Collaborative Visual Place Recognition Through Federated Learning
2024 IEEE/CVF Conference on Computer Vision and Pattern Recognition Workshops (CVPRW)(2024)
摘要
Visual Place Recognition (VPR) aims to estimate the location of an image bytreating it as a retrieval problem. VPR uses a database of geo-tagged imagesand leverages deep neural networks to extract a global representation, calleddescriptor, from each image. While the training data for VPR models oftenoriginates from diverse, geographically scattered sources (geo-tagged images),the training process itself is typically assumed to be centralized. Thisresearch revisits the task of VPR through the lens of Federated Learning (FL),addressing several key challenges associated with this adaptation. VPR datainherently lacks well-defined classes, and models are typically trained usingcontrastive learning, which necessitates a data mining step on a centralizeddatabase. Additionally, client devices in federated systems can be highlyheterogeneous in terms of their processing capabilities. The proposed FedVPRframework not only presents a novel approach for VPR but also introduces a new,challenging, and realistic task for FL research, paving the way to other imageretrieval tasks in FL.
更多查看译文
关键词
Visual Recognition,Federated Learning,Place Recognition,Visual Place Recognition,Deep Neural Network,Image Retrieval,Self-supervised Learning,Image Retrieval Task,Validation Set,Global Model,Number Of Images,Stochastic Gradient Descent,Latent Space,Autonomous Vehicles,Statistical Heterogeneity,City Level,Local Dataset,Central Server,Edge Devices,Simultaneous Localization And Mapping,Triplet Loss,Server Side,Local Iterations,Aggregation Layer,Communication Rounds,Query Image,Training Round,Color Jittering,Geographical Proximity,Data Augmentation
AI 理解论文
溯源树
样例
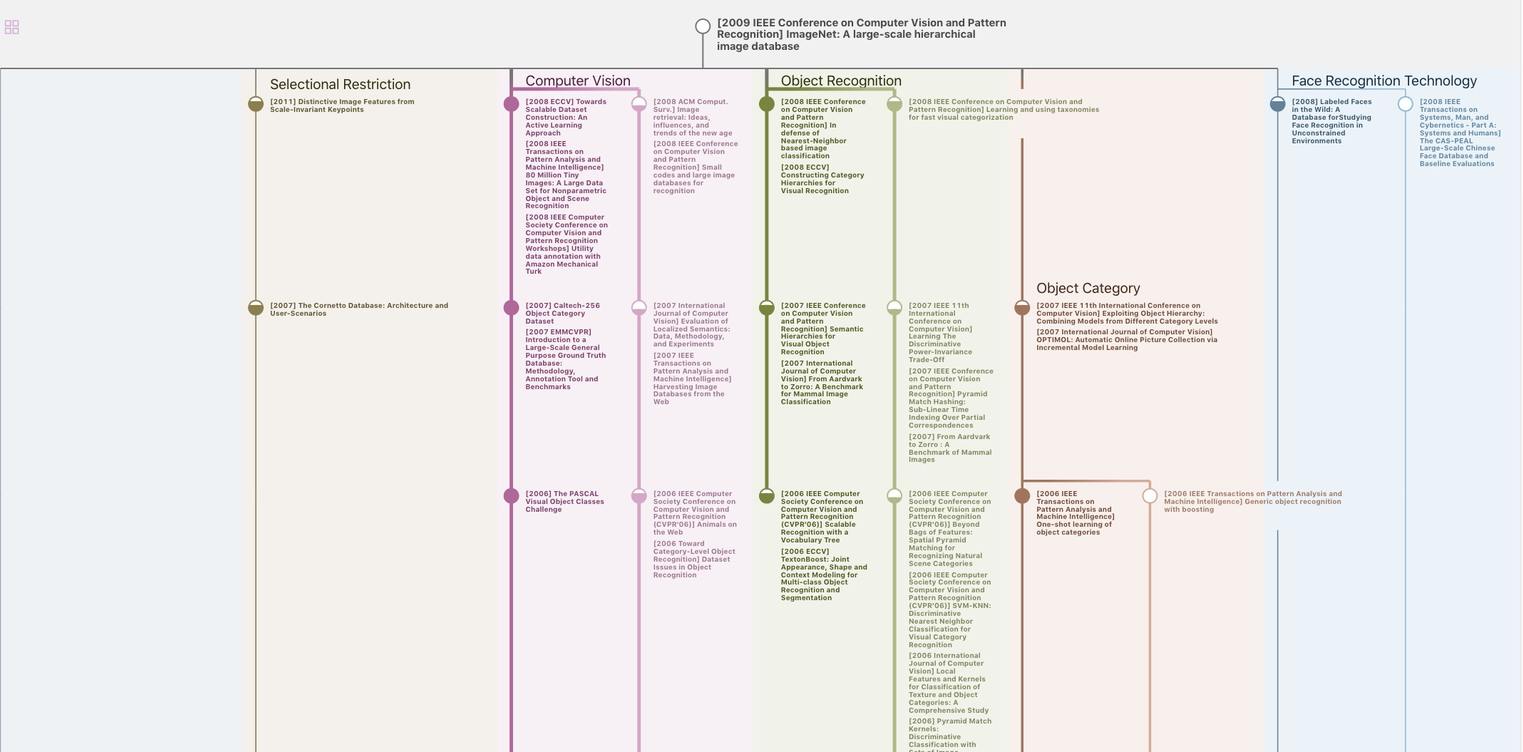
生成溯源树,研究论文发展脉络
Chat Paper
正在生成论文摘要