Bridging data barriers among participants: Assessing the potential of geoenergy through federated learning
Applied Energy(2024)
摘要
Machine learning algorithm emerges as a promising approach in energy fields, but its practicality is hindered by data barriers, stemming from high collection costs and privacy concerns. This study introduces a novel federated learning (FL) framework based on XGBoost models, enabling safe collaborative modeling with accessible yet concealed data from multiple parties. Hyperparameter tuning of the models is achieved through Bayesian Optimization. To ascertain the merits of the proposed FL-XGBoost method, a comparative analysis is conducted between separate and centralized models to address a classical binary classification problem in the geoenergy sector. The results reveal that the proposed FL framework strikes an optimal balance between privacy and accuracy. FL models demonstrate superior accuracy and generalization capabilities compared to separate models, particularly for participants with limited data or low correlation features, and offer significant privacy benefits compared to centralized models. The aggregated optimization approach within the FL agreement proves effective in tuning hyperparameters. This study opens new avenues for assessing unconventional reservoirs through collaborative and privacy-preserving FL techniques.
更多查看译文
关键词
Data privacy and security,Federated learning,Geoenergy,Bayesian optimization,Production estimation
AI 理解论文
溯源树
样例
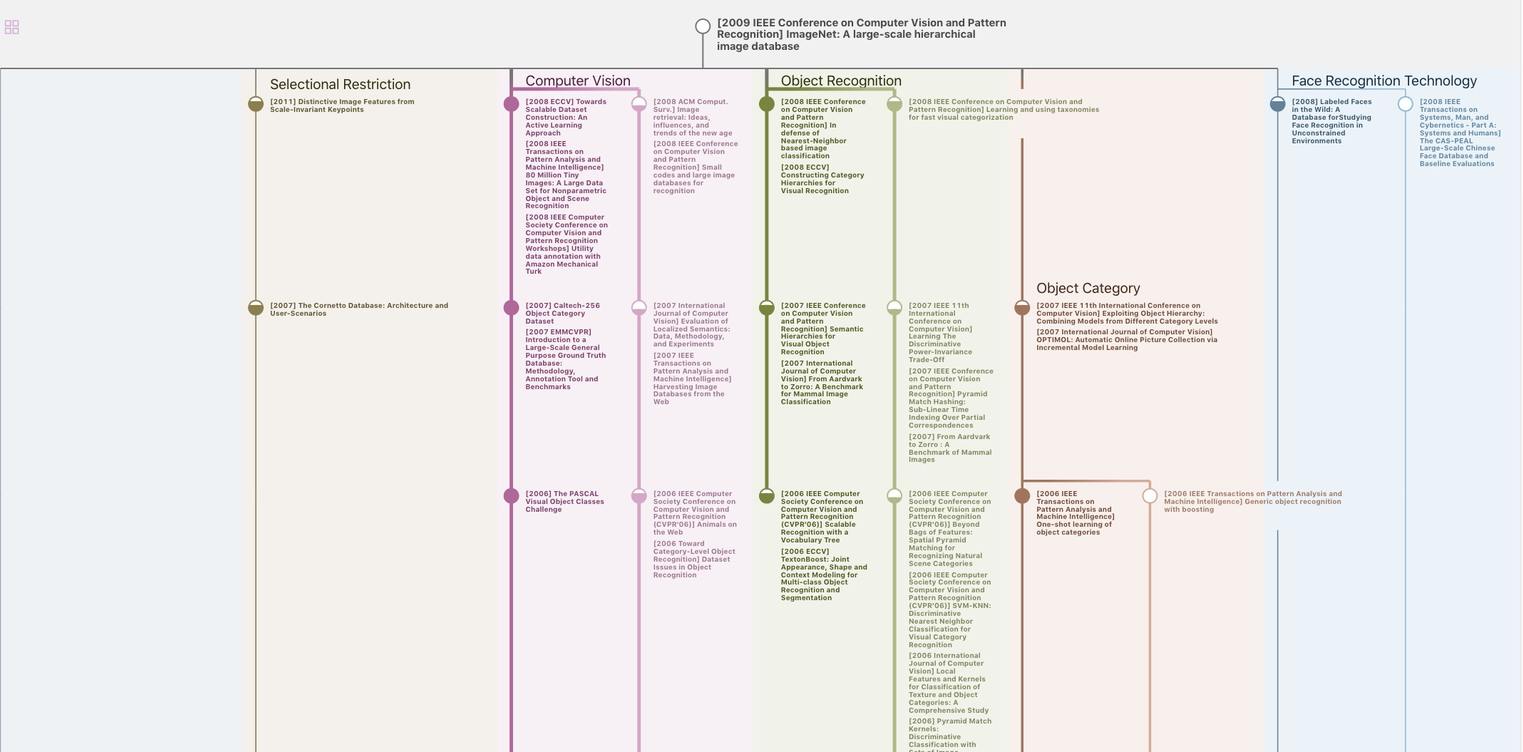
生成溯源树,研究论文发展脉络
Chat Paper
正在生成论文摘要