Coding Efficiency and Complexity Analysis of the Geometry-based Point Cloud Encoder
15TH IEEE LATIN AMERICAN SYMPOSIUM ON CIRCUITS AND SYSTEMS, LASCAS 2024(2024)
Univ Fed Pelotas
Abstract
In recent years, 3D point cloud content has gained attention thanks to applications such as virtual, augmented or mixed reality, real-time immersive communications, and autonomous driving systems. However, raw point clouds comprise large amount of data, and compression is mandatory to allow efficient transmission and storage. The MPEG group proposed the standard called Geometry-based Point Cloud Compression (G-PCC) implemented in the Test Condition Category 1 and 3 (TMC13) software. TMC13 can significantly reduce the amount of data in static point clouds, but requires a high computational cost making real-time compression unfeasible, especially for devices with limited computational power and energy resources. This paper presents a computational cost and coding efficiency evaluation of the G-PCC coding tools. Two main contributions are presented: first, a coding efficiency analysis of different G-PCC tools; second, a complexity profiling of the G-PCC coding steps aimed at understanding the computational effort distribution. This work provides the first complexity assessment of G-PCC. The presented results can help in the proposal of complexity reduction approaches for the development of more efficient versions of G-PCC for real-time purposes.
MoreTranslated text
Key words
G-PCC tools,coding efficiency analysis,complexity,computational cost
求助PDF
上传PDF
View via Publisher
AI Read Science
AI Summary
AI Summary is the key point extracted automatically understanding the full text of the paper, including the background, methods, results, conclusions, icons and other key content, so that you can get the outline of the paper at a glance.
Example
Background
Key content
Introduction
Methods
Results
Related work
Fund
Key content
- Pretraining has recently greatly promoted the development of natural language processing (NLP)
- We show that M6 outperforms the baselines in multimodal downstream tasks, and the large M6 with 10 parameters can reach a better performance
- We propose a method called M6 that is able to process information of multiple modalities and perform both single-modal and cross-modal understanding and generation
- The model is scaled to large model with 10 billion parameters with sophisticated deployment, and the 10 -parameter M6-large is the largest pretrained model in Chinese
- Experimental results show that our proposed M6 outperforms the baseline in a number of downstream tasks concerning both single modality and multiple modalities We will continue the pretraining of extremely large models by increasing data to explore the limit of its performance
Upload PDF to Generate Summary
Must-Reading Tree
Example
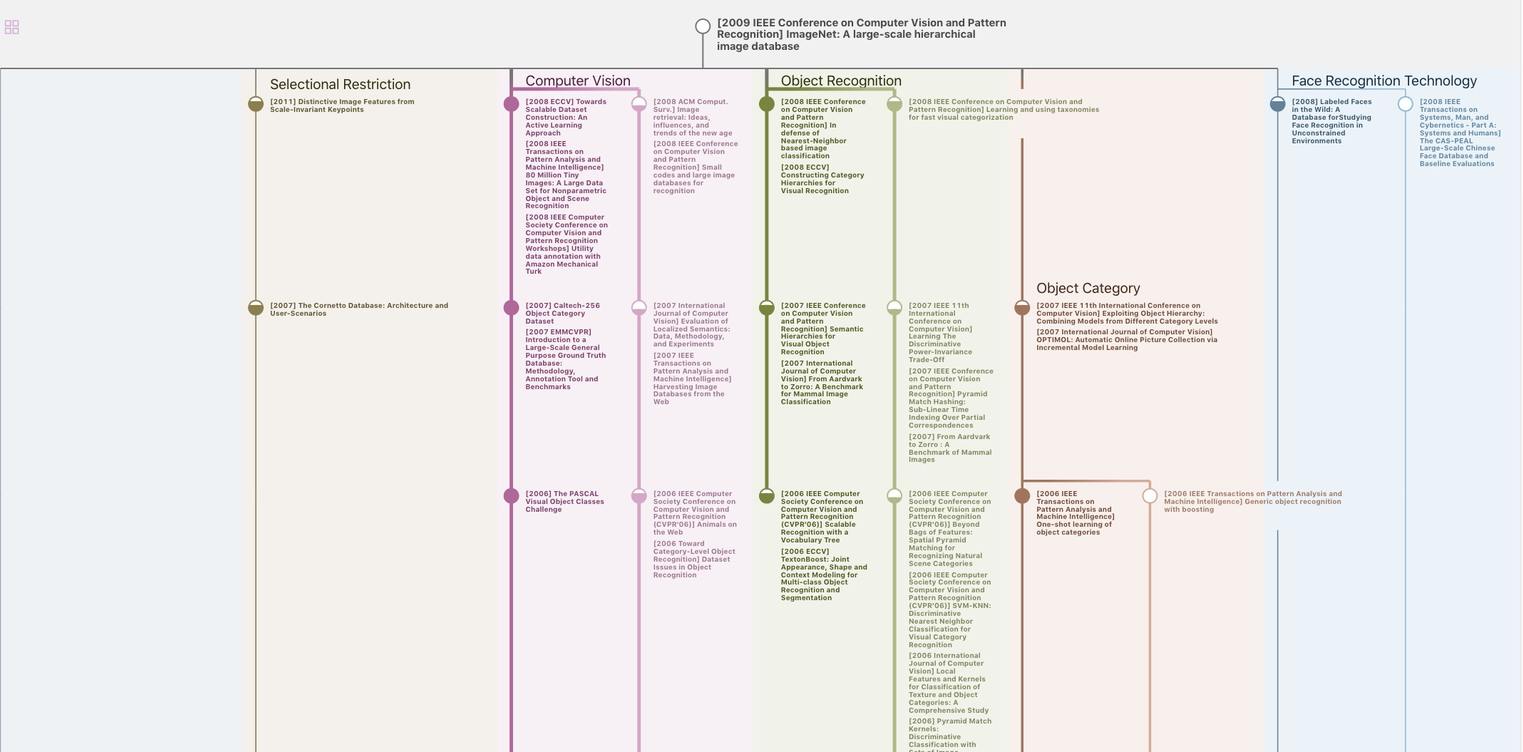
Generate MRT to find the research sequence of this paper
Related Papers
Data Disclaimer
The page data are from open Internet sources, cooperative publishers and automatic analysis results through AI technology. We do not make any commitments and guarantees for the validity, accuracy, correctness, reliability, completeness and timeliness of the page data. If you have any questions, please contact us by email: report@aminer.cn
Chat Paper