Adaptive Blind Super-resolution Model Based on Degradation Representation.
International Conferences on Computing and Pattern Recognition(2023)
摘要
Most CNN-based super-resolution methods are normally designed based on the assumption that low-resolution images have a fixed degradation pattern. When the actual degradation differs from its assumption, the performance of these methods will severely degrade. In order to deal with this problem, most of the previous methods adopt the way of predicting the blur kernel to reconstruct the SR image. However, this type of methods needs to accurately predict the blur kernel, where the error propagation caused by the estimation deviation of the blur kernel cannot be avoided. To address these issues, we propose an adaptive blind super-resolution model based on degenerate representation (ABSRM) in this paper. First, the shallow feature extraction module extracts the original blurriness features from the low-resolution (LR) image. Second, the degradation representations generator generates degradation representations to distinguish various degradations in the representation space instead of specific blur kernels. Third, the adaptive reconstruction module flexibly handles various degradations to reconstruct clearer images based on different degradation representations. Extensive experiments show that our ABSRM shows advantages compared with the state-of-the-art blind super-resolution methods.
更多查看译文
AI 理解论文
溯源树
样例
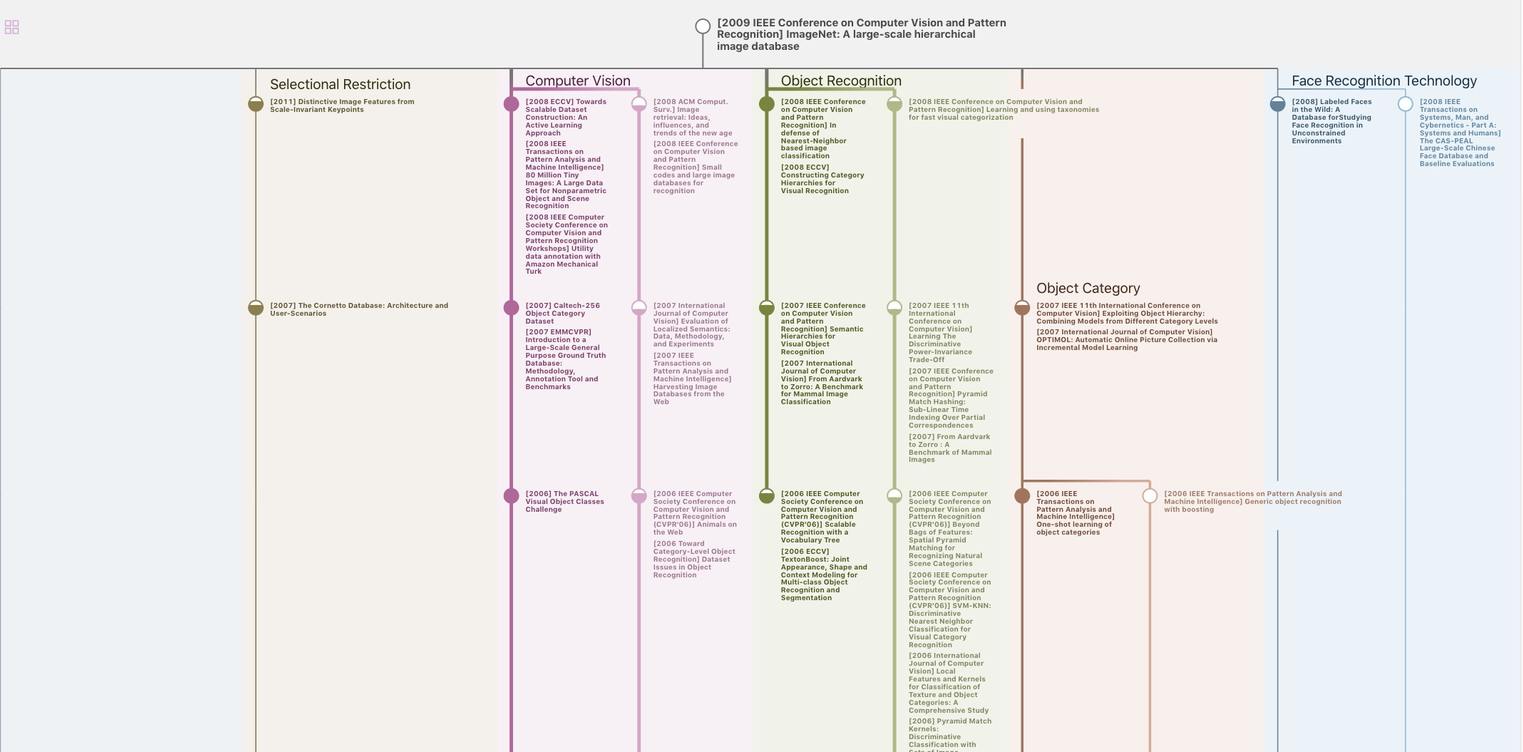
生成溯源树,研究论文发展脉络
Chat Paper
正在生成论文摘要