Neighborhood convolutional graph neural network
Knowledge-Based Systems(2024)
摘要
Graph convolutional networks (GCNs) have emerged as powerful tools for learning representations of graph structured data. A recent advancement is a decoupled GCN, which separates neighborhood aggregation from feature transformation within each graph convolutional layer and yields promising results. However, our investigation on node-level operations reveals its limitations in terms of modeling capacity and scalability, which hinder effective learning of rich node representations of diverse graphs. To address these challenges, we introduce a new paradigm of decoupled GCNs, called a neighborhood convolutional graph neural network (NCGNN). The NCGNN uses a novel neighborhood aggregator to generate a feature matrix of multi-hop neighborhoods of each node before model training. This avoids training the model via the adjacency matrix, effectively controls the training cost, and enhances scalability. Additionally, the NCGNN integrates advanced feature learning components, including a dedicated convolutional neural network for rich neighborhood information extraction and a feature fusion module to seamlessly integrate the raw attributes with the extracted features. Extensive experiments validate the superiority of the NCGNN over representative graph neural networks in node classification tasks, demonstrating its effectiveness and scalability for diverse graph structures.
更多查看译文
关键词
Graph convolutional network,Graph representation learning,Convolutional neural network,Multi-hop neighborhood,Neighborhood information
AI 理解论文
溯源树
样例
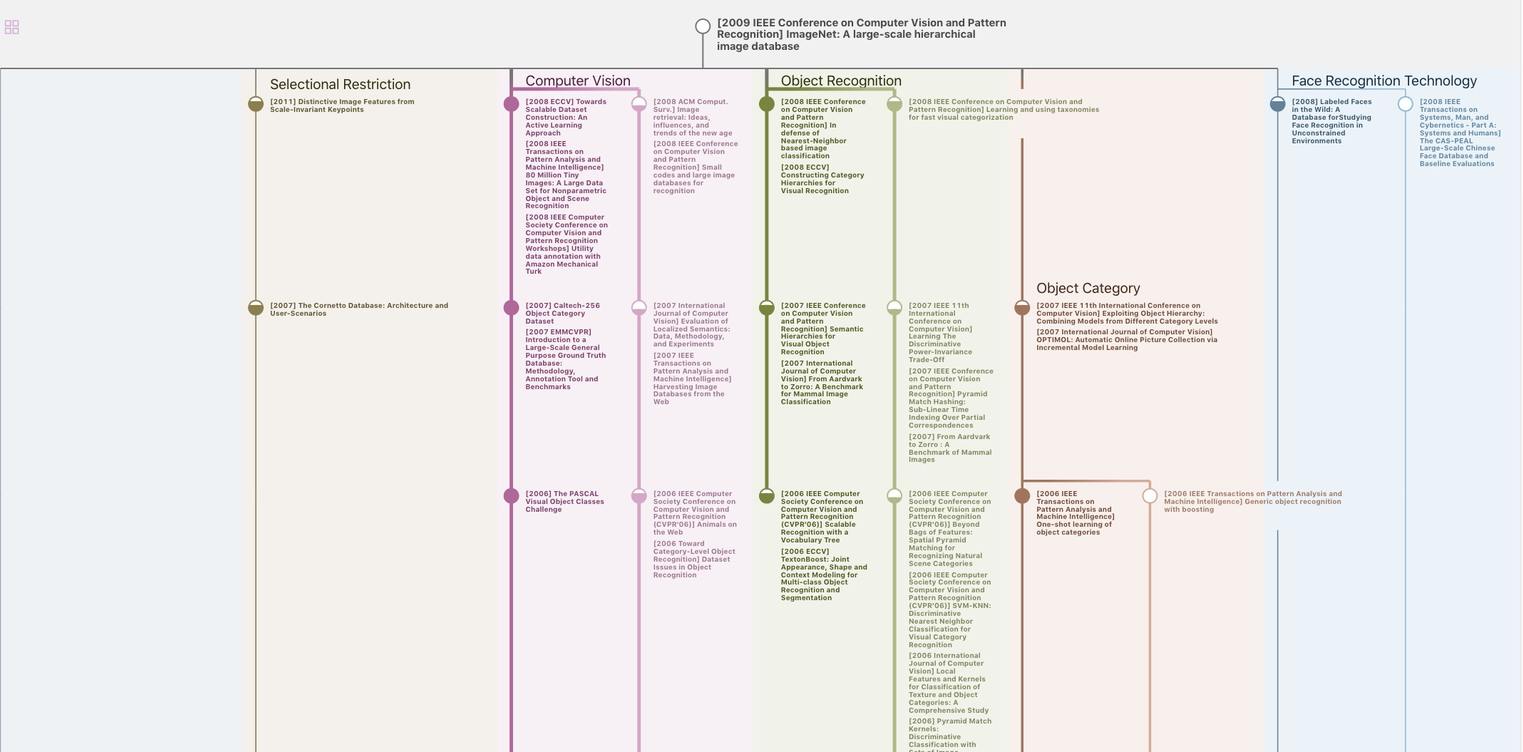
生成溯源树,研究论文发展脉络
Chat Paper
正在生成论文摘要