Development and external validation of a transfer learning-based system for the pathological diagnosis of colorectal cancer: a large emulated prospective study.
Frontiers in oncology(2024)
摘要
Background:The progress in Colorectal cancer (CRC) screening and management has resulted in an unprecedented caseload for histopathological diagnosis. While artificial intelligence (AI) presents a potential solution, the predominant emphasis on slide-level aggregation performance without thorough verification of cancer in each location, impedes both explainability and transparency. Effectively addressing these challenges is crucial to ensuring the reliability and efficacy of AI in histology applications.
Method:In this study, we created an innovative AI algorithm using transfer learning from a polyp segmentation model in endoscopy. The algorithm precisely localized CRC targets within 0.25 mm² grids from whole slide imaging (WSI). We assessed the CRC detection capabilities at this fine granularity and examined the influence of AI on the diagnostic behavior of pathologists. The evaluation utilized an extensive dataset comprising 858 consecutive patient cases with 1418 WSIs obtained from an external center.
Results:Our results underscore a notable sensitivity of 90.25% and specificity of 96.60% at the grid level, accompanied by a commendable area under the curve (AUC) of 0.962. This translates to an impressive 99.39% sensitivity at the slide level, coupled with a negative likelihood ratio of <0.01, signifying the dependability of the AI system to preclude diagnostic considerations. The positive likelihood ratio of 26.54, surpassing 10 at the grid level, underscores the imperative for meticulous scrutiny of any AI-generated highlights. Consequently, all four participating pathologists demonstrated statistically significant diagnostic improvements with AI assistance.
Conclusion:Our transfer learning approach has successfully yielded an algorithm that can be validated for CRC histological localizations in whole slide imaging. The outcome advocates for the integration of the AI system into histopathological diagnosis, serving either as a diagnostic exclusion application or a computer-aided detection (CADe) tool. This integration has the potential to alleviate the workload of pathologists and ultimately benefit patients.
更多查看译文
关键词
CRC (colorectal cancer),pathological diagnosis,AI-assisted pathological diagnosis,transfer learning,artificial intelligence (AI)
AI 理解论文
溯源树
样例
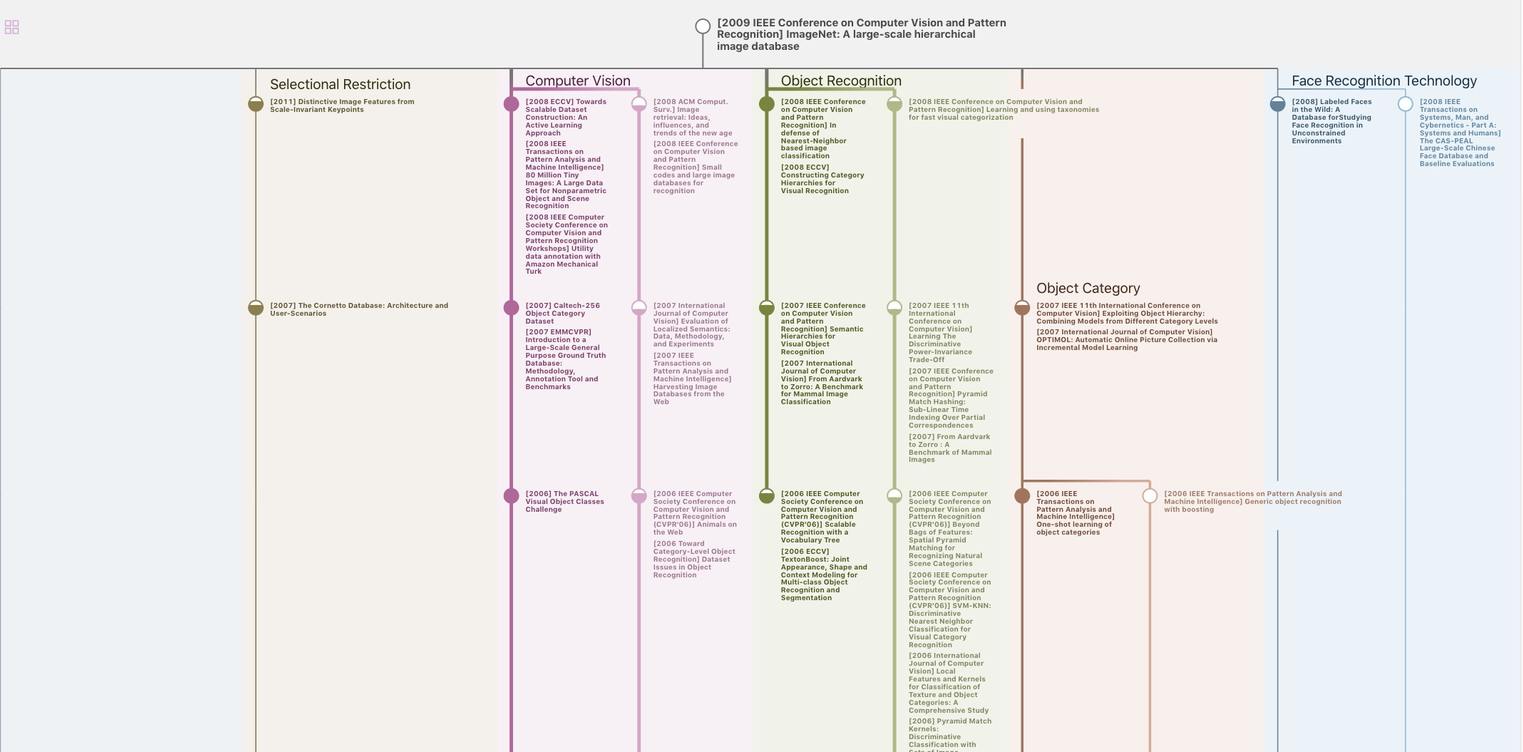
生成溯源树,研究论文发展脉络
Chat Paper
正在生成论文摘要