Cracking black-box models: Revealing hidden machine learning techniques behind their predictions
Intelligent Data Analysis(2024)
摘要
The quest for transparency in black-box models has gained significant momentum in recent years. In particular, discovering the underlying machine learning technique type (or model family) from the performance of a black-box model is a real important problem both for better understanding its behaviour and for developing strategies to attack it by exploiting the weaknesses intrinsic to the learning technique. In this paper, we tackle the challenging task of identifying which kind of machine learning model is behind the predictions when we interact with a black-box model. Our innovative method involves systematically querying a black-box model (oracle) to label an artificially generated dataset, which is then used to train different surrogate models using machine learning techniques from different families (each one trying to partially approximate the oracle’s behaviour). We present two approaches based on similarity measures, one selecting the most similar family and the other using a conveniently constructed meta-model. In both cases, we use both crisp and soft classifiers and their corresponding similarity metrics. By experimentally comparing all these methods, we gain valuable insights into the explanatory and predictive capabilities of our model family concept. This provides a deeper understanding of the black-box models and increases their transparency and interpretability, paving the way for more effective decision making.
更多查看译文
AI 理解论文
溯源树
样例
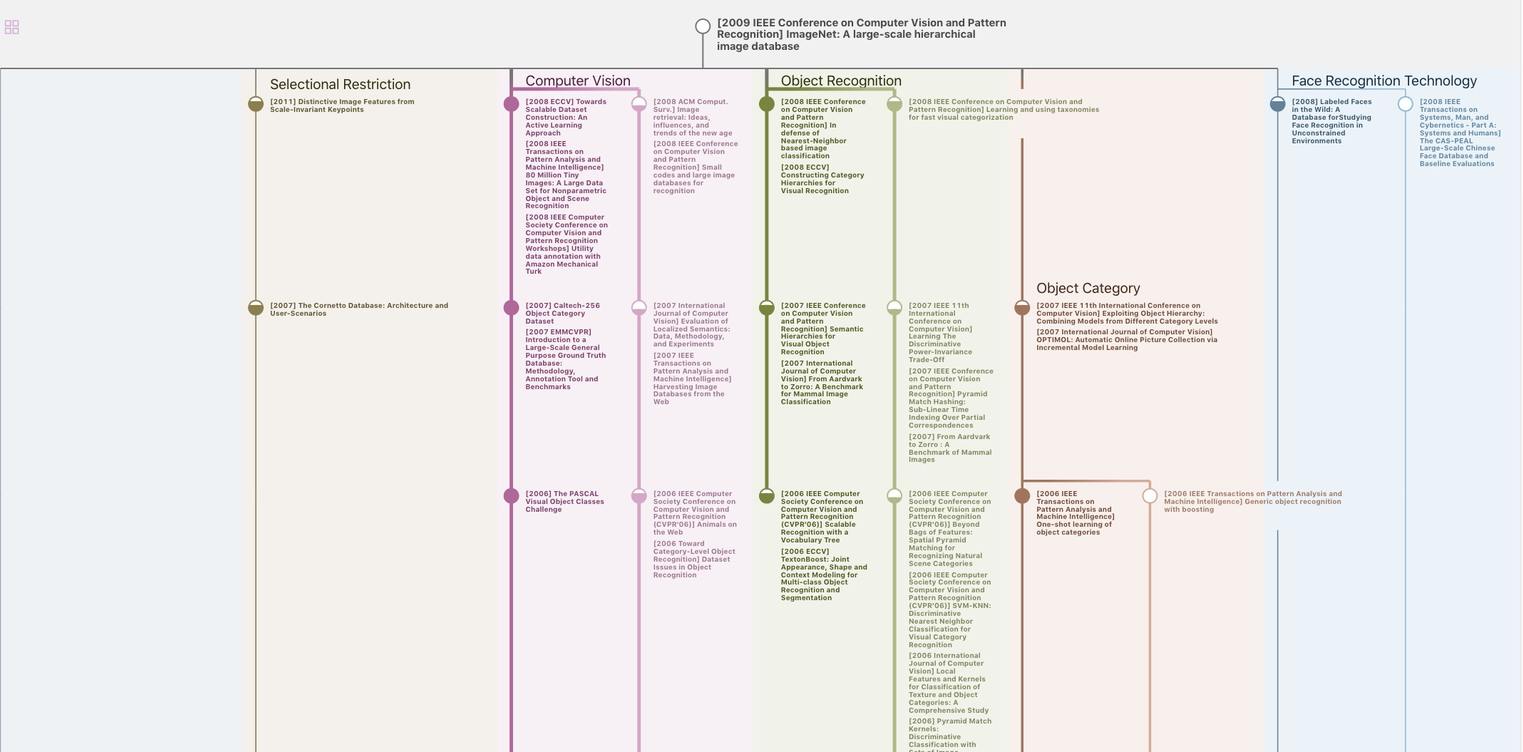
生成溯源树,研究论文发展脉络
Chat Paper
正在生成论文摘要