Electrochemical Degradation of Diclofenac Generates Unexpected Thyroidogenic Transformation Products: Implications for Environmental Risk Assessment
JOURNAL OF HAZARDOUS MATERIALS(2024)
关键词
Ecotoxicity,Endocrine disruption,Electrochemical advanced oxidation processes,UHPLC-QTOF-MS,Xenopus laevis
AI 理解论文
溯源树
样例
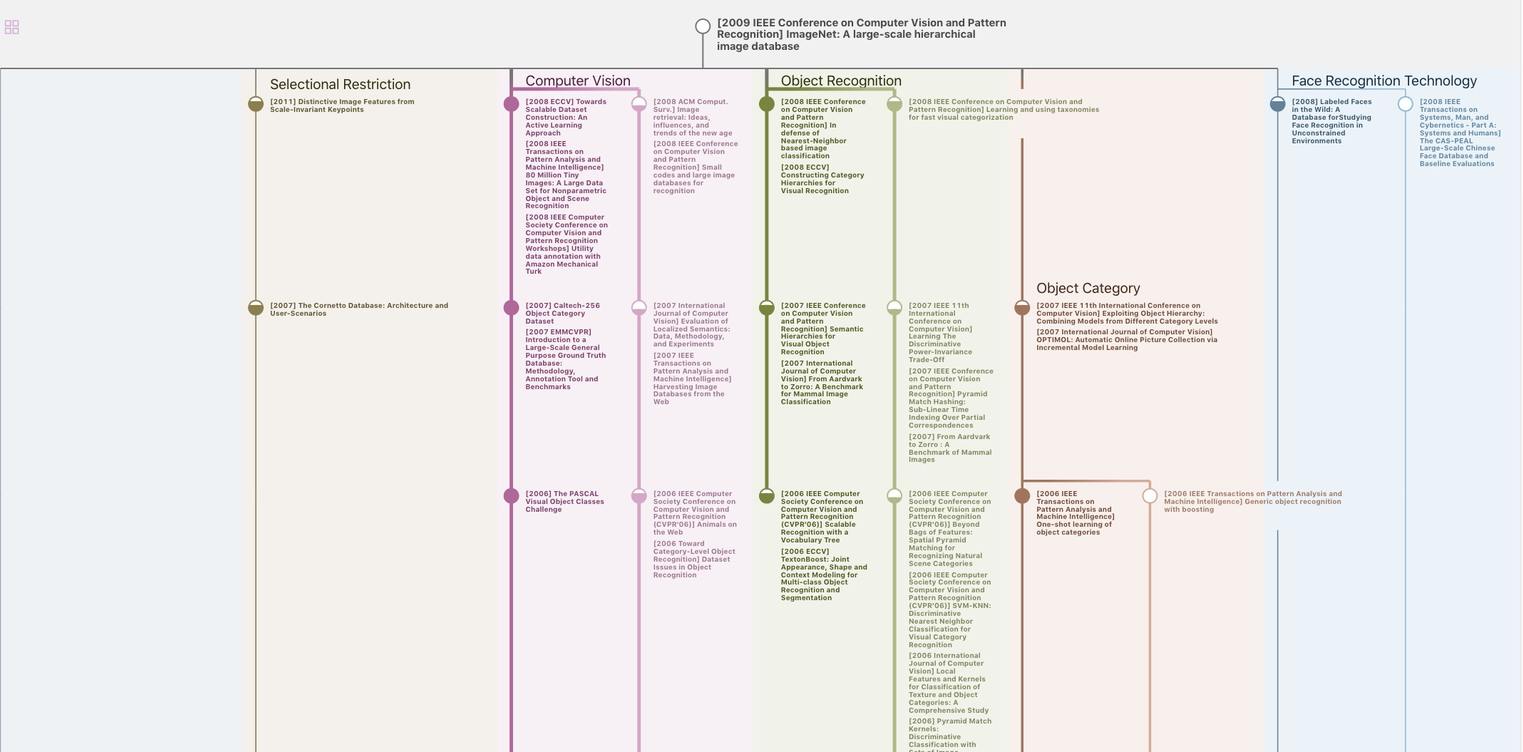
生成溯源树,研究论文发展脉络
Chat Paper
正在生成论文摘要