Learning Mixtures of Gaussians Using Diffusion Models
CoRR(2024)
Abstract
We give a new algorithm for learning mixtures of k Gaussians (with identity
covariance in ℝ^n) to TV error ε, with quasi-polynomial
(O(n^poly log(n+k/ε))) time and sample
complexity, under a minimum weight assumption. Unlike previous approaches, most
of which are algebraic in nature, our approach is analytic and relies on the
framework of diffusion models. Diffusion models are a modern paradigm for
generative modeling, which typically rely on learning the score function
(gradient log-pdf) along a process transforming a pure noise distribution, in
our case a Gaussian, to the data distribution. Despite their dazzling
performance in tasks such as image generation, there are few end-to-end
theoretical guarantees that they can efficiently learn nontrivial families of
distributions; we give some of the first such guarantees. We proceed by
deriving higher-order Gaussian noise sensitivity bounds for the score functions
for a Gaussian mixture to show that that they can be inductively learned using
piecewise polynomial regression (up to poly-logarithmic degree), and combine
this with known convergence results for diffusion models. Our results extend to
continuous mixtures of Gaussians where the mixing distribution is supported on
a union of k balls of constant radius. In particular, this applies to the
case of Gaussian convolutions of distributions on low-dimensional manifolds, or
more generally sets with small covering number.
MoreTranslated text
PDF
View via Publisher
AI Read Science
AI Summary
AI Summary is the key point extracted automatically understanding the full text of the paper, including the background, methods, results, conclusions, icons and other key content, so that you can get the outline of the paper at a glance.
Example
Background
Key content
Introduction
Methods
Results
Related work
Fund
Key content
- Pretraining has recently greatly promoted the development of natural language processing (NLP)
- We show that M6 outperforms the baselines in multimodal downstream tasks, and the large M6 with 10 parameters can reach a better performance
- We propose a method called M6 that is able to process information of multiple modalities and perform both single-modal and cross-modal understanding and generation
- The model is scaled to large model with 10 billion parameters with sophisticated deployment, and the 10 -parameter M6-large is the largest pretrained model in Chinese
- Experimental results show that our proposed M6 outperforms the baseline in a number of downstream tasks concerning both single modality and multiple modalities We will continue the pretraining of extremely large models by increasing data to explore the limit of its performance
Try using models to generate summary,it takes about 60s
Must-Reading Tree
Example
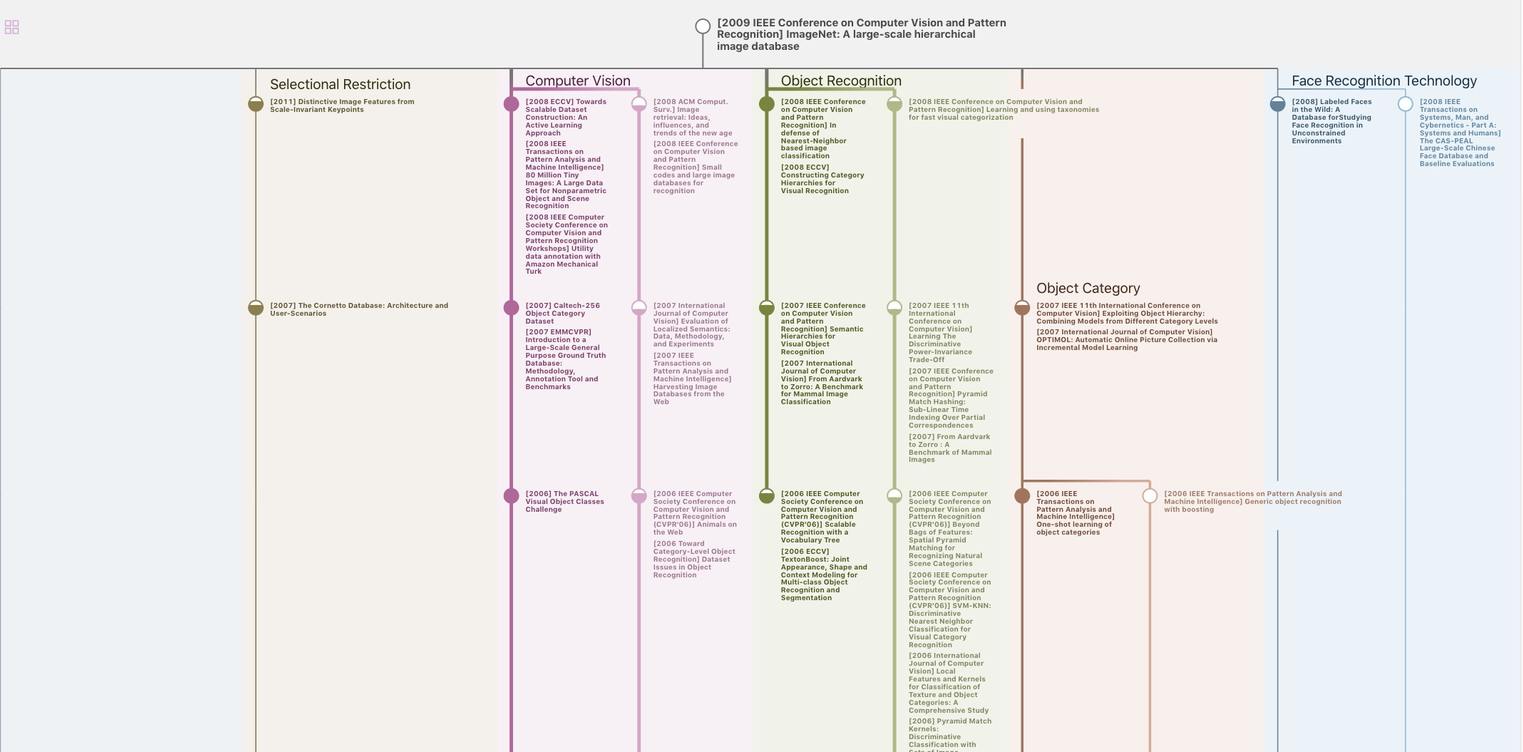
Generate MRT to find the research sequence of this paper
Related Papers
CoRR 2024
被引用0
Data Disclaimer
The page data are from open Internet sources, cooperative publishers and automatic analysis results through AI technology. We do not make any commitments and guarantees for the validity, accuracy, correctness, reliability, completeness and timeliness of the page data. If you have any questions, please contact us by email: report@aminer.cn
Chat Paper
去 AI 文献库 对话