The Characteristics of Fungal Responses to Uranium Mining Activities and Analysis of Their Tolerance to Uranium
Ecotoxicology and Environmental Safety(2024)SCI 2区SCI 1区
Key Laboratory of Coarse Cereal Processing | School of Public Health | Sichuan Environm Monitoring Ctr | Chengdu Med Coll
Abstract
The influence of uranium (U) mining on the fungal diversity (FD) and communities (FC) structure was investigated in this work. Our results revealed that soil FC richness and FD indicators obviously decreased due to U, such as Chao1, observed OTUs and Shannon index (P<0.05). Moreover, the abundances of Mortierella, Gibberella, and Tetracladium were notably reduced in soil samples owing to U mining activities (P<0.05). In contrast, the abundances of Cadophora, Pseudogymnoascus, Mucor, and Sporormiella increased in all soil samples after U mining (P<0.05). Furthermore, U mining not only dramatically influenced the Plant_Pathogen guild and Saprotroph and Pathotroph modes (P<0.05), but also induced the differentiation of soil FC and the enrichment of the Animal_Pathogen-Soil_Saprotroph and Endophyte guilds and Symbiotroph and Pathotroph Saprotroph trophic modes. In addition, various fungal populations and guilds were enriched to deal with the external stresses caused by U mining in different U mining areas and soil depths (P<0.05). Finally, nine U-tolerant fungi were isolated and identified with a minimum inhibitory concentration range of 400–600 mg/L, and their adsorption efficiency for U ranged from 11.6% to 37.9%. This study provides insights into the impact of U mining on soil fungal stability and the response of fungi to U mining activities, as well as aids in the screening of fungal strains that can be used to promote remediation of U mining sites on plateaus.
MoreTranslated text
Key words
Uranium,Environmental pollution,Bioremediation,Fungal diversity,Pollution control
求助PDF
上传PDF
View via Publisher
AI Read Science
AI Summary
AI Summary is the key point extracted automatically understanding the full text of the paper, including the background, methods, results, conclusions, icons and other key content, so that you can get the outline of the paper at a glance.
Example
Background
Key content
Introduction
Methods
Results
Related work
Fund
Key content
- Pretraining has recently greatly promoted the development of natural language processing (NLP)
- We show that M6 outperforms the baselines in multimodal downstream tasks, and the large M6 with 10 parameters can reach a better performance
- We propose a method called M6 that is able to process information of multiple modalities and perform both single-modal and cross-modal understanding and generation
- The model is scaled to large model with 10 billion parameters with sophisticated deployment, and the 10 -parameter M6-large is the largest pretrained model in Chinese
- Experimental results show that our proposed M6 outperforms the baseline in a number of downstream tasks concerning both single modality and multiple modalities We will continue the pretraining of extremely large models by increasing data to explore the limit of its performance
Upload PDF to Generate Summary
Must-Reading Tree
Example
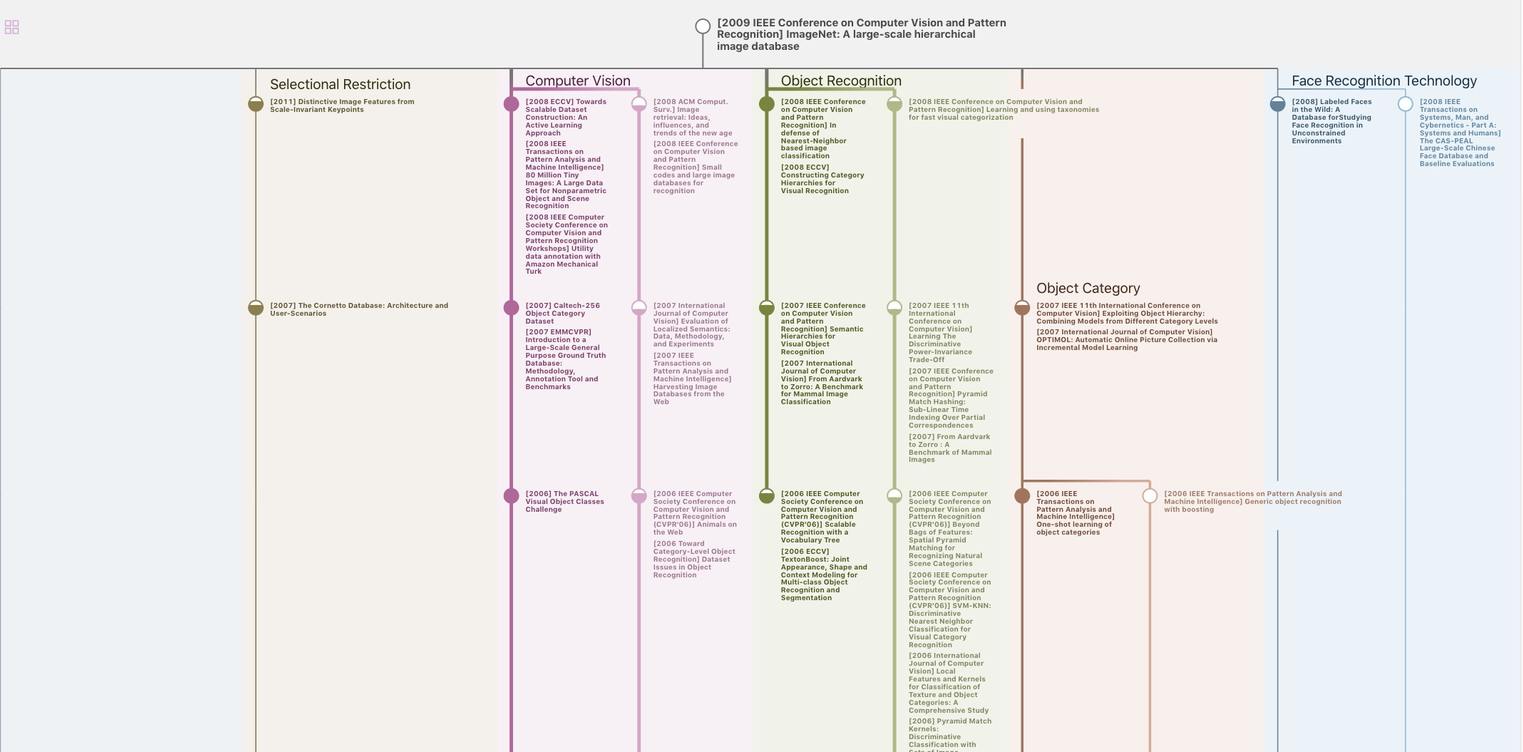
Generate MRT to find the research sequence of this paper
Related Papers
Data Disclaimer
The page data are from open Internet sources, cooperative publishers and automatic analysis results through AI technology. We do not make any commitments and guarantees for the validity, accuracy, correctness, reliability, completeness and timeliness of the page data. If you have any questions, please contact us by email: report@aminer.cn
Chat Paper