Stochastic Inversion of Fracture Networks Using the Reversible Jump Markov Chain Monte Carlo Algorithm
Energy(2024)
摘要
Characterization of fracture networks is essential in the production optimization or storage calculation for enhanced geothermal systems or geologic carbon storage. A novel inversion approach is proposed for estimating the fracture networks in this research. The discrete fracture network technique is adopted to probabilistically describe various fracture parameters such as trace length, midpoint position or azimuthal angle. The reversible jump Markov chain Monte Carlo algorithm is applied to explore the target posterior distribution of model parameters of differing dimensionality, in which the number of fractures is assumed to be unknown. More specifically, the birth-death strategy is utilized to perturb the fracture number iteratively in the sampling process. The proposed methodology is applied with two different types of observational datasets, namely the head records from steady-state flow simulation and the acoustic impedance obtained from seismic inversion. The sampling results can successfully recover the fracture geometry in the observed domain, and the number of fractures in the system can be retrieved as well. Benchmarked on multiple Markov chain trials, the technique of parallel tempering can greatly improve the convergence efficiency and increase the diversity of sampled posterior models, through the random swapping of model states across the whole temperature ladder.
更多查看译文
关键词
Fracture geometry,Stochastic inversion,rjMCMC,Parallel tempering,Probability distribution
AI 理解论文
溯源树
样例
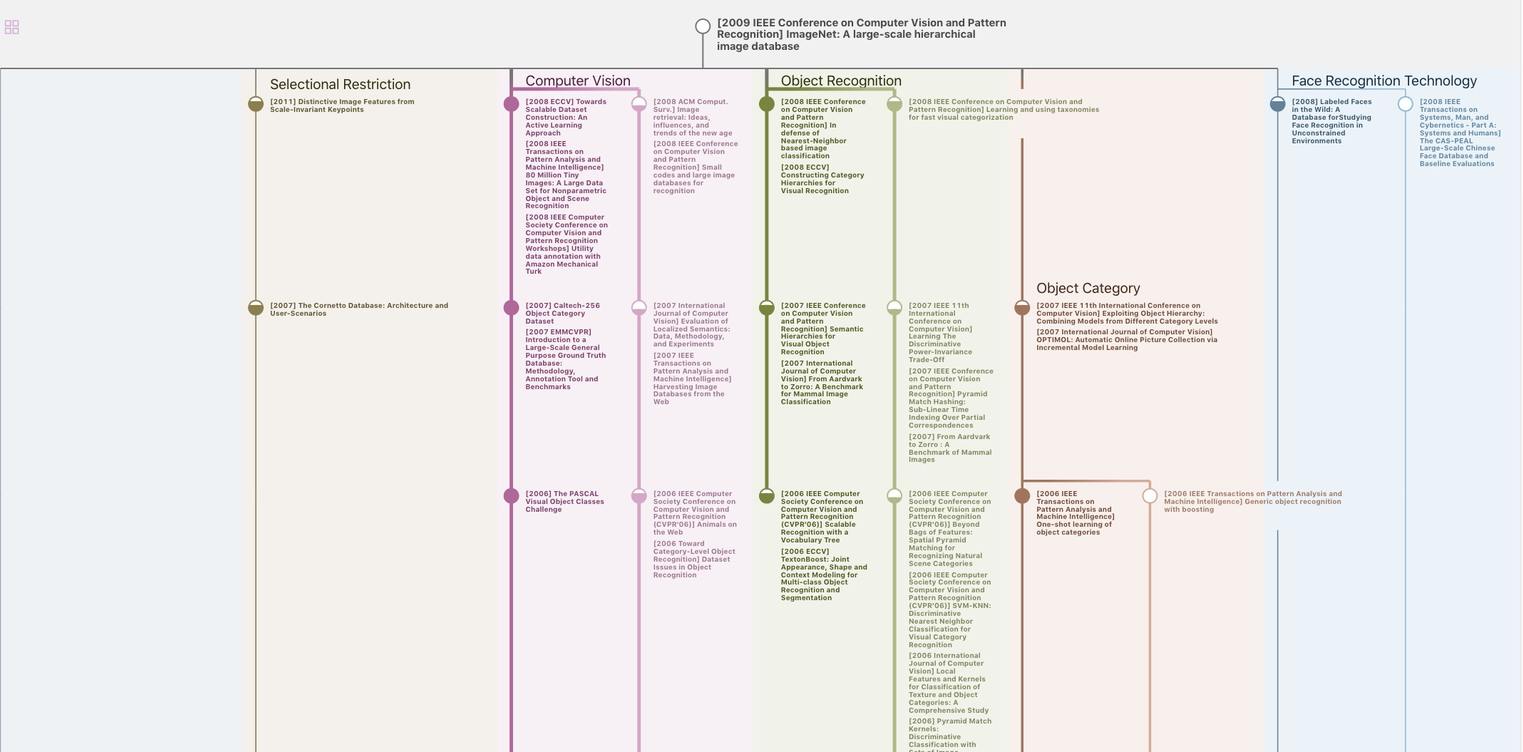
生成溯源树,研究论文发展脉络
Chat Paper
正在生成论文摘要