Stronger Random Baselines for In-Context Learning
arxiv(2024)
摘要
Evaluating the in-context learning classification performance of language
models poses challenges due to small dataset sizes, extensive prompt-selection
using the validation set, and intentionally difficult tasks that lead to
near-random performance. The standard random baseline – the expected accuracy
of guessing labels uniformly at random – is stable when the evaluation set is
used only once or when the dataset is large. We account for the common practice
of validation set reuse and existing small datasets with a stronger random
baseline: the expected maximum accuracy across multiple random classifiers.
When choosing the best prompt demonstrations across six quantized language
models applied to 16 BIG-bench Lite tasks, more than 20% of the few-shot
results that exceed the standard baseline do not exceed this stronger random
baseline. When held-out test sets are available, this stronger baseline is also
a better predictor of held-out performance than the standard baseline, avoiding
unnecessary test set evaluations. This maximum random baseline provides an
easily calculated drop-in replacement for the standard baseline.
更多查看译文
AI 理解论文
溯源树
样例
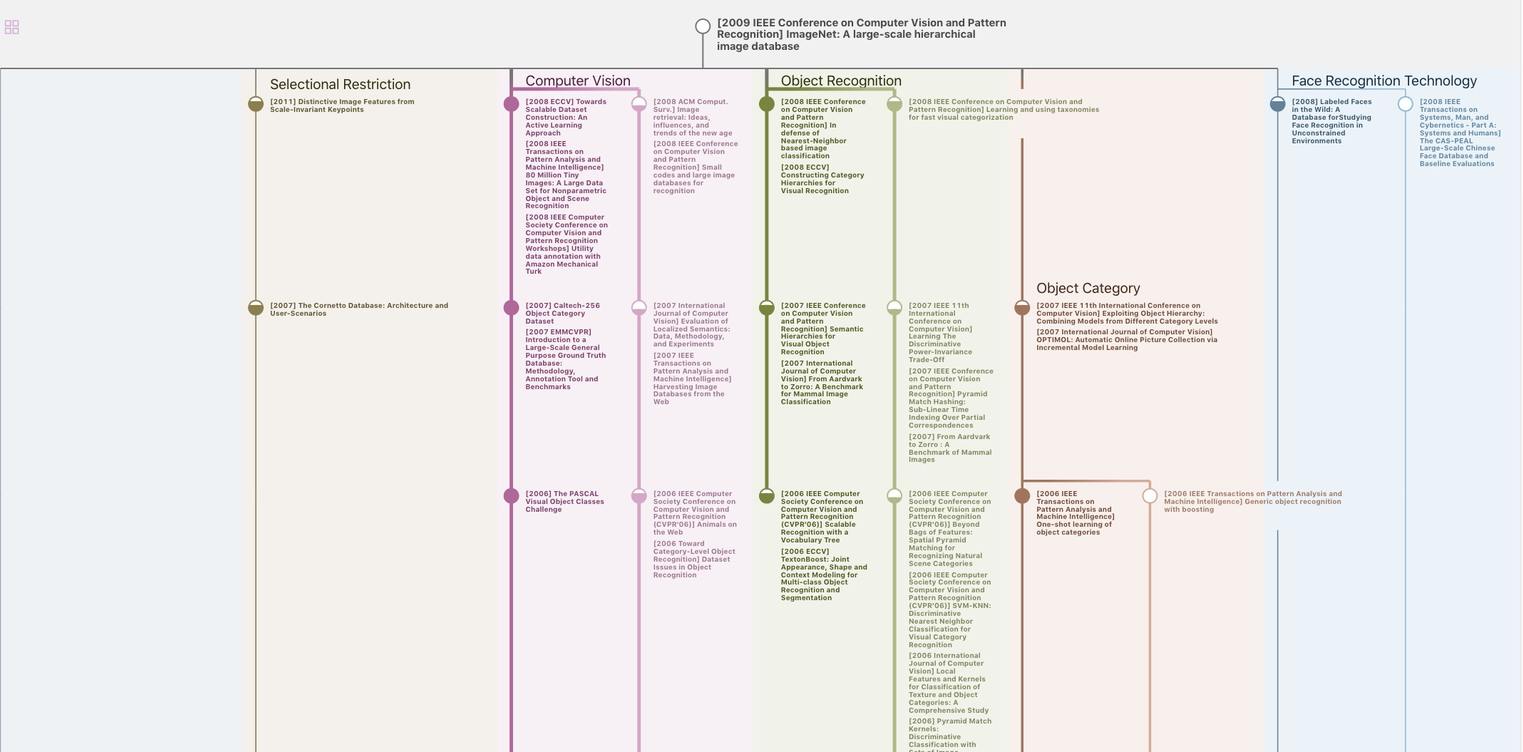
生成溯源树,研究论文发展脉络
Chat Paper
正在生成论文摘要