Collaborative Learning Based Spectrum Sensing under Partial Observations
IEEE Transactions on Cognitive Communications and Networking(2024)
摘要
To deal with the complex wireless cognitive radios, data-driven learning technologies have been advocated for spectrum sensing. While the existing learning-based methods are designed for basic single-band circumstances, they may not work well in practical wideband regimes. Due to the limited sensing capability and hardware constraints of practical secondary users (SUs) devices, individual SUs can only collect limited training data to observe a narrowband part of the entire wideband spectrum pool. It is known as the issue of partial observations, which leads to a heterogeneous multi-task learning problem. To overcome these challenges, this work proposes a novel framework of cooperative spectrum sensing via collaborative learning among distributed SUs. Capitalizing on the hierarchical nature of neurons of deep neural networks (DNN) in heterogeneous feature extraction, we propose a novel multi-task DNN architecture to detect wideband spectrum occupancy accurately and efficiently. By decoupling the large multi-band DNN into smaller band-specific sub-networks, these sub-networks can be jointly trained among distributed SUs even with heterogeneous local data. Simulation results indicate that our proposed method outperforms existing benchmarks in small-data regimes by achieving higher learning accuracy with less model complexity and computational cost.
更多查看译文
关键词
Cognitive radio,spectrum sensing,collaborative learning,partial observation,deep neural network,decoupling
AI 理解论文
溯源树
样例
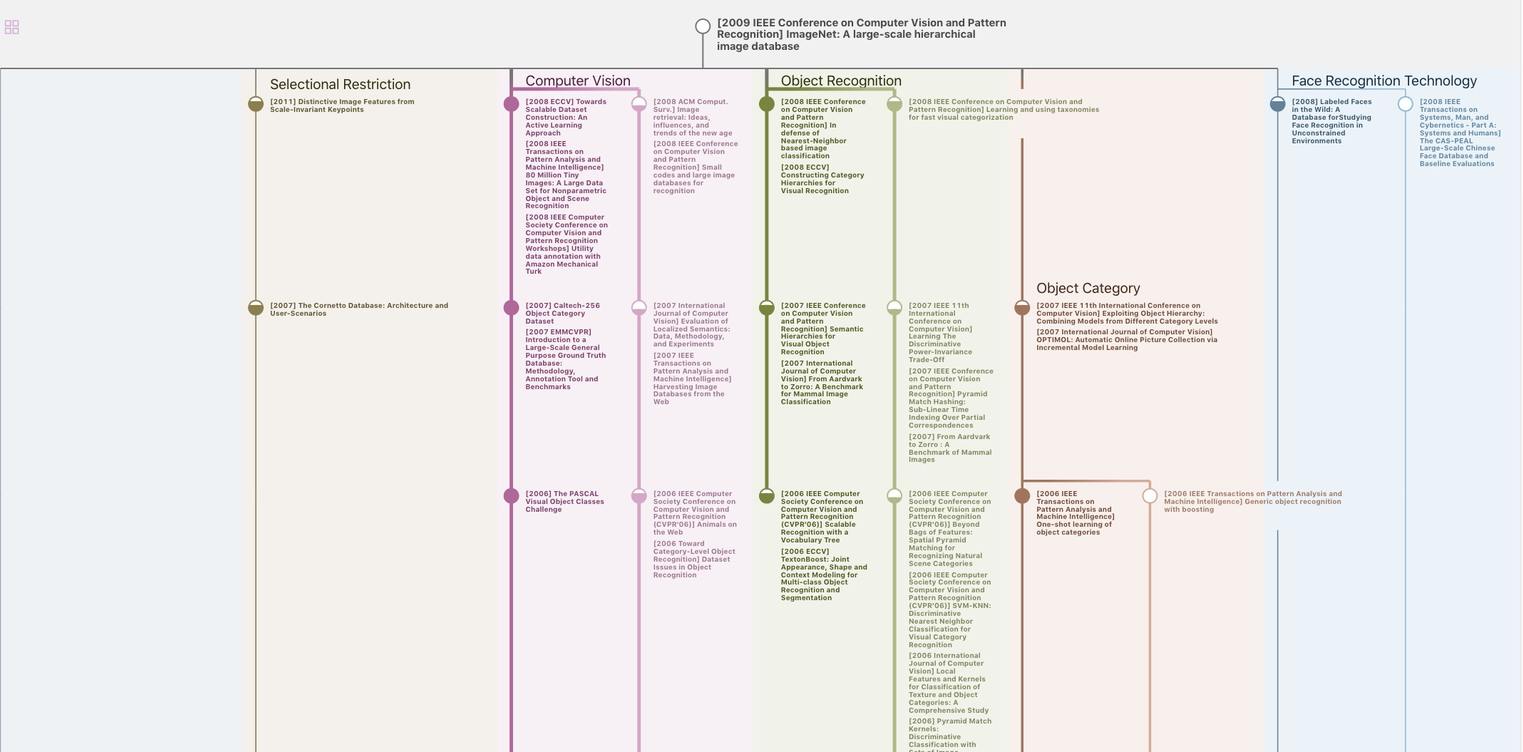
生成溯源树,研究论文发展脉络
Chat Paper
正在生成论文摘要