Composing Pre-Trained Object-Centric Representations for Robotics from "what" and "where" Foundation Models
arXiv (Cornell University)(2024)
摘要
There have recently been large advances both in pre-training visualrepresentations for robotic control and segmenting unknown category objects ingeneral images. To leverage these for improved robot learning, we proposePOCR, a new framework for building pre-trained object-centricrepresentations for robotic control. Building on theories of "what-where"representations in psychology and computer vision, we use segmentations from apre-trained model to stably locate across timesteps, various entities in thescene, capturing "where" information. To each such segmented entity, we applyother pre-trained models that build vector descriptions suitable for roboticcontrol tasks, thus capturing "what" the entity is. Thus, our pre-trainedobject-centric representations for control are constructed by appropriatelycombining the outputs of off-the-shelf pre-trained models, with no newtraining. On various simulated and real robotic tasks, we show that imitationpolicies for robotic manipulators trained on POCR achieve better performanceand systematic generalization than state of the art pre-trained representationsfor robotics, as well as prior object-centric representations that aretypically trained from scratch.
更多查看译文
关键词
Representation Learning,Imitation Learning,Sensorimotor Learning
AI 理解论文
溯源树
样例
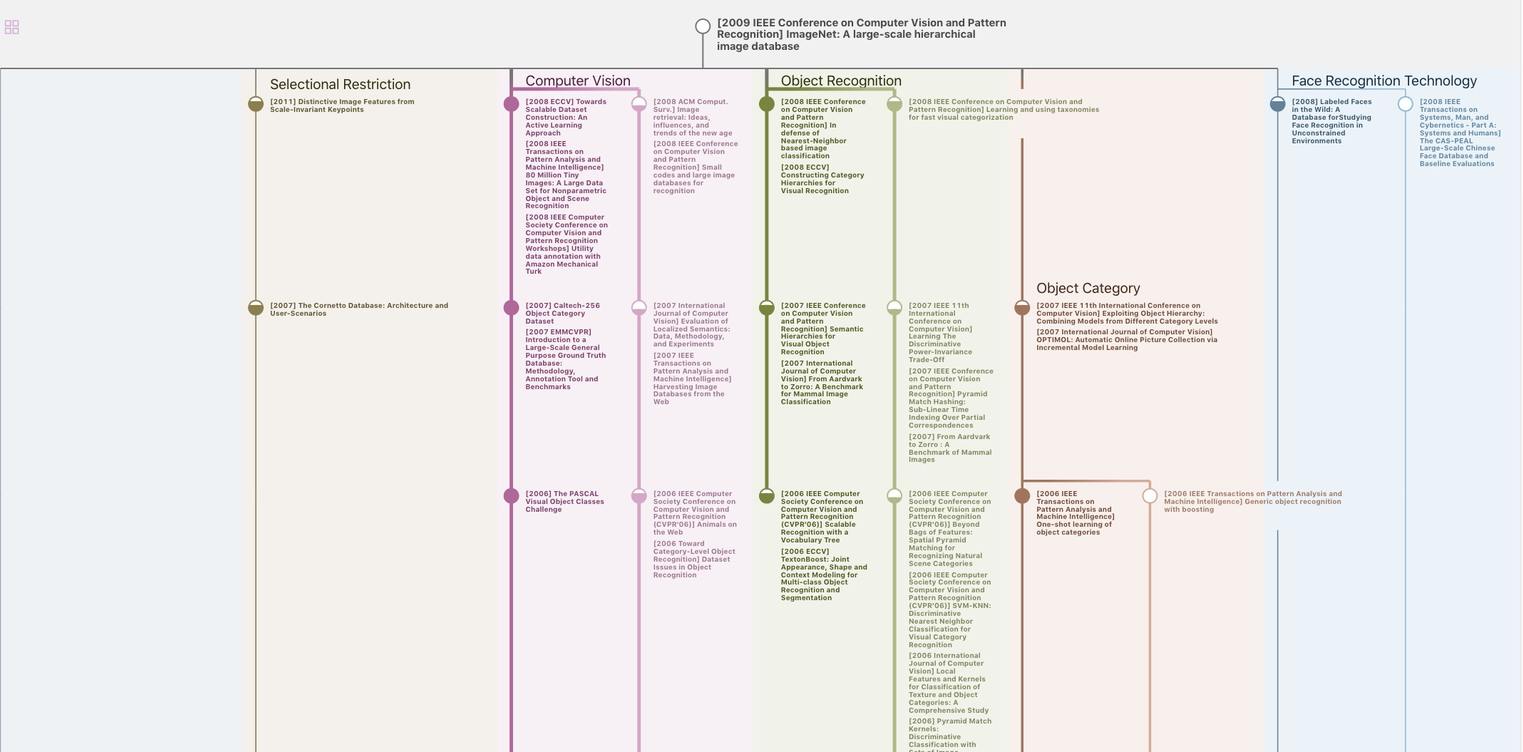
生成溯源树,研究论文发展脉络
Chat Paper
正在生成论文摘要