Multi-Sample Dynamic Time Warping for Few-Shot Keyword Spotting
CoRR(2024)
摘要
In multi-sample keyword spotting, each keyword class is represented by multiple spoken instances, called samples. A na\"ive approach to detect keywords in a target sequence consists of querying all samples of all classes using sub-sequence dynamic time warping. However, the resulting processing time increases linearly with respect to the number of samples belonging to each class. Alternatively, only a single Fr\'echet mean can be queried for each class, resulting in reduced processing time but usually also in worse detection performance as the variability of the query samples is not captured sufficiently well. In this work, multi-sample dynamic time warping is proposed to compute class-specific cost-tensors that include the variability of all query samples. To significantly reduce the computational complexity during inference, these cost tensors are converted to cost matrices before applying dynamic time warping. In experimental evaluations for few-shot keyword spotting, it is shown that this method yields a very similar performance as using all individual query samples as templates while having a runtime that is only slightly slower than when using Fr\'echet means.
更多查看译文
AI 理解论文
溯源树
样例
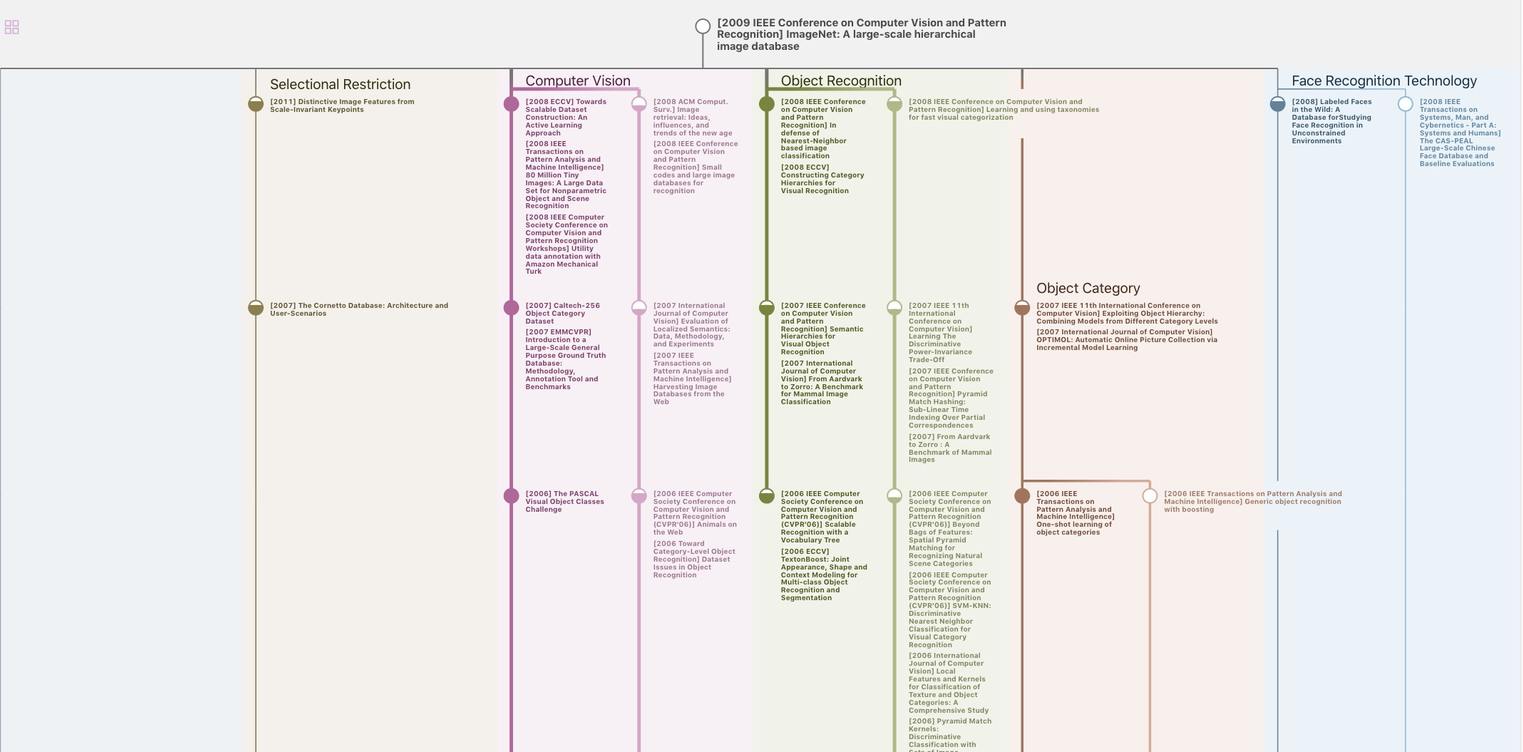
生成溯源树,研究论文发展脉络
Chat Paper
正在生成论文摘要