RE-Specter: Examining the Architectural Features of Configurable CNN With Power Side-Channel
IEEE Transactions on Computer-Aided Design of Integrated Circuits and Systems(2024)
摘要
As domain-specific training data is recognized as valuable intellectual property, acquiring well-trained weights in Convolutional Neural Networks (CNN) has emerged as a new threat to the neural network design community. To design a CNN accelerator that is resilient to side-channel threats, it is crucial to have an accurate and efficient security-driven framework at the early design stage. However, there is no standard way to perform root-cause analysis on the power side channel that exists in FPGA-based CNN accelerators. Therefore, we build RE-Specter, a framework that facilitates security-driven design space exploration (DSE) across various building components, combination patterns, and parallelism configurations in CNNs. The goal is to fully understand the power side-channel effects resulting from architectural modifications or optimization decisions. We further compare the benchmarks considering precision, resource utilization, and power side-channel leakage. Finally, we experimentally explore the design space of various architectural features. The experimental results show that low-bit precision delivers more secure architectures (68.9× among DSPs, 2439× among LUTs) in Measurement-To-Disclosure (MTD), but mixed-precision strategies are necessary to maintain the model accuracy. For loop optimization, in 16-parallel scenario, accumulator-based architecture outperforms the architecture featuring an adder tree with the improvements of 8.28× in MTD and 1.38× in PST.
更多查看译文
AI 理解论文
溯源树
样例
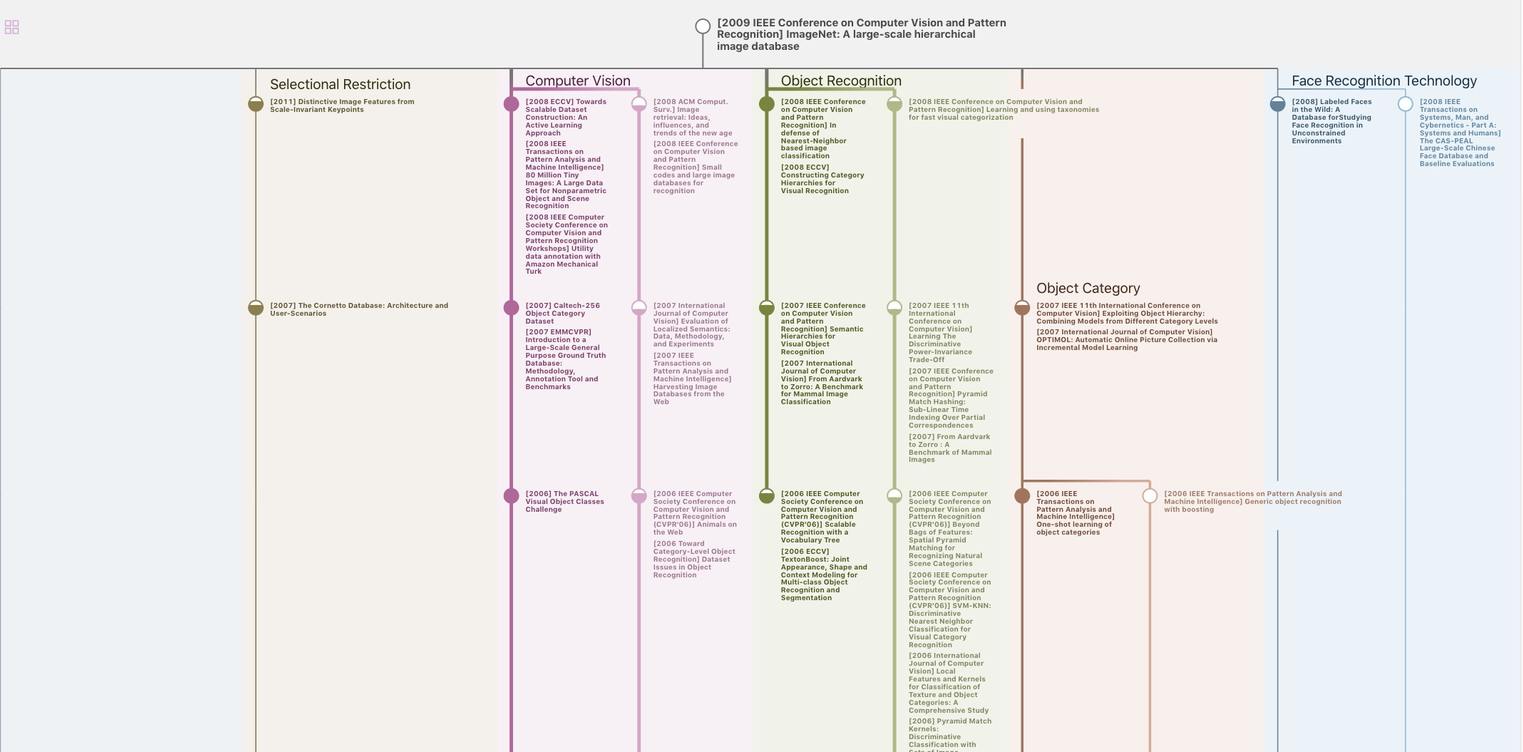
生成溯源树,研究论文发展脉络
Chat Paper
正在生成论文摘要