An Intelligent Bayesian Inference Based Learning Automaton Approach for Traffic Management in Radio Access Network
Wireless Personal Communications(2024)
摘要
Wireless cellular networks can be used to establish machine-to-machine (M2M) connections, enabling the use of the Internet of Things (IoT). Access class barring (ACB) is one of the most efficient strategies for directly regulating M2M traffic. However, traditional ACB techniques use a set factor to manage the traffic flow of machine-type communication devices (MTCD). This research proposes a unique intelligent traffic management system that uses Bayesian inference-based learning automatons (BI-LA) to estimate M2M traffic by dynamically changing the ACB factor. The BI-LA-based ACB system uses the self-adaptive learning property of learning automata (LA) to adjust the ACB factor for determining M2M traffic. To adjust the ABC factor, the problem can be phrased based on the collision probability, and it is addressed by integrating Bayesian inference (BI) into LA to compute the environment’s response to each action. The proposed method is simulated with the network simulator-3 (NS3), and performance measures such as average access delay, average number of access attempts, access success rate, and access success are evaluated. The experiment results reveal that the BI-LA ACB technique achieves nearly theoretically ideal performance when compared to conventional LSTM-based ACB schemes, dynamic ACB approaches, and reinforcement learning (RL)-based ABC approaches. The minimum access delay attained by the proposed BI-LA ACB system is approximately 1876 ms and 27.6 ms, respectively.
更多查看译文
关键词
Access class barring,Bayesian inference,Learning automaton,Barring factor,Machine-to-machine communication,Traffic management
AI 理解论文
溯源树
样例
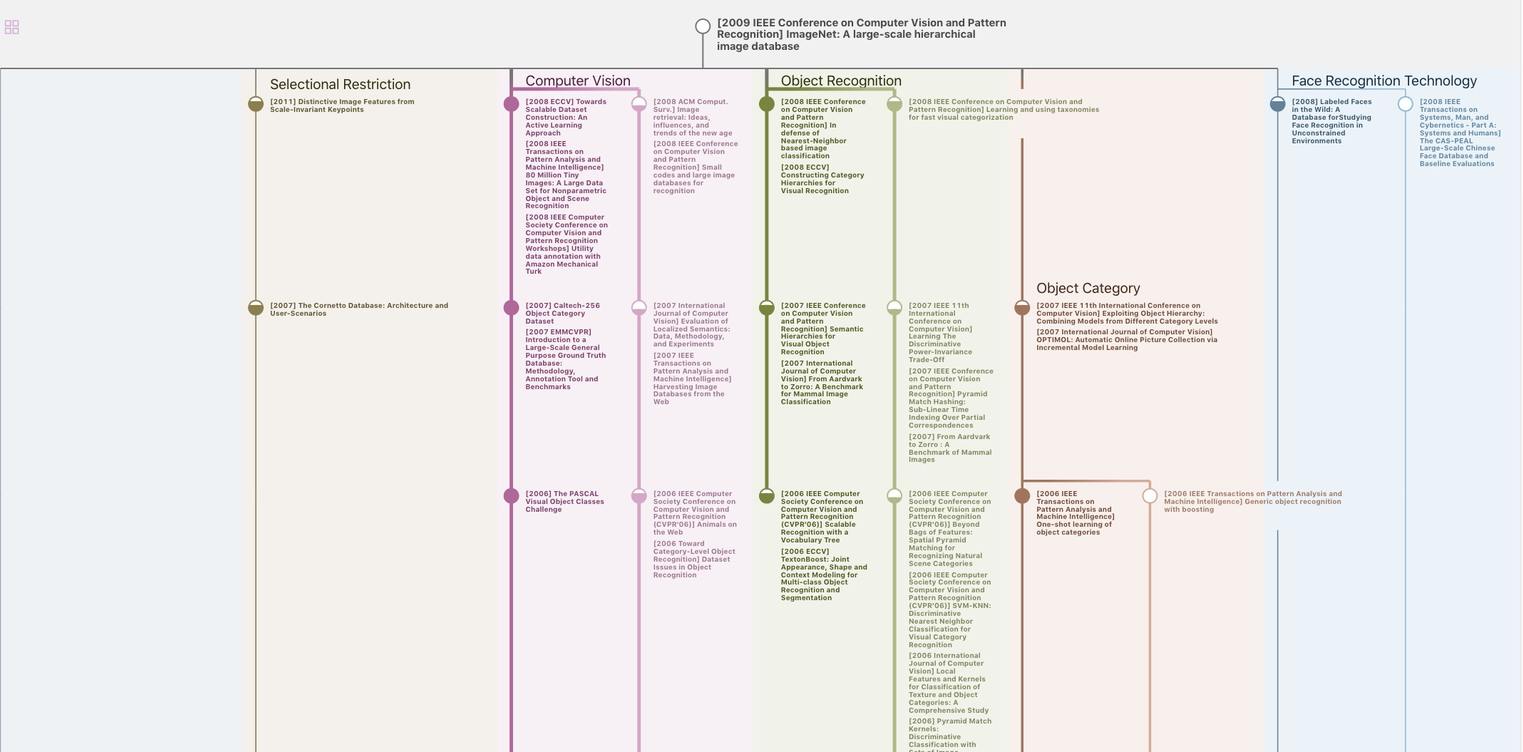
生成溯源树,研究论文发展脉络
Chat Paper
正在生成论文摘要