Change Detection in Hyperspectral Images Based on a Spatial-Spectral-Temporal Attention Network.
ICCPR '23 Proceedings of the 2023 12th International Conference on Computing and Pattern Recognition(2024)
摘要
Most of the existing hyperspectral image change detection methods based on deep learning utilize convolutional neural networks (CNN), recurrent neural networks (RNN), and long-short-term memory (LSTM). However, CNNs primarily focus on extracting spatial information from hyperspectral images, thereby failing to fully utilize channel and temporal information. In this paper, we propose a network called STSTNet that can jointly extract spatial, spectral, and temporal feature information. To achieve a richer feature representation space, we employ a spatial attention module to extract spatial feature information from feature maps, and a spectral transformer encoder structure to extract abundant spectral sequence information. Specifically, we design two temporal information extraction structures after each spatial information extraction structure or spectral information extraction structure to capture correlations and interactions within the hyperspectral images. Extensive experiments have been conducted on three hyperspectral datasets, and the experimental results demonstrate the network’s excellent performance.
更多查看译文
AI 理解论文
溯源树
样例
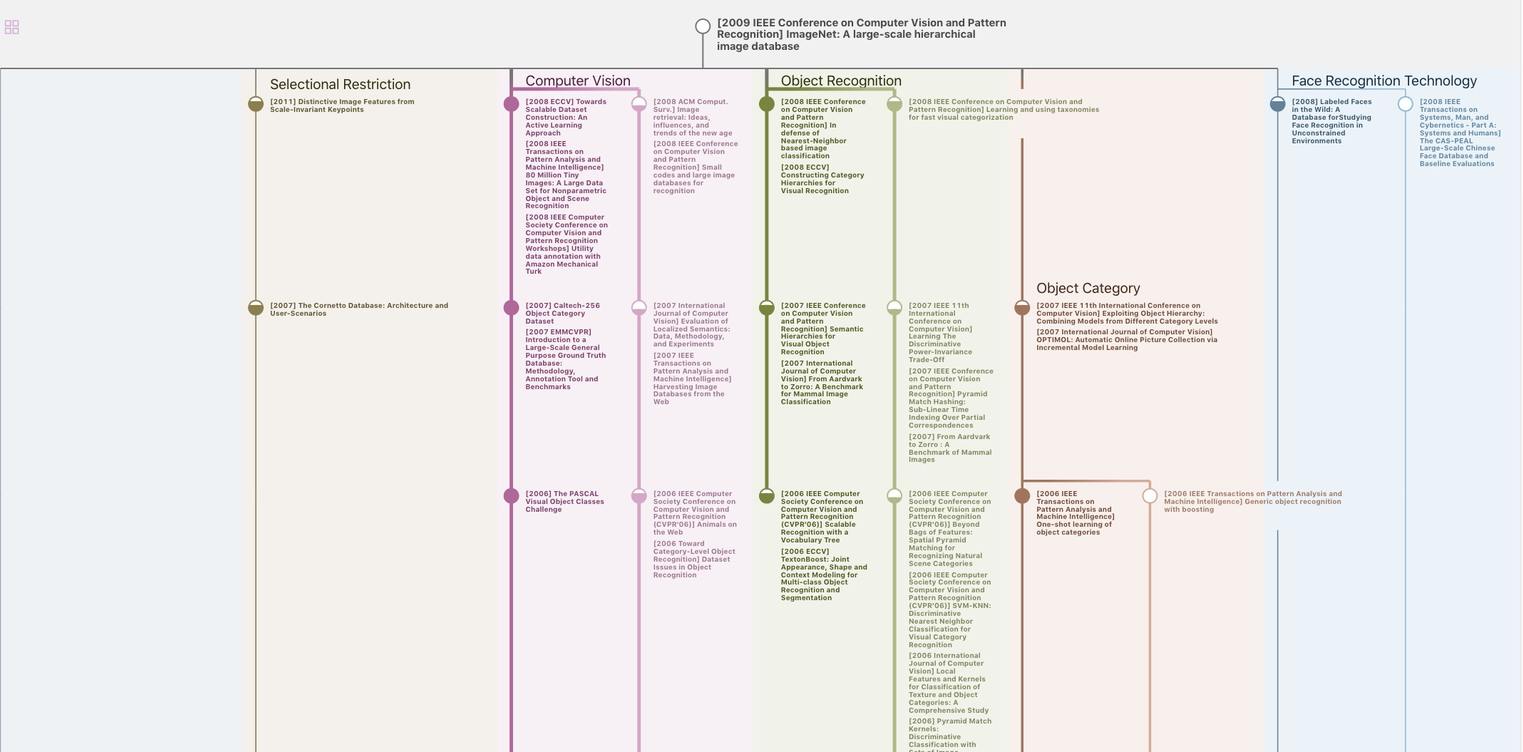
生成溯源树,研究论文发展脉络
Chat Paper
正在生成论文摘要