FTL: Transfer Learning Nonlinear Plasma Dynamic Transitions in Low Dimensional Embeddings via Deep Neural Networks
CoRR(2024)
摘要
Deep learning algorithms provide a new paradigm to study high-dimensional
dynamical behaviors, such as those in fusion plasma systems. Development of
novel model reduction methods, coupled with detection of abnormal modes with
plasma physics, opens a unique opportunity for building efficient models to
identify plasma instabilities for real-time control. Our Fusion Transfer
Learning (FTL) model demonstrates success in reconstructing nonlinear kink mode
structures by learning from a limited amount of nonlinear simulation data. The
knowledge transfer process leverages a pre-trained neural encoder-decoder
network, initially trained on linear simulations, to effectively capture
nonlinear dynamics. The low-dimensional embeddings extract the coherent
structures of interest, while preserving the inherent dynamics of the complex
system. Experimental results highlight FTL's capacity to capture transitional
behaviors and dynamical features in plasma dynamics – a task often challenging
for conventional methods. The model developed in this study is generalizable
and can be extended broadly through transfer learning to address various
magnetohydrodynamics (MHD) modes.
更多查看译文
AI 理解论文
溯源树
样例
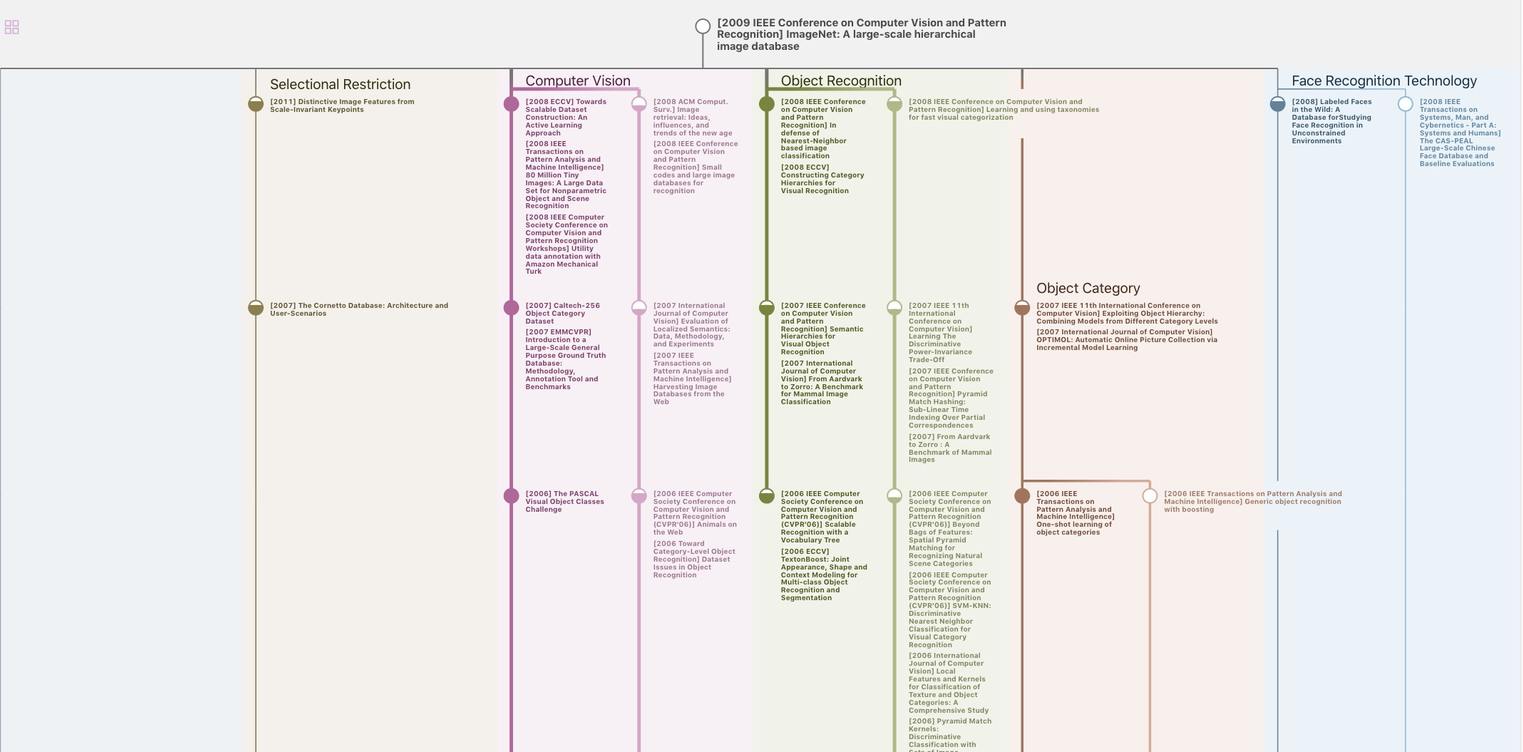
生成溯源树,研究论文发展脉络
Chat Paper
正在生成论文摘要