Study on Defect Detection of Metal Castings Based on Supervised Enhancement and Attention Distillation
Machine Vision and Applications(2024)
摘要
Metal castings are essential parts and their quality greatly impacts the product’s performance. Current techniques for identifying surface defects in metal castings usually suffer from issues like inadequate accuracy in defect recognition. In comparison to other models, the performance of the Deformable DETR model in detecting defects in metal castings is commendable, yet its attentional mechanism inherently demands a larger sample size and exhibits a slower convergence rate. However, the dataset collected in this paper for metal castings possesses a limited number of samples and imbalanced data, which consequently limits the overall effectiveness of the Deformable DETR model on this task. Responding to the above, a supervised enhancement algorithm for one-to-many assignment based on the Deformable DETR was proposed in this paper. This algorithm accelerates convergence speed and reduces data requirements while eliminating Non-Maximum Suppression post-processing. Applying the supervised enhancement algorithm to DETR on this dataset also improves defect recall. In addition, attention distillation was applied to the deformable attention mechanism to reduce time and space complexity to O(Llog(L)). It creates favourable conditions for the generation of attention weights, which are changed from a linear transformation of query to query-key interaction, and emphasises casting defects while ensuring global attention. Furthermore, data processing methods such as image slicing and data augmentation are also used to enhance casting defect detection ability in this paper. Finally, the recall rate and precision rate of metal casting defects have been improved, increasing to 97.7
更多查看译文
关键词
Defect detection,Attention mechanism,Supervised enhancement,Attention distillation,Data augmentation
AI 理解论文
溯源树
样例
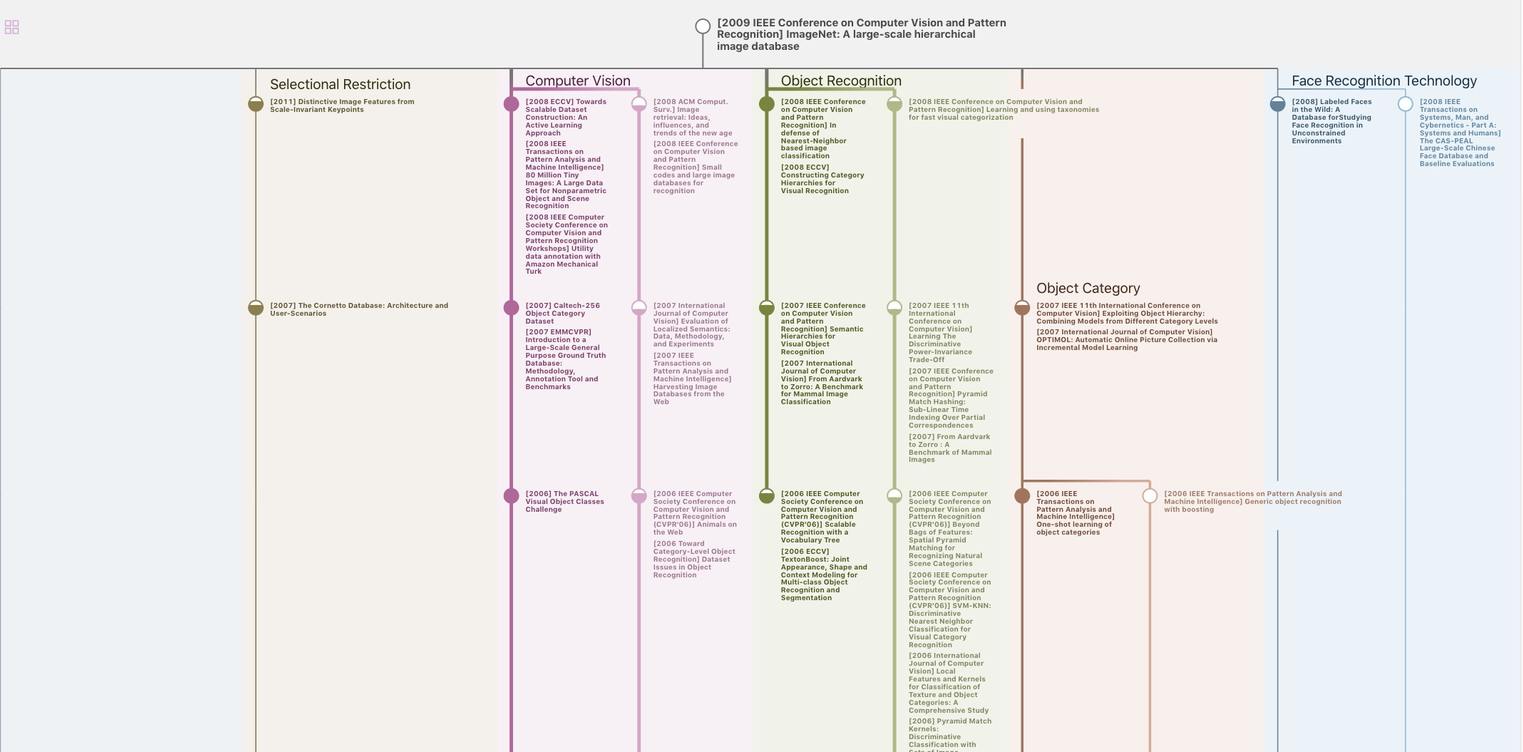
生成溯源树,研究论文发展脉络
Chat Paper
正在生成论文摘要