Prediction of Self-Similar Waves in Tapered Graded Index Diffraction Decreasing Waveguide by the A-gPINN Method
Nonlinear Dynamics(2024)
关键词
Tapered graded index waveguide,Generalized inhomogeneous nonlinear Schrödinger equation,Self-similar waves,Physics-informed neural network,Forward and inverse problems
AI 理解论文
溯源树
样例
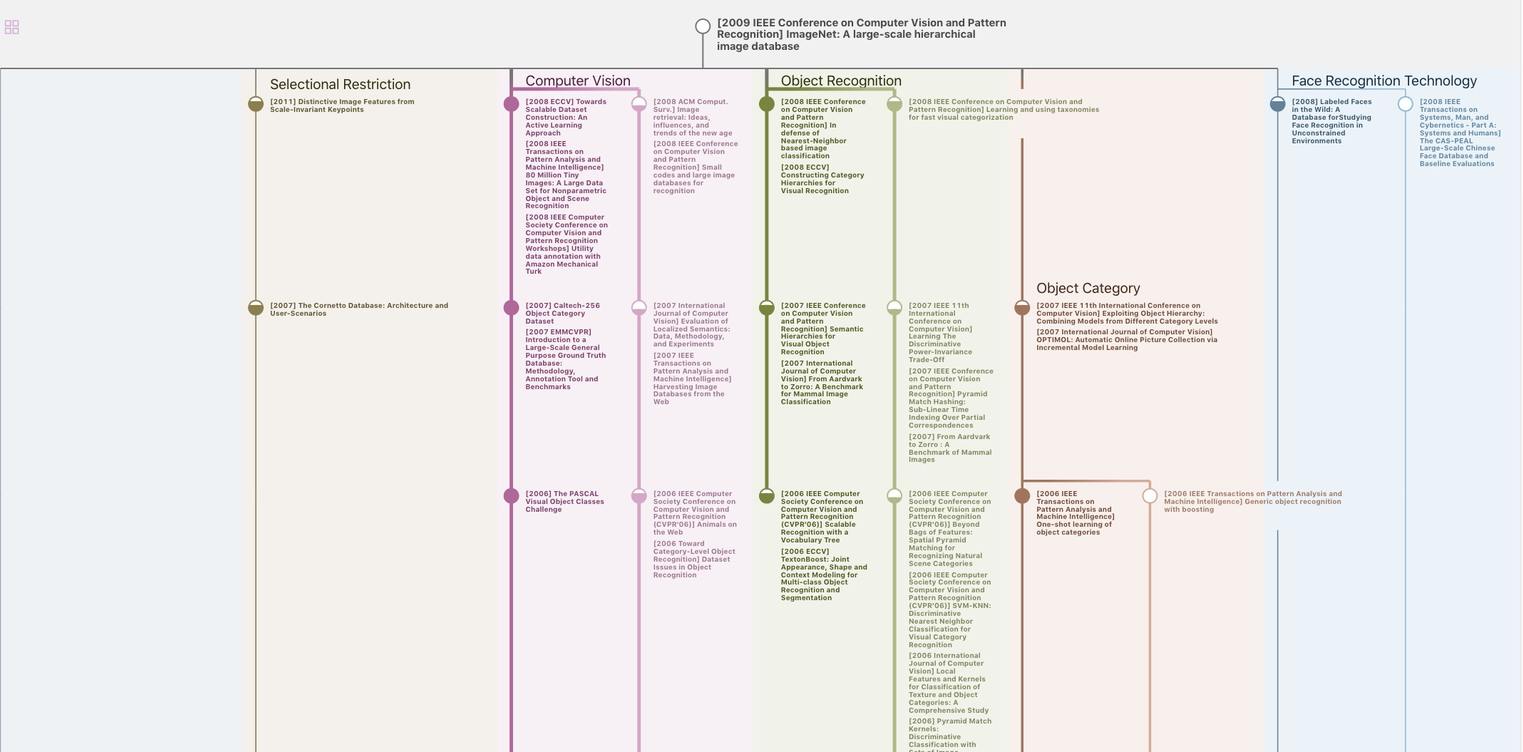
生成溯源树,研究论文发展脉络
Chat Paper
正在生成论文摘要