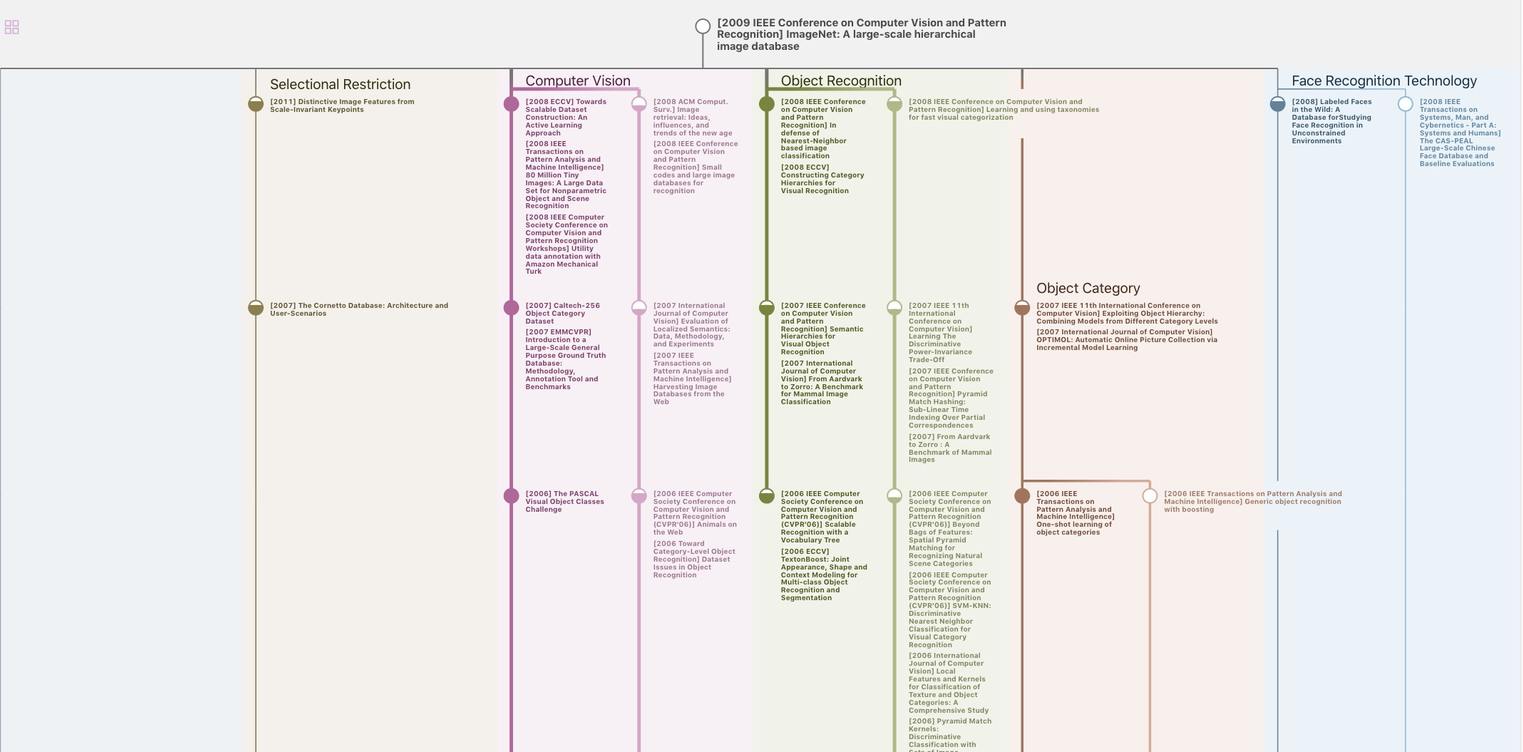
生成溯源树,研究论文发展脉络
Chat Paper
正在生成论文摘要