Causal Learning for Robust Specific Emitter Identification Over Unknown Channel Statistics.
IEEE Trans. Inf. Forensics Secur.(2024)
摘要
Specific emitter identification (SEI) is a device identification technology that extracts radio frequency (RF) fingerprint from received signals. However, channel effects on RF fingerprint can vary between the training and testing stage, and SEI based on deep learning (DL) will be unable to withstand channel changes. To address this problem, we propose a channel-robust SEI scheme driven by causal learning. We analyze received signals from the causal perspective and construct a structural causal model (SCM) of SEI. In the SCM, received signals are considered as mixtures of the causal element and interference element, and only the former affects identification. Additionally, we design a new RF fingerprint feature representation called the centralized logarithmic power spectrum (CLPS) to reduce the impact of channel effects. Furthermore, we propose a causal purification network (CPNet) driven by causality to further alleviate channel effects. CPNet weakens the spurious associations between the channel and emitter labels through feature decorrelation and feature purification, strengthens the correlation between RF fingerprint and labels, and improves the generalization of SEI. Finally, our approach is evaluated extensively using 20 ZigBee devices under different channel environments. Experimental results demonstrate that our scheme can effectively alleviate channel effects, improve SEI performance under various channel environments, and exhibit good generalization and stability.
更多查看译文
关键词
Specific emitter identification,radio frequency fingerprint,causal learning,channel,deep learning
AI 理解论文
溯源树
样例
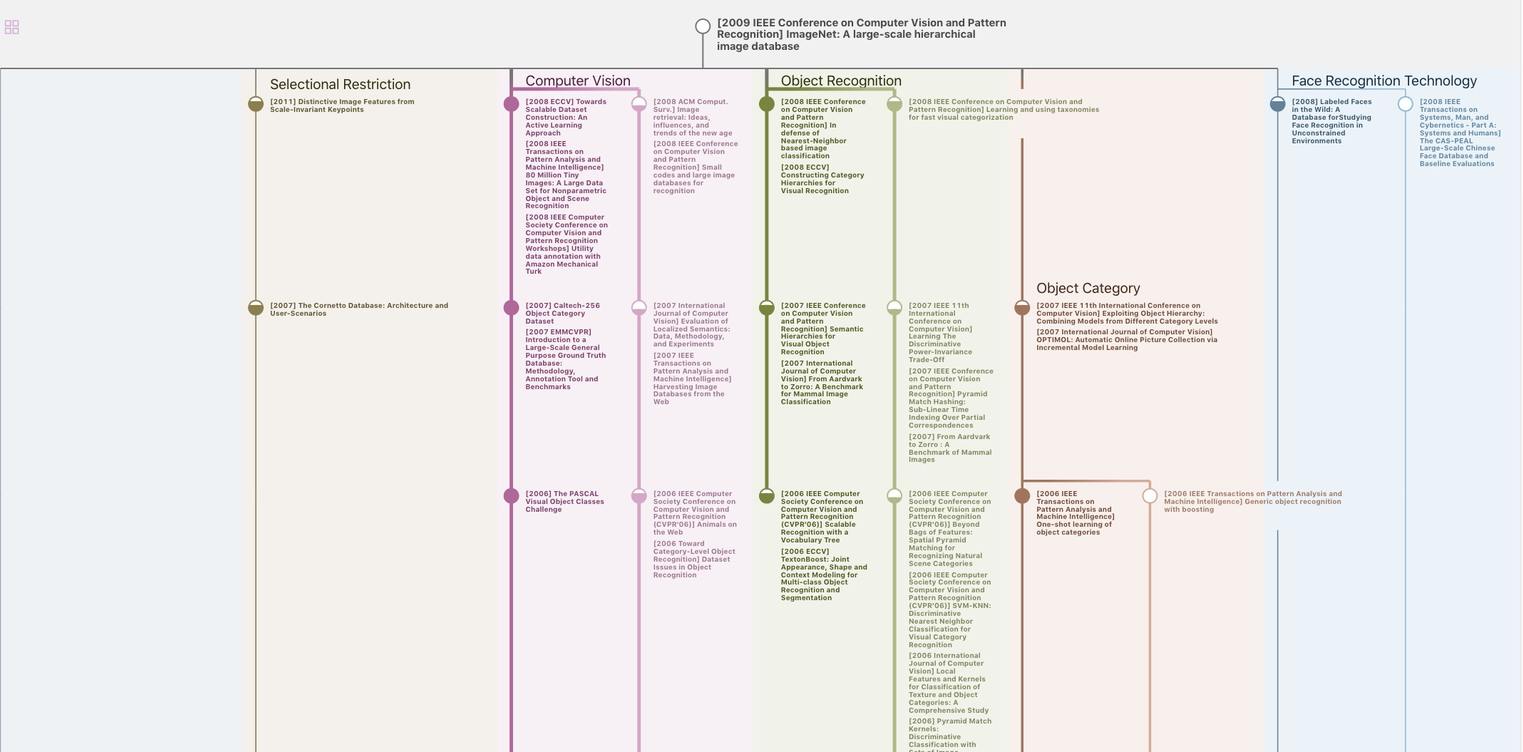
生成溯源树,研究论文发展脉络
Chat Paper
正在生成论文摘要